Transportation and logistics (T&L) companies have embraced advanced analytics in their operations, allowing them to run sophisticated global networks. Unfortunately, those investments in analytics haven’t been matched on the commercial side of the business. It’s time for that to change if companies in the T&L sector want to drive sales performance and growth.
Stay current on your favorite topics
Overall, the sector’s commercial analytics capabilities lag the average performance of B2B companies, which suggests that there is considerable potential (see exhibit). That’s primarily because distributed sales forces, common in T&L companies, often rely on outdated processes and don’t have accurate insight into customer preferences and growth opportunities. Catching up shouldn’t be daunting. T&L companies already have much of the data they need and can turn to analytics programs that are proven to work. Based on our experience, companies in the sector that embrace analytics can generate an additional 3 to 5 percent return on sales.
Such commercial success is not simply a nice-to-have. T&L companies are vulnerable because their increasingly sophisticated customer base has access to more products and services than ever and is adept at evaluating prices. In the trucking industry, for example, new entrants such as Uber Freight and Convoy have given customers transparency into the baseline cost for any journey.
Other B2B industries are already marching down the analytics-enabled path to commercial growth. T&L companies that have turned to analytics to drive growth have successfully addressed customer churn, improved customer leads by understanding which have the most potential, increased cross-selling and upselling, boosted sales-rep productivity, and tailored pricing.
But to deliver this level of commercial excellence, sales leaders must overcome some significant organizational barriers—not least the commercial function’s lack of access to operational data, which is so crucial to optimizing the commercial engine.
In our experience, T&L companies need to do three things to realize the potential of advanced analytics.
1. Wrangle the data you already have
The first step is to recognize what useful data already exists in the organization. It just requires some creative thinking to get it. For example, a distributor realized that although its customer-relationship management (CRM) system tracked customer volumes, it didn’t track them by location. That information did exist but was buried in the company warehouses’ order-management system, which tracked order dates, volumes, and delivery destinations. By analyzing the warehouse data set instead of the CRM, the company could learn how customer buying patterns shifted when new SKUs were introduced, when prices were increased, or when delivery frequencies changed. The newfound insights allowed them to create a predictive churn model that allowed proactive intervention and reduced churn by 15 percent (2 percent of revenue).
Accessing operational data from within your own company is surprisingly hard. The commercial team needs to find way to learn about what operational data exists and how it is being used by talking to operational leaders about what data they use, asking data analysts who work on operational topics, or taking a step back and looking at the companywide master data. To help generate excitement on the operations side, the commercial team can pick one or two specific examples of data that will generate meaningful insights and thus show the operations team the value of the data they have.
With this operational data as a starting point, companies can then look to combine it with specific external data. This might include potential customer locations, total revenue, industry dynamics, etc. One distributor, struggling to determine which customers its sales force and delivery teams should prioritize, combined its data on delivery sites with mobile data on foot traffic to identify locations with the highest potential spend. Combining insights like this with prioritization and rigorous outreach has driven growth by 5 percent or more in some markets.
Would you like to learn more about our Marketing & Sales Practice?
2. Invest in explaining the data and keeping it simple for the sales team
Sales teams typically have neither time nor the inclination to delve into data. Predictive models, for example, are often ignored because they are not 100 percent accurate; managers need to explain that even modest accuracy (predicting that 60 to 80 percent of customers are likely to leave, for example) can drive growth, help target customers, and improve sales reps’ compensation. Ultimately, for analytics to succeed, the complexity must be minimized. The sales team needs simple and effective insights and tools.
A North American logistics company had an overall goal of raising prices by 3 percent annually, but it knew that wide variation in growth and competitive intensity across its markets might make this difficult. To meet the target, it used analytics to develop a detailed “like customers” pricing scheme that provided simple rules based on each customer’s profile: reps were expected to raise prices on a certain type of customer while maintaining or even decreasing prices for a different type of customer. Customers were grouped based on a host of characteristics, including obvious ones like their size and location, but also less obvious ones like the product shipped, the destinations they needed to reach, and when they had volume spikes.
Reps embraced the new concept with great enthusiasm. The new approach also made performance management easier, as reps accepted that local market characteristics were being considered and that they were being benchmarked against peers from similar markets with similar customers.
Similarly, a cargo airline developed a complex micromarket strategy to categorize customers based on flight/space availability and demand. The airline’s new model essentially recognized which customers contributed more in challenging micromarkets and rewarded them accordingly. The impact of the analysis was tremendous. It helped the company identify opportunities for different negotiation strategies, raising prices for flights on routes with high demand or asking for higher-volume commitments on lower‐demand routes. To make it easy for the sales force, the company developed a simple performance dashboard for sales teams to manage pricing and volume negotiations with large customers by route on a daily basis.
Making analytics insights actionable for sales reps also requires coaching. The underlying analytics model can be hard to understand, but a second level of modeling (technically called a LIME or SHAP model) often makes it much easier to explain why a customer was selected or targeted. A transport company built a lead-generation engine that fused recommendations from three independent models. The new system published the characteristics and relevant context of each lead into one-page profiles that sales reps could then use in their conversations. This information also gave the sales manager a set of talking points or actions on which to coach the rep.
3. Embed analytics in daily routines
Behavioral change is particularly hard in T&L companies, where sales teams are distributed across many sites, rarely meeting in person at a terminal or office. For these people, there is often no formal way to build new capabilities. A distributor addressed this issue by building a routine around insights generated by a commercial analytics engine that identified pricing opportunity and churn risk. Reps were coached to sit down on Friday and plan their next few weeks based on the insights (such as prioritizing visits with customers with the biggest opportunity, or running a consistent play, such as addressing customers who needed margin improvement). Managers knew to ask reps how they prioritized their visits and then to ground their weekly coaching session in the analytics insights (what to say to get the best outcome, for example). The combined approach increased volume in its target segment while increasing margin (by reducing volume) in less-profitable segments, thus improving EBITDA in the business group by more than 30 percent.
Sales reps naturally want analytics they can understand at first glance, but the insights need to be presented in such a way that they can delve into the details when they need to understand what lies behind the recommendations. Allowing reps to dig deeper turns a report into a tool that enables them to develop and have confidence in their own insights. A year after one distributor introduced analytics, the sales reps recognized how much better they knew their customers because they had seen so much data over the previous 12 months and were actually clamoring for more.
Another critical component of embedding analytics is to hold people accountable for using them. That starts with setting targets, then showing the sales team the analytics-based recommendations on accounts, products, or actions that will help them reach the targets. Those targets should be coupled with incentives.
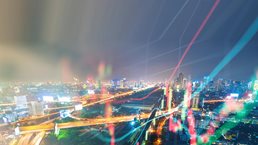
Boosting your sales ROI: How digital and analytics can drive new performance and growth
The key to getting started: Just get started
Even basic analytics can deliver value, so companies should not hesitate because they believe they need perfect data. After all, insights are useless if they take too long to develop. Nowhere is this more evident than when an analytics team disappears for weeks, only to resurface with some terribly clever analysis that neither they nor their internal customers know what to do with. We have seen extremely complex pricing and upsell models developed over six months, but we have also seen models developed in as little as six weeks that spark initial tests.
Many T&L companies lack the data-engineering capabilities to jump-start their programs and partner with third parties who can build the early algorithms. At the other end of the spectrum, one leading logistics company has hired more than 100 data scientists, embedding many in the business units to learn the business and crack its highest-value use cases. Whether companies want to do the data science in-house or outsource it, the most important step is simply to start. Advanced analytics can prove its value very quickly.
One T&L organization kicked off an analytics program by bringing together senior commercial leaders and people familiar with internal data to brainstorm what types of insights would be valuable. The team decided it wanted to understand what was driving customers to switch to other providers. The company then embarked on a four-week effort to clean and organize the relevant data by looking at the order and fulfillment histories of customers who had recently left. This was done by a relatively low-cost data engineer so was not a major effort.
The company then started a series of two-week sprints to build algorithms and generate insights. The commercial team reviewed them and helped to refine the models in an early stage. It was quickly time to test them in the field using a well-designed A/B pilot. Sales reps were given details on which customers were at risk of leaving and, based on the algorithm, recommendations on how to entice them to stay. For some it was a question of price, others had concerns about service quality, and some were not aware of the breadth of the company’s offerings and thought it could not meet all their needs. These insights were pushed to the sales team, who were coached to ensure they knew how to act on them. The pilot was successful, delivering a two-percentage-point uptick in revenue and a ten-percentage-point improvement in EBITDA.
Transportation and logistics companies have enormous potential to deliver commercial success by investing in advanced analytics to mine the data they already have, even if it’s not data that sits within the sales function. Up to 5 percent return on sales is achievable for firms that are able to make creative use of their existing data, keep insights simple for their sales teams, and embed analytics into their daily routines.