Many companies do their best to optimize production processes using established rules of thumb or incomplete data. But at the end of the month or reporting period, they often discover sizeable gaps between actual profits and what they had expected. In our experience, that is because they typically lack precise-enough measures to understand the small, real-time variations in process flows and manufacturing steps that cumulatively erode returns at facilities such as mines, steel mills, or other manufacturing plants. This information, moreover, is rarely shared quickly enough for managers to respond in the tight time frames required.
Stay current on your favorite topics
Our work across a number of industries suggests that companies can eliminate these profit-draining variations, as well as speed up reaction times by using advanced data analytics combined with upward cascades of data to manage performance. A metric we have termed profit per hour—which in an earlier article we described as a way to improve resource productivity—provides a much more exact view of fluctuations in the operating environment and a much better means of communicating the implications to top managers.
Extending the measurement frontier
By combining measures of value, cost, and volume over time, profit per hour is more potent than the sort of metrics commonly used in many industries. Using data captured from sensors, along with advanced-analytics tools, industrial companies can deploy self-learning models that simulate the expected value and cost of individual processes and even entire factories on a continuous basis. From this analysis, patterns emerge on where costs, heat levels, recovery levels, and other variables are deviating from predicted values. Operators can then fine-tune process procedures or adjust inputs so as to eliminate losses as much as possible during those periods in the day when profitability falls below optimum levels. The insights create a new information backbone, linking real-time performance at ground level to company profitability and allowing managers time to make the necessary trade-offs.
Would you like to learn more about our Operations Practice?
Until recently, companies lacked the usable data, advanced sensors, and processing capabilities to gauge the performance of operations with real-time precision. But increases in lower-cost sensors, wireless connectivity, cloud data storage, and computing power have changed the equation, as has the development of smarter analytics tools that analyze continuous process flows and complement advanced-process-control systems such as those found in refining, the petrochemical industry, or major production steps of the steel industry. Moreover, as more efficient and effective analytics emerge, there is greater scope to widen profit-per-hour analysis beyond just a few of the most critical processes. Meanwhile, further reduction in the cost of storing vast quantities of data allows finer-tuned performance management to reach across entire plants and even across companies.
Reaping the benefits
Two examples demonstrate how profit per hour can result in significant performance gains.
Process-level improvements at a chemical plant. The manufacturer had previously invested substantially in automation and advanced process control to increase throughput of a product line. Managers, however, knew that the external weather was affecting the efficiency of the process and the performance of the plant: the problem was they didn’t know to what extent. Technicians therefore identified a list of ambient and internal conditions that tended to vary in summer, such as wind direction, relative humidity, and temperature, among others. Armed with the necessary data, they built an advanced neural analytics model that was able to simulate profit per hour for the line under ideal, seasonally adjusted conditions—enabling management to note disturbances and take remedial action. The model further allowed the team to identify precisely the lost output and margin effect resulting from variations in each factor, including and in addition to the weather parameters. The team then focused on the top five that could be controlled by process adjustments or targeted investments. The company ultimately discovered that upgrading one piece of equipment could yield nearly €500,000 in value annually, in an investment that paid for itself within 12 months. The model also indicated how speedy reaction to operating deviations boosted profit per hour, a message communicated in additional training sessions for the frontline operators charged with monitoring dashboards and adjusting processes in real time. The newly defined parameters and rules were thereafter included in the process-control systems with the goal of increasing profits per hour by up to 2 percent.
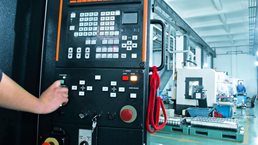
Buried treasure: Advanced analytics in process industries
Facility-wide gains at a steel mill. A steelmaker’s most important site seemed to be operating in the dark. Capital upgrades only intermittently resulted in higher returns. Operating decisions were often based on historical wisdom and personal experience, with little in the way of facts to demonstrate their potential financial impact. Meanwhile, data gathering was substandard, and manufacturing units within the plant often used different top-level key performance indicators (KPIs), preventing an integrated view of performance across the whole plant.
Senior management decided to remedy the situation with a radically different, multistep approach. At the core was a new KPI, which cascaded to the entire executive suite and linked operations performance to a single plantwide daily profit standard, grounded in profit-per-hour analytics. The goal was to give plant-level managers and frontline operators greater visibility into production variability, as well as to offer financial executives a surer sense of the facility’s performance. During the first phase, the mix of operational metrics was aligned with the new profit measure. In phase two, technicians tested the metric for insights into operating performance across the site’s hot rolling mills, steel-making plant, ironmaking plant, sintering plant, auxiliary power generators, and other units. In a third phase that involved new investments in IT, the company installed dashboard monitors (exhibit) that displayed the metrics both on the plant floor and in senior-management offices. A centralized data-storage system and standardized data analytics form the IT backbone.
The unified metric has allowed full tracking of costs. With additional training of frontline employees and managers alike, it has driven kaizen1 -like problem solving on a real-time basis. Variations in efficiency, previously likely to continue for days, are now eliminated within hours on average thanks to new ways of working across the facility. Costs have fallen by 8 percent in the two years since the new profit standard was adopted, and, coupled with other improvement initiatives, it has resulted in close to an $80 million cumulative increase in earnings. Additional gains are expected as better data analytics open pathways to new process improvements and work flows.
Exploring new horizons
With rapid adoption of process sensors and greater capture of data, artificial intelligence (AI) is likely to figure prominently in the next wave of gains. Analytics models will “learn” from process variations and make adjustments automatically. Google’s DeepMind AI is already doing this to reduce energy used for cooling its data centers by up to 40 percent. Models learn from historical data such as temperature, power consumption, and the functioning of cooling systems. They use that information to understand variations in data-center operating conditions and “judge” how best to run cooling systems with minimum power use. In future AI systems such as these, profit per hour could become the benchmark for optimizing operations.
While still in its early days, we’re seeing instances where profit per hour can be applied across multiple company manufacturing sites and even more broadly to supply-chain networks and decisions about how to serve customers. A more accurate, real-time view can help companies understand—among a growing list of possibilities—how to optimize the supply routes to a given finished product, how to most profitably serve customers when several production sites exist, how many products to manufacture from a single production site, and the best combination of make-versus-buy options. Such end-to-end systems could provide companies with unparalleled “postmortem” analysis of where value is leaking across their operations, as well as new ways to simulate the forward impact of strategic decisions.
With the growing capture of unstructured data on human interactions from video and social media, profit-per-hour metrics could soon be applied in nonindustrial settings, such as retail operations. As the quality of IT and analytic skills improves across sectors, and as managers learn to accelerate frontline adoption, productivity levels are likely to increase in a wide range of economic activities.