Researchers agree that unleashing deep-learning models on the vast amounts of data available today has progressed artificial-intelligence capabilities to once unattainable levels. However, deep learning isn’t the ideal technique for every application in which AI could provide significant performance gains over what humans could achieve alone. Earlier this year at the AI Frontiers conference in Santa Clara, California, we sat down with AI experts from some of the world’s leading technology-first organizations to learn about other techniques researchers are exploring to expand the applications of AI. An edited version of their remarks follows.
This video is one in a five-part Ask the AI Experts series that answers top-of-mind questions about the technology:
- What’s driving today’s progress in AI?
- What are the applications of AI?
- Should we be afraid of AI?
- What advice would you give to executives about AI?
Interview transcript
Mohak Shah, lead expert, data science, Bosch Research and Technology Center, North America: In terms of technology, I think acceleration is going to come when we put all the pieces together. We already have things like deep learning that are making advances. Now what we are going to see is machines that can develop capabilities or understanding without being provided this information from the outside. I think that in the classical machine-learning or AI world we would like to go from the supervised-learning world, where we are telling machines what to learn, to a world where the machines can infer things on their own.
Adam Coates, director, Baidu Research Silicon Valley AI Lab: One of the big problems with deep learning the way we use it today is that we need huge amounts of annotated data. For example, for speech recognition—which I think is exciting because it’s getting so good—one of the challenges is that we don’t just need the audio for the speech, we need a human to give us a transcription. That can be very expensive.
So if you want to build a new application, you have to first think about where you are going to get all that annotated data for the thing you want to predict. One of the things I think is really exciting is that unsupervised learning is starting to show some very interesting results. Unsupervised learning is an algorithm, or a class of algorithms, that can learn to either make predictions or learn about your data set without being told what to look for. We know that humans do this all over the place. I have a young son, and it’s clear that I didn’t have to tell him that this was a coffee cup a thousand times. He just knows that it’s a cup after a couple of examples. The reason that humans can do this is that we’re learning from all the other things we’re seeing and hearing, and somehow fusing that into our knowledge, so that when you say, “That’s a cup,” I quickly learn that concept.
We haven’t quite made the last connection to figure out how to make all of our supervised systems, all of our predictions, much better by using these unsupervised technologies. I think if that happens, that’ll mean we can harness new kinds of data, and we can make much better predictions than we’ve done in the past.
Li Deng, chief AI officer, Citadel: The talk that I gave today about what I call “the dialogue systems”—some people call them “the bots,” but it’s really the same concept: how to have an agent, an intelligent agent, who is able to converse with a human. That is the kind of problem that not just big data can solve and not just the deep depth of the neural network can solve. It requires some very intelligent way of interaction. That’s a different paradigm, it’s called the reinforcement-learning paradigm.
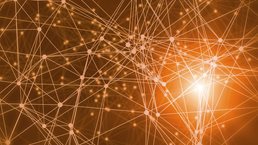
Ask the AI experts: What advice would you give to executives about AI?
Gary Bradski, chief technology officer, Arraiy: The big thing in deep learning is deep reinforcement learning. These are the techniques that have been beating people at game play. Reinforcement learning is where, in the end, you want to achieve a goal, such as putting something in a slot or a rat getting food.
If you’re doing a maze, you went left-right-left-right-left-right, found food. That’s when you get your signal, “Hey, I got food.” You have to remember and propagate that back in time so that you know, “Oh, I should take a left here and a right here.” So that’s what reinforcement learning is—it’s learning from these end rewards, and being able to assign that signal, that learning signal, back through that trace in time.