Asset-intensive industries—such as chemicals, oil and gas, mining, metals, pulp and paper, and power production—have been turning to new technologies in their effort to increase the reliability and availability of their equipment, while keeping maintenance costs under control. Using digital tools and advanced-analytics capabilities alongside traditional lean techniques, they aim to predict and prevent equipment failures, increase labor productivity, and streamline the management of external contractors.
When companies get all of the requirements right, the results can be impressive. Leading players in a variety of heavy industries have used digital tools in dramatically reducing unplanned outages while boosting maintenance-labor productivity. Higher availability and a more-efficient workforce have increased profitability by 4 to 10 percent in some organizations.
But these lighthouse examples are the exception rather than rule. Many companies that have implemented digital maintenance and reliability solutions have seen little measurable impact. What makes the difference?
In our experience, the most successful digital maintenance and reliability implementations share three common characteristics. First, these companies take a holistic view of these new tools, building digital technologies into a clearly defined vision for the future of the maintenance and reliability function. Second, they focus on two domains that have proved to be truly effective in heavy industrial applications: predictive maintenance (PdM) and digital work management (DWM). Finally, they support their use of digital tools by ensuring the necessary enablers are in place, including optimized workflows, a robust data infrastructure, and the capabilities of their personnel (exhibit).
A closer examination of the approach reveals important details that the early adopters got right as part of a wider transformation of their maintenance and reliability functions. In particular, they focus on how DWM and PdM generate value, using these digital tools to reimagine entire processes and better meet users’ needs.
Digital work management: work gets done faster, cheaper, better
DWM encompasses a wide range of systems that enhance maintenance work, covering everything from work identification and planning through to scheduling, materials management, dispatching, execution, and closeout. Typically, DWM is a built into, or on top of, the organization’s existing enterprise resource-planning (ERP) platform. The ERP system serves as a source for much of the foundational data used by DWM, including structured equipment data, spare-parts inventory, personnel data, and preventive-maintenance schedules.
The DWM system then optimizes job prioritization, job planning, job assignment, permitting, scheduling, and dispatch, based on constraints such as the competencies of available maintenance personnel and availability of parts. These systems also provide execution support in the field, with mobile devices or augmented-reality systems guiding staff as they complete their tasks, collecting detailed data on asset condition, and improving the accuracy and speed of work-order closeout and contractor payment.
Successful DWM systems deliver value by increasing the efficiency of internal and external maintenance labor, reducing planned downtime through shutdown and outage optimization, and providing the opportunity to upskill the maintenance workforce. Taken together, these levers can lead to cost reductions of 15 to 30 percent. Secondary benefits include more effective procurement and working-capital management through improved inventory visibility.
Would you like to learn more about our Operations Practice?
How DWM worked for a chemicals company
One global chemicals company, for example, introduced a bolt-on DWM system that seamlessly interfaced with its ERP platform. Implemented in just five weeks, including user training, the new system transformed the company’s maintenance planning, scheduling, and work execution. Productivity in the maintenance planning and scheduling department increased by 30 percent, and the organization doubled the number maintenance tasks that were completed on schedule.
To maximize the impact generated by their investment in DWM, successful organizations like this take a number of essential actions. Most importantly, they redesign their maintenance processes in parallel with the introduction of the new technology. Redesigning the process to fully leverage DWM technology will almost invariably produce a more efficient outcome than simply digitizing the existing paper-based process.
Getting the most from DWM
In the design and implementation of the new system and processes, successful organizations take a perspective that is both value-centric and user-centric. While the overriding aim of DWM is to reduce waste and address pain points for the business, that won’t happen if adoption is poor. Therefore, successful systems must also make life easier for of users, such as maintenance and operations staff or external contractors. That requires a good understanding of their working practices, challenges and pain points.
Finally, successful companies build tight, two-way integration between their DWM tools and their other systems, such as computerized maintenance management systems (CMMS). This ensures that data captured in the field become part of the organization’s master data, and can be accessed and analyzed to aid OEE tracking, condition monitoring, and root-cause analysis of reliability issues.
Predictive maintenance can prevent failures
Encompassing a wide variety of methods used detect the early signs of problems in equipment, PdM allows operators and maintenance staff to take action before a failure occurs. In today’s heavy industry, PdM techniques have become common—but most companies are using lower-maturity approaches, such as sensors on machines that trigger alarms when vibration or temperature thresholds are exceeded. More advanced approaches attempt to predict failures by analyzing sensor data to identify the “signatures” of known failure modes. At the highest level of maturity, PdM systems apply an array of machine-learning and advanced-analytics techniques to identify and categorize issues, and to provide actionable insights to operations and maintenance teams.
Successful PdM programs reduce the requirement for both planned and unplanned maintenance interventions. That cuts maintenance costs, and increases production and throughput (especially valuable for assets that are capacity constrained). In addition, these programs can increase the useful life of high-cost components and reduce safety risks related to machine failure events.
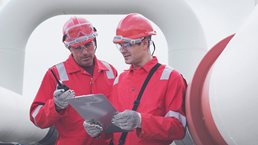
The right tools for every job: Lean and agile in maintenance
PdM yields results in oil and gas
One leading offshore oil and gas operator introduced a sophisticated PdM system across nine platforms in Africa and Latin America. Using data collected from 30 years of platform operations, the company identified critical assets that it wanted to immunize against failure. It then developed and refined its PdM approach on one platform before rolling it out across its fleet. The effort resulted in a 20 percent average reduction in downtime and production increases of more equivalent to more than 500,000 barrels of oil annually—across a fleet that was already in the top-quartile of performance for the sector.
These results required a significant data-science effort. Over a period of two years, a team of ten to 15 data scientists built more than 500 advanced-analytics models. Each model was then tested and refined to achieve an acceptable level of false positive warnings. The typical initial precision of the models was too low to ensure acceptance by frontline workers, but data scientists and maintenance teams worked together, failure by failure, to refine their design and eliminate false positive results.
Although high-maturity PdM approaches such as these have now been proved at scale, their complexity should not be underestimated. Successful implementations require a certain level of data history, sensor deployment, near-real-time data streaming, and a high enough value of downtime to provide an attractive ROI. This is the case in most upstream oil and gas facilities, large refineries, petrochemicals plants, and similar operations in power generation (traditional and renewables), paper mills, and mining.
Less-intensive PdM alternatives can overcome data limitations
For operations where PdM may not yet be economic, less data-intensive methods of PdM, such as anomaly detection, can be successful as a first step. These methods are faster to implement but provide significantly lower predictive power, with high numbers of alarms that are low priority or difficult to act upon. Depending on the situation, that can lead to unnecessary work and reduce workforce confidence in the recommendations. Lower-maturity PdM systems typically deliver only a fraction of the benefits (10 percent or less) of a fully-scaled system. Ultimately the decision on where and at what level to implement predictive maintenance requires an asset-by-asset validation of the potential benefits and data availability.
Companies with advanced PdM ambitions should also look for the right partners. The approach typically requires more knowledge, data, and development investments than other digital and analytics use cases. And, as the value of reliability for large industrials typically lies in a wide range of equipment and failure modes, a large and complex set of PdM models is required. For most organizations, developing and refining these models in-house will be an extremely expensive and time-consuming process. Instead, there are partners that can provide substantial intellectual property and data, significantly reducing both the time to impact and the investment required.
As with digital work management, the people side of predictive maintenance is as important as the technology. Alongside the development of the software and hardware elements of the system, the oil and gas company launched a comprehensive process-redesign and capability-building effort to measure the impact of the approach and embed new ways of working into its daily operations. In our experience, such a change-management strategy is a central part of every successful PdM implementation.
Finally, leading companies also integrate predictive maintenance with their other operations and maintenance systems. Ideally, the link between PdM and DWM systems should be tight, with PdM outputs triggering work requests in the in DWM systems, and data from the DWM used to refine and improve predictive models.
Unlocking the potential of digital and analytics in maintenance and reliability is not easy, but leading players in heavy industries have enjoyed significant rewards for their efforts. In future articles, we will take a deeper dive into digital work management and predictive maintenance, to uncover the secrets for success in each domain.