The engineering and construction (E&C) sector is worth more than $10 trillion a year. And while its customers are increasingly sophisticated, it remains severely underdigitized. To lay out the landscape of technology, we conducted a comprehensive study of current and potential use cases in every stage of E&C, from design to preconstruction to construction to operations and asset management. Our research revealed a growing focus on technological solutions that incorporate artificial intelligence (AI)-powered algorithms. These emerging technologies focus on helping players overcome some of the E&C industry’s greatest challenges, including cost and schedule overruns and safety concerns.
Stay current on your favorite topics
In the immediate future, we expect AI’s proliferation in the E&C sector to be modest. Indeed, despite proven high return on investment (ROI) and widespread management interest in AI solutions, few E&C firms or owners currently have the capabilities—including the personnel, processes, and tools—to implement them.1
However, a shift is coming. Stakeholders across the project lifecycle—including contractors, operators, owners, and service providers—can no longer afford to conceive of AI as technology that’s pertinent only to other industries. Indeed, adjacent industries, such as transportation and manufacturing, are already in the process of breaking down the barriers between one another and operating more as ecosystems (for example, solutions, tools, and algorithms that were industry-specific are more likely to become effective having impact across industries)—increasing the threat of competition from market entrants that have not traditionally been capital project players.2
These lowered market barriers are compounded by the increasing ability of AI methods to work across industries. These advances will be seen in the mid- to long-term, but to play a role in future ecosystems—and to compete with incoming market entrants—E&C will need to catch up in its adoption of AI applications and techniques. We predict this effort will lead to the allocation of more resources to build the necessary capabilities, and to AI playing a more significant role in construction in the coming years.
So where should E&C leaders begin? Building on last year’s report, we offer predictions for where and how AI can infiltrate construction across three categories:
- Examining where AI solutions are beginning to emerge in construction today.
- Exploring AI-powered applications and use cases that have already made an impact in other sectors and that can be applied in the construction industry.
- Assessing additional machine learning algorithms and their potential E&C applications.
The current state of AI in engineering and construction
AI use cases in construction are still relatively nascent, though a narrow set of start-ups are gaining market traction and attention for their AI-focused approaches. There are a few early-stage examples construction firms can evaluate:
- Project schedule optimizers can consider millions of alternatives for project delivery and continuously enhance overall project planning.
- Image recognition and classification can assess video data collected on work sites to identify unsafe worker behavior and aggregate this data to inform future training and education priorities.
- Enhanced analytics platforms can collect and analyze data from sensors to understand signals and patterns to deploy real-time solutions, cut costs, prioritize preventative maintenance, and prevent unplanned downtime.
Still, adoption of AI solutions is quite low in E&C, particularly compared with other industries (Exhibit 1). McKinsey research compared building materials and construction to 12 other industries; ten of those industries are further along in current AI adoption, and all 12 are projected to increase spending on AI at a faster pace over the next three years.
Of course, any AI algorithm is based on learning from the past. This means that AI needs a certain critical mass of data to deliver on its promise so scale will matter; as such, firms will need a significant amount of data (in this case projects) to train an AI algorithm. Therefore, the largest companies are likely to benefit more, particularly in the short term.
It is possible that an external third party enters and leverages E&C data to train its models—a scenario that would likely result in improvement across the industry as a whole but limited competitive advantage for individual firms—but this seems unlikely given the enormous restrictions on data sharing and data ownership.
Five AI-powered applications from other industries transferrable to construction
AI encompasses a large universe of possibilities and use cases, including machine learning, natural language processing, and robotics. Our research has homed in on five AI applications used in other industries that have direct application in the construction sector:
Transportation route optimization algorithms for project planning optimization
Currently available technology already offers transportation companies the ability to optimize routes and improve traffic navigation. In the future an AI technique called reinforcement learning, which allows algorithms to learn based on trial and error, could provide even more effective optimization as well as solve for objective functions (e.g. duration or cost of fuel).3 Such technology could be directly applicable to E&C project planning and scheduling, as it has the potential to assess endless combinations and alternatives based on similar projects, optimizing the best path and correcting themselves over time.
Would you like to learn more about our Capital Projects & Infrastructure Practice?
Pharmaceutical outcomes prediction for constructability issues
The pharmaceutical industry has emerged as a leader in investing its large R&D budgets into predictive AI solutions, which lower R&D costs in the long run, chiefly by forecasting medical trial outcomes. These applications can be directly applied to the construction industry—particularly in major projects with R&D budgets as large as those of Big Pharma—in two ways to forecast outcomes. First, predictive applications can forecast project risks, constructability, and the structural stability of various technical solutions, providing insight during the decision-making phase and potentially saving millions of dollars down the road. And second, these applications can enable testing of various materials, limiting the downtime of certain structures during inspection.
Retail supply chain optimization for materials and inventory management
AI has changed the game for the retail supply chain by reducing manufacturing downtime, reducing oversupply, and increasing predictability of shipments—all resulting in impressive reductions in costs, logistical burdens, and variability. Supervised learning applications (e.g., gradient-boosting trees4 ) will become directly applicable to E&C as modularization and prefabrication become more prevalent. More projects are using off-site construction for large quantities of materials, and the need for enhanced supply chain coordination will become critical to control costs and overall cash flows.
Robotics for modular or prefabrication construction and 3-D printing
While use of modularization and 3-D printing is advancing in construction today, there could be a longer-term opportunity to maximize the benefits of these approaches through machine learning. For example, robotics industry researchers have successfully trained robotic arms to move by learning from simulations.5 In E&C, this application might someday be applied to prefabrication techniques and maintenance operations for oil and gas as well as other industrial sectors.6
Healthcare image recognition for risk and safety management
In the healthcare industry, machine-learning methods are creating breakthroughs in image recognition to support the diagnosis of illnesses (e.g., detecting known markers for various conditions). Down the road, this technology could be applied to drone imagery and 3-D-generated models to assess issues with quality control, such as defects in execution (both structural and aesthetic) and early detection of critical events (e.g., bridge failure). These techniques could help engineers compare developing and final products against initial designs, or train an unsafe-behaviors detection algorithm to identify safety risks in project sites based on millions of drone-collected images.
Additional machine learning algorithms with potential to disrupt E&C
The number of AI solutions applicable to E&C are potentially endless. To scratch the surface, we offer a focused look at a few of the possibilities in machine learning (Exhibit 2).7 While machine learning is but one branch of AI, its breadth of supervised and unsupervised learning techniques, as well as deep learning convolutional and recurrent neural networks, offer myriad business cases for investment.
Several use cases will be applicable across the broad spectrum of E&C stakeholders, including owners, contractors, and operators:
Refining quality control and claims management
Firms can use deep-learning techniques to enhance quality control. Neural networks can, for example, assess drone-collected images to compare construction defects against existing drawings. These networks are also capable of helping owners and firms alike understand the likelihood that a contractor or subcontractor will file a claim, enabling owners and firms to proactively allocate contingencies and deploy targeted mitigation plans.
Increasing talent retention and development
One major challenge the E&C industry will face over the coming years is attracting and retaining top talent. Leaders can tackle this issue by applying both unsupervised machine learning algorithms such as Gaussian mixture models, which can segment employees based on likelihood of attrition, and developing targeted plans to retain them. K-means clustering can identify potential candidate pools and tailor recruiting strategies to attract the right talent. AI algorithms can also help leaders locate and predict overarching talent pain points such as turnover, skill or labor shortages, and flaws in organizational design. For example, it might help forecast labor shortages for skilled craft in specific geographies, or plan for hiring or locking contracts to limit costs or project delays.
Boosting project monitoring and risk management
E&C stakeholders can use neural networks, using drone-generated images and laser generated data capturing project progress, to teach an AI how to create 3-D “twin models” to match BIM-generated models. These applications would dramatically reduce decision-making cycles in a construction project from a monthly basis to a daily basis—through full automation of the project scheduling and budgeting update on the combination of BIM, AI, drone, and laser capabilities.
Constant design optimization
Owners and contractors can employ a recommender system approach (supervised learning) that uses cluster behavior production to identify the important data necessary for making a recommendation. These applications can recommend to engineers and architects the use of a specific design, such a structural solution (for example, type of connections—welded or bolted) or an architectural finishes (for example, curtain walls vs window walls) based on various criteria (for example, total cost of ownership, timeline to complete execution, likelihood of defective constructions-mistakes during execution). The end result is that owners and contractors have more information with which to make an informed decision.
Several other applications have a specific use case for E&C contracting firms:
Building commercial excellence and a competitive edge
By assessing previous project bids and replicating elements of the successes while avoiding elements of the failures, supervised and unsupervised learning algorithms can boost an E&C firm’s project win rate, enhance margins, and ensure project value. Linear/quadratic discriminant algorithms, for example, can enhance a firm’s forecasting ability to estimate a lead’s likelihood of being accepted (i.e. go/no-go ratio) and likelihood of closing (i.e. get/no-get ratio). Simple neural network algorithms can be used to assess the rates or lump-sum price discounts clients may be willing to pay for a project, while in the future, reinforcement learning could help optimize bids and designs based on prior successful bid decisions. These algorithms can also predict what combination of services might be most attractive to clients, particularly as firms move toward offering integrated solutions rather than traditional one-off projects.
Firm reputation and risk management
Given the recent wave of earnings misses and project write-offs in the E&C industry, the confidence of the market and individual clients in a given firm’s ability to meet commitments has dropped. Because of this shift, firms are losing project bids and the market is penalizing stock prices. Firms can apply machine learning to rapidly address market and client concerns. For example, Naïve Bayes algorithms can be employed to perform sentiment analysis on a firm’s market perception and inform the launch of targeted, reputation-building efforts needed to preserve its backlog and stock price. Algorithms can also be used to profile customers based on their characteristics and desires to better target business development efforts and improve retention.
What leaders can do to get ahead of the curve and take advantage of AI
There are several steps that all stakeholders can take to get ahead of the curve in AI:
Identify high-impact use cases based on a firm’s starting points
Firms need to identify the areas of major need and what AI-powered use cases can have the most impact in the short term. Without a clear business case, ROI, and burning platform, E&C firms will be inefficient in the use of time and resources, which can create frustration, increase skepticism in the organization, and cause firms to lose momentum. Leaders should prioritize their investments based on the areas where AI can have the most impact on the firm’s unique situation and need—for example, safety or talent retention—and where it will be easiest to implement in the firm’s current stage of digital maturity.
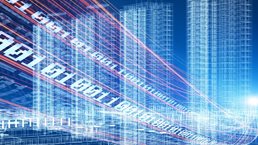
The new age of engineering and construction technology
Dedicate a significant portion of R&D investment to digital capabilities immediately
Today, the E&C industry is investing roughly 1 percent overall into technology—a significantly smaller proportion than other industries, such as financial services and manufacturing. Because the impact of AI is contingent on having the right data, E&C leaders cannot take advantage of AI without first undertaking sustained digitization efforts. This includes investing in the right tools and capabilities for data collection and processing, such as cloud infrastructure and advanced analytics. McKinsey research finds that companies with a strong track record of digitization are 50 percent more likely to generate profit from using AI.
Embrace the ecosystem concept and understand solutions from other industries
For too long, the E&C sector has operated within a vacuum. Given the move toward ecosystems discussed above, industry insiders need to look beyond sector borders to understand where incumbents are becoming more vulnerable and to identify white space for growth. Both owners and E&C firms can explore nontraditional partnerships with organizations outside the industry to pool advanced R&D efforts that have multiple applications across industries (for example, start-ups, universities, or even major players in other sectors where AI is more evolved). For E&C firms that can pursue unsolicited bids or real-estate development, such partnerships could be a way to increase data points and generate value. In addition, owners and firms can ensure corporate development teams have the talent and topical expertise to assess potential technologies with the entire ecosystem in mind.
Adapt the talent capabilities of the company
The industry will need to reverse its trend of underinvesting in developing talent and place significant focus on hiring people from other industries with backgrounds and skill sets in AI and digital technologies. In addition, firms will need to reskill their current workforces to acquire the necessary capabilities to thrive in the digital age and provide training in necessary concepts, such as machine learning algorithms.
Change internal processes to accommodate the innovation that AI will bring
Today, the processes critical to actualizing AI solutions—such as how to propose and implement a new idea—are handled several levels below the CEO. But top leadership needs to be involved in developing these processes and bolstering employees’ flexibility to innovate. While seemingly a simple step to take, ensuring the C-suite is influencing process development is a key enabler of preparing to embrace AI.
First movers and fast followers will be rewarded
The concrete steps outlined above can serve as an immediate starting point for firms to pursue AI. Indeed, early movers will set the direction of the industry and reap both short- and long-term benefits. Though E&C tends to lag behind by measure of technology adoption, now is the time for owners and firms to act and secure their places at the vanguard of pulling AI applications and techniques into the sector.