‘Humans in the loop’: It’s the angst-ameliorating mantra for the new age of generative AI (gen AI). But what does it really mean? In this episode of McKinsey Talks Talent, Stanford University professor Melissa Valentine joins McKinsey partners Bryan Hancock and Brooke Weddle, along with global editorial director Lucia Rahilly, to discuss human-centered artificial intelligence: what it is, how it improves performance, and how it can help shift skittish employees’ mindsets from “ugh” to “wow.”
This transcript has been edited for clarity and length.
What is human-centered AI?
Lucia Rahilly: AI, and particularly generative AI, has exploded into both the business and popular lexica in the past year. Melissa, talk to us about “human-centered AI.” What do we mean when we use that particular term?
Melissa Valentine: There are different aspects that refer to different paradigms of design. But the way to think about it is, if you’re really focused on augmenting human capabilities, that’s human-centered AI.
So generative AI; it’s just data. It’s just a language model. But it’s also all the social arrangements that have to happen around it for it to actually accomplish any of the things where we see the potential.
Lucia Rahilly: What does research tell us? Are folks really that afraid of AI in the workplace and the threat that it’s likely (or unlikely) to pose to their jobs?
Melissa Valentine: It’s easy for people to understand the potential of generative AI, where everyone having a “pair AI” helping them with their jobs doesn’t seem super threatening. I think it’s a little harder to connect what we’re seeing—where making a slide deck goes a lot faster or where your emails get auto-completed—to this sense of existential job loss that people are worried about. That’s more where the rhetoric about fear is happening—fear in a more macroeconomic sense that there won’t be jobs in the future. Local adoption seems to be less threatening to people.
In the ’90s, there were a lot of labor economists and occupational researchers studying digital technologies coming online. The trend they were documenting was that some occupations were being reskilled—they became augmented—and some were de-skilled. And some new occupations came online. As we see, the predictions of job loss in the ’90s haven’t played out the way the more cataclysmic predictions foretold. However, there have been so many changes in occupations between the ’90s and now. It is profound what has happened.
There is going to be a lot of change. This is the discussion right now: will this be a continual change? Is it going to be like the past, where there are profound changes to what occupations look like but there’s not societal job loss across the board?
Moving from ‘ugh’ to ‘wow’
Lucia Rahilly: What are some ways of addressing skepticism and overcoming early resistance to the adoption of generative AI in the workplace?
Melissa Valentine: I did a study at a tech company in San Francisco called Stitch Fix, and I picked one of its data science teams to study. They were developing a new algorithm and trying to help their workforce adopt it. By the end of the study, they had gotten broad adoption across a huge department and had really reskilled their workers.
Among the key actions, the team looked at what the workforce was doing, with their data science tool kit in mind, and asked themselves, “How can we help our teams accomplish their goals better? How can we use some of these data science capabilities to really augment people’s analyses?” All their framing was in terms of new capabilities: how can we help people do all of this better? I think that’s key.
The second piece that seemed powerful was that they had a really talented user interface [UI] lead developer. The users were Stitch Fix fashion buyers, so they would buy inventory. The lead developer built a UI showing the purchasing team pictures of everything the company was stocking into inventory, along with pivot tables to guide decision making. And the bubbles picturing the clothes would get bigger depending on the volume of items the buying team was picking.
The buyers loved this. It really unlocked for them what the algorithm was doing. It made it so much easier for them to explore what they had input. It let them explore what the algorithm was recommending. It let them play around with different ways to make the decision. So the whole thing was really set up around giving them new capabilities. The UI was powerful in helping them adopt the technology.
Subscribe to the McKinsey Talks Talent podcast
Bryan Hancock: Conversations with my clients around generative AI sometimes start with, “Well, what’s the automation potential? What’s the cost savings?” But by the end of the conversation, it gets to, “Wait a minute. What’s the revenue potential here? Because I think there’s a real opportunity for us to sell better, be better in tune with markets, better pick up on trends, and better synthesize information across multiple sources to ultimately serve our customers better and then grow revenue.”
Then the energy in the room shifts from this “ugh” conversation around, “Yeah, those are the roles, those are tasks, those are the pieces,” to, “Wow, there’s huge potential here for untapped market opportunity if we could only go after it.” Is that similar, where capabilities lead to revenue growth and excitement?
Melissa Valentine: Yes, exactly. That’s a great way to put it.
Rethinking roles with AI as copilot
Lucia Rahilly: Melissa, is adoption of gen AI affected by employees’ self-identity? I’m thinking particularly here of creative fields where folks may view algorithms as anathema or as a poor substitute for imagination or ingenuity. Or even in the fashion example, just for years of experience and acumen that’s hard won.
Melissa Valentine: Yes, I think the importance of professional identity is really underexplored, largely because so much of this is new and there hasn’t been time for identities to evolve.
It’s reminding me of a great study at NYU, where they were looking at how NASA teams were learning how to use open-innovation platforms, where you post a problem online and then someone external can solve it. That’s very threatening to scientists who are used to solving problems themselves. I think it was a five-year period where the NASA scientists’ identities changed from being the ones who solved problems to the ones who sought solutions.
When I was at Stitch Fix, I saw something similar. My study was over only about an 18-month period, so I wouldn’t say that the identities had fully evolved by the time the study ended. But I did see that identity conflict you’re talking about—because people don’t go into fashion because they want to do optimization models. They go into fashion because they love fashion. So the best fashion buyers were also fashionable. And they had really great relationships with vendors and a pulse on what was happening in the industry.
But these are the people who are supposed to be in this dashboard looking at risk and uncertainty, and asking, “How am I optimizing? And what are the trade-offs that I’m making?” It was really a shift for them to have to integrate what it meant to be a great fashion buyer with what it meant to use data in strategic ways and to support decision making and strategic trade-offs.
Bryan Hancock: How do you think about this idea of the copilot intersecting with identity?
Melissa Valentine: If a copilot is doing the tasks you didn’t want to do in the first place, like calculating all your financial metrics, then you’re just pleased. But if you’re a fashion person and suddenly your copilot is designing clothes for you, what does that mean? What does that do to your identity?
Bryan Hancock: It’s fascinating. It reminds me of an article I read about a university English professor who was encouraging students to use generative AI tools because “you need to write better than that.” This is a baseline. This is the start. This isn’t replacing. You’re providing the human insight, understanding. That’s how you build on top. Some of the biggest areas of potential for gen AI are in marketing, in sales, in communications.
Melissa Valentine: Similarly, I wonder which of our products are good enough, when they’re just that first draft of generative AI. And which products are you really going to innovate on and enhance with a lot of prototyping, and interactivity, and human insight, and creativity?
Brooke Weddle: Also, how can you bring this more discerning lens of bias, and interrogating the impact of that bias—having that critical thinking layer that we need to hone as a skill to get maximum value out of generative AI?
Melissa Valentine: I totally agree. The interesting thing that will be added to that is figuring out how to evaluate the impact. Going back to my Stitch Fix study, the skills the fashion buyers had to learn included how to be in the loop with the algorithm to measure the impact of their intuition.
It wasn’t generative AI in that case; the algorithm would make a recommendation and display the metrics associated with its recommendation. The buyers would go in and overwrite a recommendation. And then the algorithm would automatically tell the user, “OK, you’re imposing that intuition here. It’s going to cost you (I’m just making up a number here) $1 of revenue.” And then the buyers could say, “OK, that’s worth it.” So they were evaluating how intervening in the algorithm would cost $1.
Learning how to measure the impact of human intervention into whatever the algorithm is putting out is a skill that’s going to be needed, even for generative AI.
Gen AI and organizational culture
Brooke Weddle: From a broader organizational culture standpoint, if I have my copilot and it’s advising on markdowns, does that make it less likely for me to interact with the pricing department or with finance? Are there silos we’re creating by enabling people with this copilot? And we all know that organizations are based on a series of values. Trust is important. Relationships are important to making a company run well. Any thoughts on that?
Melissa Valentine: Yes, absolutely. Let’s just think for a second about the way some companies have set up algorithms as ratings systems, as this interactive algorithmic data product for workers. A good example is Tripadvisor, where all sorts of ratings and data come together. Hotels, when they’re trying to react to a Tripadvisor score, don’t know where all that data came from that has been collected by these algorithms. So they end up having this opaque algorithm that they’re trying to work with to learn how to become better.
I’m using the hotel example because people can imagine what it’s like for a hotel to get a list from a professional rater of what to change, versus dealing with Tripadvisor. But that same dynamic is also happening with workers, especially in online labor markets. They’re getting one score that rates how they’re doing. And it’s been pretty hard for people to learn how to get better. What does it mean when you don’t have a professional manager—when you just have this algorithm to tell you what it means to get better?
Bryan Hancock: What that makes me think of, Melissa, is the potential for large language models to help people sift through all the unstructured data and comments, to come back with the list of what you need to do.
One of the use cases managers are interested in is employee complaints. Somebody responsible for a region might have thousands of employees. Is a complaint a one-off or is it a trend? And is there something I can gather from data to quickly help me understand that? Is there a promise that future technology will be able to go through the data and say, “Hey, that one-piece looks like an outlier. We don’t see it anywhere on Glassdoor, nor do we see it in any of the surveys, or elsewhere.” Or it could say, “Actually, thematically here are the four things that have come up from our employee surveys, and what we see online, what we see on Reddit.”
I wonder if some of these technologies can provide a lot more of the color behind what’s happening and recommendations about what to do?
Melissa Valentine: What you’re calling out—and I think this is totally right—is to imagine with all the information, all the sentiment that is in play, what you can learn.
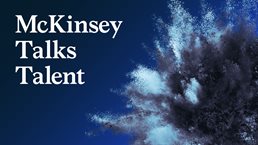
McKinsey Talks Talent Podcast
Lucia Rahilly: Are folks afraid that gen AI might tell HR who to hire—and maybe more forebodingly, who to fire, on the basis of these kinds of complaints that surface through the algorithm?
Melissa Valentine: A lot more surveillance, a lot more automated hiring and firing—clearly, you’re going to see people resist that. It’s not an empathetic way to configure things. But in the same organization, some occupations have a lot of autonomy and are likely to become augmented. And you might also have lower-status occupations that are subject to a lot more surveillance, a lot more algorithmic management, a lot more of the unpleasant aspects of algorithmic control.
A well-known example from the newspaper is a driver who was fired by a bot and had no recourse. He couldn’t even talk to HR to figure out what had happened. I think that’s where we’re seeing a lot of resistance.
Brooke Weddle: While one way of thinking about generative AI is with this frame of control, another is using these approaches to try to unleash employees in new ways, in productive ways, to get them from burnout to thriving.
One of the organizations I’m working with is trying to build what they could call a new managerial operating model, taking all their pulse data and marrying that with management science around the practices that help teams drive productive outcomes. To be clear, you’d need to think through ways to cultivate manager buy-in, employee buy-in, and make this two-way. I think that would absolutely be part of it. But then you could imagine a system that’s nudging employees to be the best version of themselves.
That’s the big idea. And I think it’s quite exciting. But clearly there are a lot of minefields to work through, making this not a control state but rather an enabling state.
The future of organizational design
Bryan Hancock: One of the things I’m excited about is the idea of applying generative AI to design managerial roles. Let’s look at all the things a manager hates to do and use AI to take away some of these administrative tasks. I’d love to hear your thoughts on using a design lens to take away work that managers do that drives them nuts.
Melissa Valentine: Design thinking using generative AI to really examine middle managers—I think that’s really smart. A lot of the focus at present is on frontline decision making, doctors, or the people I was talking about: fashion buyers, merchandisers, and the pricing algorithm. But something I’m super fascinated by is that managers are regularly making organizational-design decisions. And organizational design is not a science; it’s an art. So if managers were more data-driven or if they had more empirical insight into the organizational-design decisions that they made, I think it would unlock some really exciting stuff. I would love to watch that.
Lucia Rahilly: Melissa, on the question of organizational design, what’s an example of the way AI or gen AI might alter the way companies staff project work? And here I’m thinking of the experiments you’ve been running on flash teams and flash organizations in particular.
Melissa Valentine: With flash teams, companies would be doing a task, and the bot would come in and make a recommendation of a new thing they should try, like more centralized decision making, more turn-taking in their decision making, or something like that: small interventions.
Over time, we were able to help the teams experiment with those recommendations and show that the ones getting recommendations specifically on their organizational design got better. With staffing and deploying flash teams on a project, a software platform could anticipate the need for different roles, and then reach out to a labor market, in this case, and automatically assemble the team. It could pull the team together and then structure the team’s work over time: for example, show them who should be passing off to whom and when, where to upload the work, who’s the manager in this case, things like that.
Bryan Hancock: Is that largely leveraging freelance marketplaces? Or are there other ways you’re tapping into the labor market to pull in those flash teams?
Melissa Valentine: We’ve done research with companies doing internal deployments of flash teams. In the past it was easier to do that with labor markets because they are online, and they have such smart platforms. But you can still do it with a large company and an internal workforce.
Data privacy and other risks
Lucia Rahilly: Everything we’re talking about hinges on data, and the proliferation of data and the acceleration of the proliferation of data. How do you assess data privacy risks in the HR context?
Brooke Weddle: That is coming up in 100 percent of my conversations on generative AI data, not just from the HR angle, which of course is extremely important. But even in the McKinsey context, where you have people serving competitors. How do you segment data thoughtfully?
How do you tag data in one way so certain data is fungible, portable, and other data is not? Who makes that call? How do you do that globally across multiple countries? It’s a really complex challenge that a lot of people are thinking about already, but one that is pretty fundamental to getting the generative AI part of the equation and the impact from that.
Melissa Valentine: Absolutely. Even just as people are typing in their prompt questions. That’s still telling something about the company.
Brooke Weddle: Yes, 100 percent.
Bryan Hancock: It makes me think of the risks more broadly. We’ve mentioned this on a prior podcast, but one of the things I worry about is the risk that we become less interesting. We don’t have the time to really push the boundaries for what makes exceptional answers and exceptional outcomes. So that’s my counterintuitive risk.
Brooke Weddle: The other that’s come up a lot in conversations I’ve had is if you go to a model where you are assisted by an algorithm, by a copilot, the concern is around experience accumulation, the act of failing, and the things you learn from that. There’s value in that in terms of professional development. If I’m assisted in these ways, if I always have this running head start, what am I giving up? Especially if I’m a junior colleague, what am I not experiencing that could end up with me having less insight down the road?
Melissa Valentine: Yes, the deprecation of expertise. The more we’re aided, the more we’re not going through all the repetitions where we develop expertise all the time.
Lucia Rahilly: Exactly. One last question: does using a human-centered lens change the way we assess the success of AI tools in an organization? What’s the protocol there?
Melissa Valentine: Can I tell one last story that empathizes both with the workers and with the developers? We were looking at algorithmic rating of flash teams. We really wanted to make sure all this algorithmic management was very worker-centered, human-centered.
We were playing around with the idea of adding a variable to an algorithm that was some weighting factor for human-centeredness. There was a quarter deadline for the company we were collaborating with, and suddenly we needed business results tomorrow. So we said, “OK, just this once we’re going to do it without this variable in.”
And then, bam. That’s how it happens. The pressure of needing to have business results fast—those are the moments of trade-offs. You need to have the space to be able to do something human-centered, because it takes longer. It’s harder.
Brooke Weddle: Couldn’t agree more.
Bryan Hancock: To me it calls to mind the classic short-term versus long-term trade-offs. There may be a real short-term profit trade-off: “Hey, the algorithm has some really cool things it can do right now. Let’s get it out there.”
But in the longer term, having the human-centered approach is going to uncover even more opportunities for employees and for customers, help with the sustainability of organizations, and help unlock new markets, new sets of opportunities, new sets of insights.
Brooke Weddle: Yes, I think the classic performance and health scorecard around AI will be really important, so that in those moments where push comes to shove and everyone is tempted to go back to performance, you’ve got the health side of the equation right there staring back at you. I think that’s going to be really critical. And if that means moving a little bit more slowly, then I think the trade-off is clear.
Melissa Valentine: Exactly.