In the chemicals industry, like many others, there is considerable excitement about the potential of advanced predictive-maintenance (PdM) approaches. The promise of these new techniques is tantalizing. Using machine-learning technologies to comb through historical performance and failure data, they aim to tell operators when and how a component is likely to go wrong in the future with a high level of predictability. This should reduce the impact of equipment failures—and the cost of efforts to prevent such failures—by turning inefficient, unplanned maintenance activities into efficient, planned ones.
Stay current on your favorite topics
At first sight, chemicals plants seem like the ideal environment for PdM. High levels of automation and instrumentation, combined with rigorous maintenance record-keeping, create the rich data that machine-learning systems require. Moreover, most plants strive for stable operating conditions, potentially making it easier to spot patterns and trends. There’s also a compelling business case for improved reliability. Overall equipment effectiveness (OEE) losses due to unplanned maintenance range from 3 to 5 percent across the industry.
Predicting poor results
Take a closer look, however, and the potential of PdM in chemicals begins to evaporate, for four main reasons.
- Too little data. In a chemicals plant, predicting failures is harder than it first appears. Unplanned downtime is typically concentrated in a small number of large events. That means there are typically too few datapoints for PdM systems to learn from.
- Too little time. Even when it’s possible to create models with predictive power, they often work over time horizons that are too short to be useful in chemicals manufacturing. Predicting that a part will fail in two days or two weeks is useful in a truck or machine tool, but it may not help in a plant where shutdowns take several days and maintenance teams require months to plan interventions and source spare parts.
- Too little impact. The impact from PdM is often low because plants operate critical assets with a high degree of redundancy and few single points of failure. If a pump stops unexpectedly, operators can often switch to a backup unit with little impact on production.
- Too little savings. Finally, a focus on reducing unplanned downtime ignores the largest source of throughput losses in most plants. Shutdowns for planned maintenance events cause OEE losses of 5 to 10 percent on average, twice as much as unplanned stoppages.
Would you like to learn more about our Operations Practice?
Towards digital reliability
Do these challenges mean analytics provides little or no value in efforts to improve asset productivity in the chemicals sector? No. The industry is achieving considerable success with a range of digital reliability techniques, many of which are far cheaper and less complex to implement than advanced PdM. Take three prominent examples:
Condition monitoring
Improving condition monitoring through better remote sensing can cut mean time-to-repair, significantly reducing the impact of equipment failures. At one chemical plant, a few critical pumps suffered repeated failures. No backups were available for these units, and the issue was a significant source of production losses at the plant.
“We decided we couldn’t wait for the plant and reliability engineers to identify the root cause, redefine the pump’s technical specifications, and then procure replacements,” the plant’s maintenance manager told us. “So, we focused on mitigating the impact of the failures, rather than avoiding them.”
The plant’s reliability team installed a handful of new sensors on the pumps and started to monitor their condition online in real time, allowing them to detect imminent failures a few hours before they occurred. By enabling maintenance personnel to be ready to intervene, this intervention reduced the mean time-to-repair on these pumps from 6.5 to around 3 hours, cutting OEE losses by almost half and saving approximately $120,000 for each failure.
Smarter capex decision–making
Better data means better investment decisions, especially when it comes to the allocation of sustaining capex costs—or avoiding equipment failure by making the right, risk-informed capex decisions. Most chemical companies struggle to set the right level of sustaining capex, as they find it difficult to allocate funds across multiple plants and disparate asset types.
This problem is ultimately about ensuring that resources are used to their maximum potential—which is exactly the question that zero-based budgeting (ZBB) has successfully addressed, through internal disciplines that assess all spending in terms of return on investment. The same techniques apply to capex investments in assets, or “asset ZBB,” which combines available historical data with local expertise to assess the potential impact of either replacing or not replacing particular equipment. The new approach allows all equipment renewal projects to be compared using the same yardstick: spend efficiency.
In the words of a manager responsible for running one chemical manufacturer’s capital-planning process, “We used to have a formal process to capture and assess sustaining capex projects, but we had no clear way to rank-order projects and always ended up prioritizing those with immediate, visible impact. Now we are able to have a fact-based, data-driven discussion about risk and trade-offs, which has led us to spend less overall—and to manage what we do spend more wisely.”
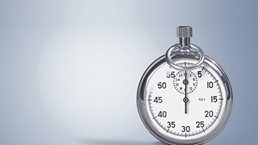
What high-reliability organizations get right
Root-cause problem solving
Better data also means better root-cause problem-solving. That helps companies to prevent the recurrence of failures, to improve their failure-modes-and-effects analysis (FMEA) processes, and to optimize preventative-maintenance plans. Together, those actions address the critical aspects of reliability performance, reducing both the impact of failures and the cost of preventing them.
At one chemicals plant, for example, failures in a critical piece of equipment caused operators to activate an emergency shutdown three times in as many months. These shutdowns were inconvenient enough—but when the site team attempted to restart the plant, they found that the abrupt shutdown of the unit led to an accumulation of solids in key vessels and pipes. Fixing that problem led to lengthy delays in start-up and significant losses in output.
To address the issue, the company applied a combination of traditional root-cause problem-solving and smart analytical techniques. Analysis of process data helped them understand how and why solids were accumulating under emergency shutdown conditions. The issue was fixed with a combination of enhanced monitoring and changes to preventative maintenance plans. But the data-driven insights also allowed the plant to revise its emergency shutdown procedures to stop the plant safely without causing the solids problem. That change reduced start-up time after any kind of emergency shutdown by 90 percent.
The potential for digital reliability extends far beyond predictive maintenance. And for chemicals companies, we believe that these other digital approaches are both easier to implement and offer greater value. The highly instrumented nature of most chemical production facilities means many companies already have a rich, and largely untapped, source of data to support digital reliability efforts. For those plants that still don’t, it’s time to “sensor up”: better data is the vital first step on the digital-reliability journey.