This is a pivotal year for pricing strategy. All those engaged in long-term contracts may want to consider reshaping their pricing approaches or risk seeing their profitability erode.
Big booms and big drops in core and semi-commodity pricing are now the norm, and everyone, from chemical manufacturers to producers of metal and mining players—is feeling the pinch. Take the example of polyethylene. Commercial teams who signed long-term contracts in 2020 using fixed index-based pricing were squeezed as prices soared by 50 percent. And buyers of this material who entered long-term purchasing contracts in 2021 quickly found themselves in upside-down arrangements after prices tumbled by nearly a third in the months that followed.
Similar trajectories have played out in many other categories across industries (Exhibit 1). As a result, pricing estimates have been way off the mark for many manufacturers, triggering earnings fluctuations and an uncertain revenue outlook. With turbulence expected to remain persistent, many recognize that traditional reliance on index-based data is no longer sustainable.
Instead, a more effective and sustainable solution can be to take a portfolio approach to pricing. McKinsey research has shown that applying predictive scenario modeling and optimizing for risk-adjusted returns can help manufacturers smooth earnings significantly. Some companies that have adopted this method have seen their return on sales grow by an average of 2 to 4 percent.
Would you like to learn more about our Growth, Marketing & Sales Practice?
Manufacturers are in a pricing predicament
Producers of commodities and near-commodities face pricing challenges in two main areas: getting the wide-angle view they need to hit their targets and getting the integrated insights they need to respond to changing market conditions.
A key reason is structural. It’s common for commercial teams to take a myopic, transaction-level approach to sales, negotiating terms on an individual-customer basis. The roots of this practice make sense as a means of accommodating for specific account and segment dynamics. But this approach may drive unforeseen complexity and reduce cross-transaction portfolio opportunities. A chemical producer, for example, had scores of product contracts, each with its own prices and terms. But the company was operating largely at the mercy of market forces because it didn’t have a way to assess potential risks or opportunities.
External data on demand drivers, trade flows, and materials cost trends, coupled with nonlinear modeling capabilities, would allow commodity producers to combine macro- and transaction-level data to price products for specific customers. But many companies lack these data assets and skills. A large packaging company, for example, tried to account for volatility by linking prices to three to five major market indices. But the indices didn’t adjust quickly enough for rising inflation, and contracts grew out of sync with the company’s higher production costs. This put significant pressure on profitability.
In addition, while options and derivatives can be used to hedge risk in publicly traded commodities such as corn and oil, these hedges may often not work in industry, because the financial instruments available in the market today cover limited products, often cost too much, and include terms that generally favor the issuer.
To gain better predictability, manufacturers may want to consider approaching pricing the way leading investors do: through a portfolio lens.
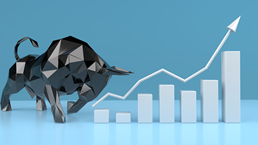
Five ways to ADAPT pricing to inflation
Price like a portfolio manager
Starting with their overall risk-and-returns objectives, many top investment firms traditionally construct their portfolios to match, taking on investments that reduce exposure in some areas and add it in others. The objective is to achieve a set of portfolios with maximum returns for a given risk profile, known as an efficient frontier. As markets change, firms can shift their portfolio composition and underlying assets dynamically. This portfolio orientation allows investors to allocate resources in areas where they can win.
Manufacturers can consider taking a similar approach—constructing balanced portfolios that satisfy their strategic priorities and setting individual contract terms based on their target risk-and-returns threshold.
Predictive modeling underlies this method. Using advanced multivariate models that can identify nonlinear relationships between market drivers, commercial teams test multiple market scenarios, pulling in data from internal and external sources to enrich their analyses. Once they discern which target portfolio performs best across a range of likely conditions, teams use optimization models to construct specific contract terms. These tools are adaptive, and most are automatable, which may make it easier for commercial teams to adjust recommendations and update contract negotiations in near-real time. These tools can also help leaders identify when contracts dip into noncompliance, enabling them to suggest potential remedies and engage proactively with customers.
For example, a North American chemicals producer set up a pricing center of excellence, staffed with data scientists, data engineers, and other technologists, to develop advanced decision-support tools for commercial teams. One of the first instruments they created was a portfolio simulation model. Testing it with one business revealed that shifting to market-driven prices instead of the cost-plus contracts they had been using had the potential to improve margin run rates by 4 to 5 percent without changing the company’s risk profile. Those insights shaped an action plan for an upcoming round of negotiations with customers. Within two months, the business unit’s earnings increased by 1 percent.
Improvements like these don’t need to involve a multiyear transformation. Instead, organizations we have worked with have been able to capture 2 to 4 percent upside, on average, by shifting to a balanced-portfolio approach, focusing first on the basics, then refining and expanding from there (Exhibit 2).
Five steps to consider
Becoming a data-driven commercial organization is a journey, but there are a few elements that manufacturers may consider putting in place to get started:
- Evaluate and consider revamp of existing contracts. Manufacturers that have a material portion of sales volume tied up in long-term contracts (longer than six months) may consider recrafting those agreements based on their organization’s goals for growing earnings, improving competitiveness, and optimizing profitability.
- Get in on AI/ML. Companies may have challenges gaining the pricing intelligence they need without robust data-and-analytics capabilities. leading data scientist teams can develop these models and data pipelines from scratch, and external models are also available for use across industry situations and technology environments.
- Integrate existing processes. Consider cutting slow-moving steps. Commercial teams at one petrochemical company, for example, used to have to run a gauntlet of meetings involving hundreds of slides to come up with pricing. By integrating insights into user-friendly digital dashboards, the company has lowered pricing response time from days to hours.
- Say yes to agile teaming. The most effective commercial teams pool expertise from across the business, bringing pricing, analytics, and product specialists together to improve the speed and quality of decision making.
- Upskill for the future. Portfolio modeling is a data- and analytics-intensive exercise. Many manufacturers may want to augment their capabilities in this area. Manufacturers can inventory their talent pool to find out where reskilling can close the skills gap and where new hires may be needed.
While slower movers may be more at the mercy of market forces, manufacturers that create dynamic portfolios aligned with their risk-and-returns objectives may better set themselves on a path to optimal performance. These are the companies that may find themselves going from playing the odds to turning them in their favor.