Voice data and analytics are a potential source of value beyond companies’ contact centers. This episode of McKinsey Talks Operations—the second in a series of two—explores how to apply them to new opportunities for competitive advantage and how to get the technology selection right.
Host Daphne Luchtenberg is joined by Paul Humphrey, CEO and founder of Call Journey, a global thought leader in conversation intelligence and speech analytics, and Eric Buesing, a McKinsey partner and a leader of McKinsey’s customer care offering. Their conversation has been edited for clarity.
Daphne Luchtenberg: Your company’s future success demands agile, flexible, and resilient operations. I’m your host Daphne Luchtenberg, and you’re listening to McKinsey Talks Operations, a podcast where the world’s C-suite leaders and McKinsey experts cut through the noise and uncover how to create a new operational reality.
I’m joined today by Paul Humphrey, CEO and founder of Call Journey, a global thought leader in conversation intelligence and speech analytics who has more than 30 years of experience across multiple industries, and Eric Buesing, a partner at McKinsey and a leader of our customer care service line. We’re continuing our previous discussion about technologies that help natural-language processing [NLP] and natural-language understanding [NLU]. In our last episode, we discussed the technology and its potential in contact centers.
Today I’d like to explore more about its implementation and how to actually capture the value available. Let’s go beyond the contact center application and take a look at the wider business and where voice data analytics can add value. Paul, it strikes me that technology, of course, is fundamental to all of this. How should people be thinking about their technology stack to actually ensure that they’ve got the right tools in place?
Paul Humphrey: It’s really a mindset, and that mindset is thinking about the interactions that are being had every day, every moment with customers. And we’ve shifted from the terminology of “call center.” If we go back to about ten years ago, it was known as a call center because most of those interactions were voice, and you would call into the call center. That’s changed now to “contact center,” because folks want to liaise with their supplier on all different channels, on all different facets, moving with how they move. So they may call, they may have a digital interaction, maybe it’s an SMS [short message service], maybe it’s a chat. Our personal experience: just today, I’m moving house, so I had to contact my different providers. I was online doing a chat, and then I shifted to voice and back to chat again.
Subscribe to the McKinsey Talks Operations podcast
Organizations first have to have technology to manage all those different interaction points; and second, they also need to be smart enough and intelligent enough to then manage that customer journey, regardless of where and how that person interacts with that organization. Again, back to my example here today of liaising with a telecommunications provider, I was online, then I was offline, but my whole journey is still, in my mind, moving house. The technology and the data insights are there to help deliver that full omnichannel journey and understand where the customers are in that journey. And we know organizations are smart enough to even get to things like episodic NPS [Net Promoter Score], so understanding where different cohorts are, different journeys, and different episodes of that journey deliver a different NPS, etcetera.
So it’s really having the right technology to manage multiple omnichannel interactions but then also the right approach and what we call, I guess, augmentation and harmonization of data. That harmonization is super important to getting better insights into the customers because you may need different data points. Eric gave an example earlier about organizations [in the first episode on this topic], for example, trying to drive improved revenue performance. They’ll go and find their top-performing agents and work out how they’re interacting with a customer, learn from that, replicate it, and then deliver that into the organization. Now, the big risk there is that if they’re not taking all sorts of different data points into consideration, then you could be delivering a bad experience for the customer—creating the wrong type of culture, for example.
In fact, we did some work recently where an organization had exactly the same challenge that Eric mentioned before. They were in the insurance sector, and the question I asked them was, “Do you have a separate policy administration system which determines the product and the service that that customer has?” They said, “Yes, we do.” And I said, “Do you track things like lapse?” which is, in an insurance world, when customers chose an insurance product but left very quickly after initially coming online and selecting a new product or service. So how quickly do people lapse? How often do they lapse? And in what period are they lapsing after that sales event? Was that sales event done by an in-house contact center, or was it done by an outsourced partner? All of these things start to determine whether there was a good interaction. You might have a high-performing salesperson who’s delivering lots of revenue, closing lots of sales, but if all of those sales are lapsing, for example, three to six months afterward, then that doesn’t necessarily mean it’s a great experience, doesn’t necessarily mean it’s what you really want to be delivering. So, again, augmenting all sorts of data, which would be the interaction data plus policy and administration data and journey data, now gives you a much better feel for what an ideal customer experience would look like at that point of acquisition and at that point of customer journey.
Would you like to learn more about McKinsey’s Operations Practice?
Daphne Luchtenberg: Yes, very clear. Thanks, Paul. Eric, it strikes me that that also means teams have to work together in a completely different way. So there are operating-model implications here, too, would you say?
Eric Buesing: I like the term that Paul used: harmonization of data. And I think organizations are looking all the time at how they do that. One thing they’re recognizing is that the contact center doesn’t operate in an isolated way. In fact, most organizations are actually a bit siloed in their thinking. But that’s not how customers transact; customers don’t think, “Now I’m interacting with your app; that’s different from speaking with a voice assistant bot, versus different from speaking with somebody in collections, which is different from speaking with somebody in servicing.” They think, “I have a problem, and I’m trying to get it resolved.” That’s requiring organizations to, in some ways, break down those silos and think about a customer interaction, not a transaction in one channel. That’s the operating-model change, I think.
Customers think, ‘I have a problem, and I’m trying to get it resolved.’ That’s requiring organizations to break down their contact center silos and think about a customer interaction, not a transaction in one channel.
Daphne Luchtenberg: Fantastic. It feels like we’re only just scratching the surface, Eric and Paul. You’ve shared so many fascinating insights, but let’s get down to some practical steps for our audience. What advice would you give to leaders who are on their journey, perhaps quite well equipped with NLP and just starting to experiment with NLU. How should they be thinking about bringing machine learning into it, to really make themselves into a mature organization? What advice would you have for them?
Paul Humphrey: My first advice, which is the first and foremost advice I almost give every one of our clients, is don’t boil the ocean—[the mindset] that there’s always a need to go and do a whole heap of things first. The great thing with interaction analytics and insights is that there are so many different fish you can catch. There are so many different use cases, as Eric was saying before.
I’d also say, “Be very clear about specific use cases.” Have clear outcomes, measures of success that you’re looking to achieve, and commit to systematic processes to underpin that activity. Then also, you really need to ensure that you have effective commitment of resources to give the opportunity life, get the project life, etcetera, and always be remembering that you can’t expect what you can’t inspect. So AI advanced analytics—it’s not just a shiny, sexy tool, it should really become part of your DNA.
AI advanced analytics—it’s not just a shiny, sexy tool, it should really become part of your DNA.
Then I always think [about] five pillars for best outcomes. Pillar one: we talked about this before, and if you could see me now, I’m holding my hands together in a praying situation, and I’m saying, “Please, please, please, homogenize and harmonize your data.” Both human intelligence, artificial intelligence, and multiple data sources. And don’t forget, your biggest channel is still likely to be voice. But make sure you’re adding your voice data and other interaction data to that central data repository so that you’ve got it for the true holistic view you absolutely should need. That’s point one.
Point two is don’t fear what you might find. Embrace the opportunity to get more clear and concise insights.
Point three, lean on experts and thought leaders. Organizations don’t know all the solutions, but if it’s done properly and efficiently and effectively, you will reap huge business performance benefits from interaction intelligence.
Point four, make it an ongoing part of your organizational DNA to interrogate and understand both your CX [customer experience] and EX [employee experience] insights. Again, of course, you need to do that through the right data sources and the right structure. So create closed-loop, ongoing operating rhythms, constantly checking and rechecking, constantly testing, constantly measuring against the appropriate benchmarks.
And last, utilize thought leaders and experts to give you a deep-dive-based, stake-in-the-ground assessment of your current state. Don’t leap into a reasonable transformational change if you haven’t done a check as to where we sit right now. External benchmarking and a deep dive are always a great way to start to get a true assessment of where you sit, how you are against world’s best practice, against different sorts of benchmarking.
Daphne Luchtenberg: That’s a really clear set of step-by-step pointers there, Paul. Thanks so much. Eric, maybe you would take more of a philosophical approach. What is the mindset that leaders should be adopting as they try to get better in this space?
Eric Buesing: I have a client that uses the phrase “Don’t let perfect be the enemy of good.” I really like that, and I think it applies in this context. Data is never perfect. It’s never going to be as connected as it could be. The architecture or the environment is never going to be fully ready. There are always capacity constraints. But great organizations are asking questions about what would it take to get started sooner versus what needs to be in place before we can begin. So the first piece of advice is to start small. Pick one cue or one area to test these capabilities and actually begin building the muscle, because you’ll learn a lot about how your organization operates as you ask a lot of questions. What are the risks? What are the failure points? So when you are ready to scale, if you ever reach that point where you’re truly ready, you’ll scale faster.
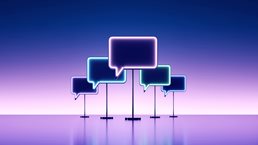
The hidden value of voice conversations: Part 1, Trends and technologies
Maybe the second philosophical question to answer is, do we really understand the problem, or are we looking to apply a solution? I think the former is the right approach. Advanced analytics, AI, even NLP and NLU—these sometimes are buzzwords that get thrown around, and I think a lot of people don’t fully understand what they are, but they’re buying on a promise. You’ll often end up getting disappointed. So spend the time up front: do a diagnostic, understand what your customers care about how they transact, and simultaneously do a diagnostic of your contact centers. That’s, as Paul mentioned, across voice, email, text, even videoconferencing; that’s the next frontier of understanding interactions. Bring those together to see where do we have a problem—and maybe, by the way, where do we not need something as sophisticated as NLP and NLU—but where could technology like this really help us tackle, as I’ve mentioned before, an unwieldy amount of data.
The third and final piece is, yes, start small, but know that you’re building a foundation for much more than the contact center. These capabilities are being perfected there, but they’re ripe for transplanting to other parts of your organization, like marketing and sales of products. NLU in the contact center can help you answer questions like, are we reaching the right customers in the right channels? Where did this customer actually come here from? Are there any warning indicators about product features that we need to know about? Or [NLU can reveal that] we didn’t realize that there’s a particular feature that’s unique and important to customers, so we should be highlighting that more. And even—and this is a unique one we learned in the telco industry—competitive intelligence: What are customers saying they’re being offered from other organizations? That’s also unique insight. So this capability might be being built specifically in contact centers, but it has broad applicability across the rest of your organization, and I think that’s something to consider as well.
Daphne Luchtenberg: Eric, thank you. That’s such a powerful thought, that this is at the forefront, it’s already adding value immediately at the core and the heart of the organization, but there’s so much more value that can be reaped. Paul, a question for you: We’ve been talking about the application of these technologies in this approach in the contact center environment, but I imagine there are many other applications in the longer term across the organization value chain, no?
Paul Humphrey: Yeah, absolutely, Daphne. In fact, I think one of the biggest trends that we’ve seen in the past, I’d say, 12 to 18 months is that smarter organizations are utilizing those things we were talking about earlier—NLP, NLU, machine learning, and solutions in the realms of speech analytics—for business performance and benefit outside of the contact center. So not just serving the contact center, which is where the best life, if you like, of speech analytics is often lived. But now using that data to serve businesses or business units outside the contact center is where we’re seeing the trend. The important thing to note, though, is that the contact center interactions are the source of the data and the deliverer of those insights. In fact, we’ve got a saying now that voice data has many interested parties.
We’re starting to see regular adoption of that interactive intelligence by marketing, for example, to drive deeper, more effective personalization [of the] customer journey experience through better real live data. We’re seeing areas like the revenue part of the business use interaction intelligence to understand share-of-wallet churn and the drivers of retention opportunities, how we improve conversion rates. For any organization, even a 3 to 5 percent improvement in conversion rates, through better revenue intelligence from those interactions, has mind-blowing impacts on revenue. We also—and I think Eric was touching on this earlier—we also see that CX shift from relying wholly on post-call surveys for customer intelligence to now having predictive NPS and customer prediction of next-best offer, for example, from every interaction, because you now can do AI at scale. Then we’re seeing areas like legal, risk, and governance turning to conversation intelligence to deliver better compliance, better risk and governance assessment—again, on every interaction. They don’t want to leave anything to chance, and they don’t want to just leave it to human intelligence.
We’re seeing areas like legal, risk, and governance turning to conversation intelligence to deliver better compliance. They don’t want to leave anything to chance, and they don’t want to just leave it to human intelligence.
Last, I think this is extremely interesting at an innovation level, particularly around our current global climate, around employee health and well-being and employee engagement, and Eric touched on this earlier. What we now know, for example, is that DEI [diversity, equity, and inclusion] is on page one of every Fortune 500 company’s annual report. And we know, for example, that thousands of CEOs have pledged commitment to true workplace foundations of equity, diversity, and inclusion through a group called CEO Action. We also know that almost every CEO or HR global leader is striving to create and enjoy the benefits of a psychologically safe workplace. I didn’t know this until recently, but the person who coined the phrase “psychologically safe culture” is Professor Amy Edmondson, and she’s a professor from Harvard Business School. Her studies show a huge correlation between psychological safety and high performance. When an organization is able to create that culture where it’s safe to express ideas, ask questions, admit mistakes, staff are retained longer, productivity is supercharged, and true self-empowerment happens.
Why am I mentioning this? With the increase now in the use of unified comms tools or UCaaS [unified communications as a service], such as Teams, Zoom, WebEx, BlueJeans, etcetera, we, collectively as a race of humans, if you like, are spending infinitely more time communicating with one another through those tools. In fact, if you look at just the US in one day alone, at organizations with plus-500 staff, 132 million hours of Teams conversations happen every day. That’s every day.
Now imagine a world where you could harness all of those rich conversations and deliver real-life experience insights to employees for their own self-empowerment—to help them understand their well-being, their mental health, how diverse and inclusive they are, whether they’ve got unconscious bias, how they best engage in work-from-home or hybrid settings. Also imagine a world where the employers understand the true lived experience of their employees and where they should actually put their investment of time for organizational transformation or cultural change or well-being—all of that anonymized and aggregated, of course, for the employee. And that’s now what we can do with advanced NLP, NLU, and machine learning combined with high-powered people science. Effectively, smart organizations need to realize, and realize quickly, that interactions are rich with insights for both CX and EX. Again, back to that same point I made earlier, ultimately, it’s about finding the right partner and thought leader to embrace that and realize those vast opportunities available in both of those areas.
Daphne Luchtenberg: Got it. I love that. Thank you so much, Eric. Thank you, Paul. I suspect if we had another hour and a half, we could really dive deeper. But I’m hoping that our listeners have been able to get a really good first picture of the opportunity and this exciting new world of NLP and NLU and really understanding what our customers want. Thanks so much to both of you.
Paul Humphrey: Thanks, Daphne.
Eric Buesing: Thank you, Daphne.
Daphne Luchtenberg: Thank you for your time today. You’ve been listening to McKinsey Talks Operations with me, Daphne Luchtenberg. If you like what you’ve heard, subscribe to our show on Apple Podcasts, Spotify, or wherever you listen. We’ll be back with a brand-new episode in a couple of weeks.