When AI emerged from its long winter and sprung onto business agendas in the 2010s, a scarcity of data science talent put considerable constraints on how and where business leaders could apply the technology. While AI talent challenges remain, strides have been made and many lessons have been learned that can be applied to tech talent strategies overall.
An arguably wider talent gap in quantum technology threatens to stall progress on breakthrough quantum use cases, jeopardizing the creation of a massive amount of business value. Quantum computing alone, which represents the largest market potential for the three mains areas of quantum technology (the other two areas being quantum sensing and quantum communications),1 could account for up to nearly $700 billion in value.2
Our research finds that there is only one qualified quantum candidate available for every three quantum job openings (Exhibit 1).3 By 2025, we predict that less than 50 percent of quantum computing jobs will be filled unless significant interventions occur.
Quantum start-ups and established tech companies—especially those in the hardware market, where more than half of today’s quantum investments are concentrated4—have been the first to wrestle with this talent crunch as they race to solve fundamental questions in the field and deliver fault-tolerant quantum systems, which are necessary to unlock the technology’s full potential. It’s still early days, with many unknowns, but the technology is rapidly progressing. With this progress, the demand for quantum talent is shifting, first to software companies and then to enterprises that will use the technology. Leaders across industries are already beginning to assemble quantum teams and test early-stage algorithms on the current class of quantum systems. This includes, for example, exploring how quantum algorithms can improve encryption protocols in financial services, optimize routes and fleets in logistics, and improve clinical-trial site selection in pharmaceuticals.
As we saw with AI, the growth of graduate programs in the field will be one necessary development to ensure a robust talent pipeline. Quantum graduate programs are still not widely available or accessible at the university level. Today, of the 176 quantum research programs at universities worldwide, only 29 provide graduate-level degrees in the subject.5
But beyond this, many of the same strategies and investments that companies have used to successfully build their AI teams, such as upskilling workers and creating pathways for new talent, can also serve them well when building quantum teams.
Based on data and insights from our research and work in the field,6 along with the experience of The Coding School’s quantum computing education initiative, Qubit by Qubit, there are five lessons from the AI talent journey that can help organizations build the quantum talent they need so they’re ready to capture value as the technology comes of age.
Would you like to learn more about McKinsey Digital?
Lesson 1: Define your talent needs clearly
In the early days of AI, some organizations hired data scientists without a clear understanding of what skills they actually needed. As a result, many struggled to realize a return on their investment. For example, one large financial services firm’s exuberance for AI led it to hire nearly 1,000 data scientists. After the organization did not realize the outcomes it expected, leaders discovered that many of the new hires were not data scientists by strict definition and that just 100 true data scientists placed in the right roles would have been sufficient.
In the case of quantum, talent needs are still evolving, but once organizations are ready to assemble their teams, most will likely need to build out their stable of quantum business and strategy experts along with technical talent in two areas: quantum software engineering and quantum hardware engineering (Exhibit 2):
- Quantum software engineering. Organizations will require quantum software engineers who can build new quantum algorithms, as well as those who can improve existing quantum algorithms or conduct exploratory research on potential quantum applications. Some positions, such as those responsible for developing quantum algorithms from the ground up, will require PhDs. Others, however, will be achievable through upskilling with general quantum training. For instance, systems architects, AI and machine learning algorithm developers, and others with traditional coding and AI skills can be upskilled in as little as six months to code and improve quantum algorithms using tools such as Cirq, Qiskit, and Q# and could begin exploring how quantum could provide an advantage in their industry.
- Quantum hardware engineering. Companies will need experts overseeing systems based on entirely different physical processes than those of conventional computers. As companies form partnerships with quantum vendors to run their applications on quantum computers, they’ll need quantum hardware experts who can configure and manage these systems, just as cloud engineers do today. This work will likely require a PhD until quantum systems become plug and play. Over the long term, we anticipate that hardware experts with a foundational understanding of quantum concepts (such as qubits [quantum bits], algorithms, and encryption), as well as those who have completed coursework or upskilling in role-specific topics (such as assembly and testing of quantum computers), will fill these roles.
In defining talent needs, organizations should first identify possible fields of applications that the quantum computing team will work on. At one large global pharmaceutical company, the CTO worked closely with colleagues in research and development and IT to assess areas where they were already pushing classical computing methods, such as machine learning and high-performance computing, to their limits. They agreed to focus the quantum computing team on computational tasks in drug discovery, with the ultimate goal of finding drugs that bind better to their targets—long considered the “holy grail” in the pharmaceutical industry. The newly formed taskforce, including HR experts from all around the world, created a list of the types of expertise and backgrounds required to successfully build quantum algorithms from the ground up in this area. This list included five team members who come from diverse backgrounds and ideally bring quantum chemistry and quantum computing expertise, along with a mixture of experience in designing quantum algorithms, identifying viable use cases, and securing venture capital funding (the last of which provides a view into the evolving world of quantum partnerships). The list also included a combination of academic backgrounds—useful for building scientific connections with universities—and applied experiences, such as experts from quantum computing companies involved in creating the technology.
Over the next nine months, the pharmaceutical company conducted targeted outreach through job postings, partnerships, active sourcing, and networking. The organization empowered the first two hires to identify and recruit the remaining team members. Today, this multidisciplinary team has a pipeline of use cases to explore as it works to understand how quantum computing can make a difference in the pharmaceutical industry.
Lesson 2: Invest in translators early
As excitement for AI grew, the role of analytics translators7 emerged as a key to helping leaders first identify and prioritize the business challenges best suited for AI to solve and later facilitate the development and adoption of these AI solutions. In the field of quantum, we see a need for translators with engineering, application, and scientific backgrounds who can help organizations make sense of a rapidly expanding ecosystem of opportunities and players. While upskilling quantum talent with domain knowledge would be ideal for this role, given that quantum talent is scarce, companies can also look to upskill adjacent roles, such as computer engineers, application developers, and chemical researchers, with general quantum training.
One immediate area of focus for quantum translators is helping their companies stay attuned to industry developments and identify how and when to jump in. Quantum computing’s potential to process exponentially more data and assess more potential solutions faster than any system available today could enable game-changing advances across industries—from dramatically reducing drug discovery timelines8 and improving supply chain efficiency to sparking the development of breakthrough products, such as green fertilizers for the agriculture industry.9 And as with AI, we anticipate a clear first-mover advantage for those prepared to capture this potential. Translators can help businesses answer important questions such as the following: What risks and opportunities will we face as quantum technology matures? Which use cases will deliver the highest value? What investments are necessary today so we don’t fall behind tomorrow?
Another area where translators can add tremendous value today is in guiding their organizations on strategic partnerships with quantum software and hardware companies. On the software side, this includes identifying and negotiating partnerships with organizations doing research on quantum applications in their industry so that their business problems can be included in test cases. On the hardware side, translators will be needed to identify which quantum technologies are best suited for any early-stage algorithms that experts in their organizations build. Different platform approaches, such as using trapped ions versus ultra-cold atoms for qubits, can result in varying levels of technical noise in quantum components that affect algorithmic speed, connectivity, and outcomes. In the near term, organizations will need to pay close attention to such differences or else they may realize suboptimal outcomes from their efforts. In the longer term, it is expected that fully fault-tolerant quantum computers that automatically correct for these errors will be developed.10
We find that engineers, application developers, and those in adjacent fields, such as statistics, computer science, and chemistry, can obtain the foundational knowledge needed to be a quantum translator in just three to six months. A 26-week introductory course on quantum computing, for example, provided one computer engineer in healthcare IT the foundational knowledge he needed to develop a strategic presentation and road map on how the sector could benefit from this emerging technology. He delivered his presentation at a developers’ conference, where the managing director of a healthcare IT provider took note and hired him. He now aspires to advise companies on how quantum technology can support their business goals.
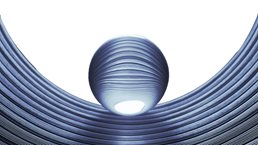
The Rise of Quantum Computing
Lesson 3: Create pathways for a diverse talent pipeline
As AI applications increasingly became part of everyday life, it became painfully clear that organizations needed to do more to attract individuals from underrepresented groups to AI’s foundational fields of computer science, math, and statistics. AI models (and the data feeding into them) caused numerous instances of unintentional bias and discrimination—for instance, the exclusion of underrepresented populations from hiring considerations by AI-driven recruiting systems—that were due, at least in part, to a lack of diverse perspectives and experiences around the table as the AI models were built and tested.
It’s too early to know what risks will emerge from quantum technologies, but we expect similar challenges if we don’t build a diverse quantum workforce. This will require efforts to bolster diverse representation in quantum-adjacent fields, including not only computer science, math, and statistics but also physics, material science, and chemistry.
Such efforts are needed at both the university level and in K–12, in which early intervention has been shown to have a significant effect on the talent pipeline. Qubit by Qubit, for instance, saw a 32 percent increase in middle schoolers interested in pursuing a career in quantum computing after running a one-week virtual summer camp. The organization also ran a four-week summer school program, which enrolled a diverse population of high school and undergraduate students, with 48 percent of participants identifying as female or nonbinary, 20 percent of students identifying as Hispanic, and 17 percent identifying as Black. Following the program, there was an 86 percent increase in students’ interest in pursuing a career in quantum computing and a 74 percent increase in students’ interest in taking additional courses in quantum computing.11
When high school students were asked what excited them about quantum computing, one of the most popular responses was that it has the potential to affect other fields they are interested in, suggesting an opportunity for leaders to engage with students who want to make a difference in their industry.12 IBM, for example, has collaborated with Qubit by Qubit over the past three years on an introduction to quantum computing course, which covers the foundations and applications of quantum computing. More than 6,000 high schoolers have participated in the course to date, and the majority say they are interested in pursuing a career in a quantum information science and engineering field as a result. Additionally, some of these students have since entered college and are now participating in internships at IBM and other organizations.
While such efforts are making a dent, ultimately, we envision school systems that provide all students with exposure to quantum technologies through mandatory lessons, units, or courses; currently, even when extracurricular courses are offered, students are often intimidated by the complexity of the subject and may not think they’re qualified to participate. For example, in a post-session interview with students who successfully completed Qubit by Qubit’s introductory course, one student shared how nervous he was when first applying for the program because he thought this area of study was meant for “geniuses.” Another wrote that, prior to the course, he believed that the topic of quantum computing was “something that I will never understand.” Following the course, their perspectives changed, and they saw quantum as an area of study that they could succeed in.
Some US states are moving to incorporate quantum learning in their schools. Ohio, for example, is the first US state to add quantum training to its K–12 science, technology, engineering, and math (STEM) standards; the Cleveland Metropolitan School District, in collaboration with Qubit by Qubit, is now training K–12 teachers and university faculty at Cleveland State University and offering students an introduction to quantum computing course for credit. Business leaders can influence this shift significantly, given that schools often see corporate involvement as a sign that this will be an important field, even if they don’t yet know what quantum is. (For more on what it takes to build a diverse quantum workforce, see sidebar, “Building a diverse quantum workforce: An interview with IBM Quantum’s Bradley Holt.”)
Lesson 4: Build technology literacy for all
As AI came of age, organizations found that everyone across the business needed a basic understanding of how the technology worked and what it could do. Without this knowledge, decision makers often were skeptical of the technology, and its outputs and innovation suffered.
The same is true for quantum technology. Business leaders will need a foundational understanding of the technology to deftly steer their companies and investments in the quantum era. Likewise, workers in supply chain, marketing, IT infrastructure, finance, and other core domains and functions will require basic fluency in quantum topics to engage with quantum experts as they work to solve complex business challenges.
Given how quickly the technology is evolving, such efforts will likely require that organizations combine targeted capability building—such as workshops to ground business leaders in the possibilities and realities of this emerging technology—and ongoing knowledge sharing. For instance, the previously mentioned pharmaceutical company’s quantum lab conducts “knowledge hours” where team members share their work with business and IT teams and answer questions about the technology. This effort gave rise to a small academy, led by inspired quantum enthusiasts outside the lab, that teaches quantum computing foundations to interested employees, enabling the organization to keep the fire burning. The lab also sends regular team communications about its work to leaders to keep them informed and connect the work to the company’s purpose. Finally, the team ensures that every scientific study includes an abstract, introduction, and conclusion that can be easily understood by any layperson and connects the work to the company’s purpose and real-world application.
Lesson 5: Don’t forget talent development strategies
Often, as new technologies arrive on the scene, companies focus heavily on talent attraction in a scramble to stitch together the new skills and knowledge they need. As we saw with AI, getting experts in the door is only one piece of the tech talent puzzle. Companies should also carve out clear paths for talent development to ensure technical experts stay with the organization and keep their skills current.
To retain highly specialized experts, the same pharmaceutical company leans into both the purpose of its work—developing real use cases that will save lives—and the freedom it offers its team. This includes the option to work from anywhere in the world and the freedom to choose what questions they work on as they explore how quantum technologies can make a difference in human and animal health. The researchers are encouraged to publish their findings, take part in conferences and workshops to keep building their skills, and actively build collaborations with world-leading academic groups and companies. Dialogue with other researchers is a key strategy to stay up to date with the latest developments and leads to new ideas and approaches.
Developing a quantum workforce is a multifaceted issue. In the near term, leaders can overcome some of the current talent gap by upskilling workers in related disciplines. Over the long term, corporate investments that create pathways for diverse talent will be crucial to ensure a robust talent pipeline is available as the quantum era goes into full swing. But as we discovered through AI, now is the time to prepare, and those who wait risk falling behind.