CEOs often live by the numbers—profit, earnings before interest and taxes, shareholder returns. These data often serve as hard evidence of CEO success or failure, but they’re certainly not the only measures. Among the softer, but equally important, success factors: making sound decisions that not only lead to the creation of value but also “do no harm.”
Stay current on your favorite topics
While artificial intelligence (AI) is quickly becoming a new tool in the CEO tool belt to drive revenues and profitability, it has also become clear that deploying AI requires careful management to prevent unintentional but significant damage, not only to brand reputation but, more important, to workers, individuals, and society as a whole.
Legions of businesses, governments, and nonprofits are starting to cash in on the value AI can deliver. Between 2017 and 2018, McKinsey research found the percentage of companies embedding at least one AI capability in their business processes more than doubled, with nearly all companies using AI reporting achieving some level of value.
Not surprisingly, though, as AI supercharges business and society, CEOs are under the spotlight to ensure their company’s responsible use of AI systems beyond complying with the spirit and letter of applicable laws. Ethical debates are well underway about what’s “right” and “wrong” when it comes to high-stakes AI applications such as autonomous weapons and surveillance systems. And there’s an outpouring of concern and skepticism regarding how we can imbue AI systems with human ethical judgment, when moral values frequently vary by culture and can be difficult to code in software.
While these big moral questions touch a select number of organizations, nearly all companies must grapple with another stratum of ethical considerations, because even seemingly innocuous uses of AI can have grave implications. Numerous instances of AI bias, discrimination, and privacy violations have already littered the news, leaving leaders rightly concerned about how to ensure that nothing bad happens as they deploy their AI systems.
The best solution is almost certainly not to avoid the use of AI altogether—the value at stake can be too significant, and there are advantages to being early to the AI game. Organizations can instead ensure the responsible building and application of AI by taking care to confirm that AI outputs are fair, that new levels of personalization do not translate into discrimination, that data acquisition and use do not occur at the expense of consumer privacy, and that their organizations balance system performance with transparency into how AI systems make their predictions.
It may seem logical to delegate these concerns to data-science leaders and teams, since they are the experts when it comes to understanding how AI works. However, we are finding through our work that the CEO’s role is vital to the consistent delivery of responsible AI systems and that the CEO needs to have at least a strong working knowledge of AI development to ensure he or she is asking the right questions to prevent potential ethical issues. In this article, we’ll provide this knowledge and a pragmatic approach for CEOs to ensure their teams are building AI that the organization can be proud of.
Sharpening and unpacking company values
In today’s business environment, where organizations often have a lot of moving parts, distributed decision making, and workers who are empowered to innovate, company values serve as an important guide for employees—whether it is a marketing manager determining what ad campaign to run or a data scientist identifying where to use AI and how to build it. However, translating these values into practice when developing and using AI is not as straightforward as one might think. Short, high-level value statements do not always provide crystal-clear guidance in a world where “right” and “wrong” can be ambiguous and the line between innovative and offensive is thin. CEOs can provide critical guidance here in three key areas (Exhibit 1).
1. Clarify how values translate into the selection of AI applications.
Leaders must sharpen and unpack high-level value statements, using examples that show how each value translates into the real-world choices that analytics teams make on which processes (and decisions) should be candidates for automation.
We have seen some great examples of companies using “mind maps” to turn corporate values into concrete guidance, both in terms of when to use AI and how. One European financial-services organization systematically mapped its corporate values to AI reputational risks. The exercise led it to decide that, while AI could be used to recommend new services to clients, it should always include a “human in the loop” when advising the financially vulnerable or recently bereaved.
In addition to leading mapping exercises, CEOs should ask both business and analytics leaders to explain how they interpret values in their work and how they use these values to make better decisions. This can jump-start conversations that identify and clear up any fuzzy areas.
Would you like to learn more about McKinsey Analytics?
2. Provide guidance on definitions and metrics used to evaluate AI for bias and fairness.
Value statements can also fall short when it comes to how concepts such as bias and fairness should be defined and measured in the context of assessing AI solutions. For example, as data scientists review an automated resume-screening system for gender bias, they could use a metric that ensures similar percentages of candidates are selected (known as parity) or one that is equally predictive of future successes among candidates (known as equal opportunity), or, if the company is striving for a more representative workforce, they can ensure the system recommends a diverse set of candidates.
As a result, leaders need to steer their organizations toward defining and setting metrics that best align AI with company values and goals. CEOs should make clear exactly what the company goals and values are in various contexts, ask teams to articulate values in the context of AI, and encourage a collaborative process in choosing metrics. Following employee concerns over AI projects for the defense industry, Google developed a broad set of principles to define responsible AI and bias and then backed it with tools and training for employees. One technical-training module on fairness has helped more than 21,000 employees learn about the ways that bias can crop up in training data and helped them master techniques to identify and mitigate them.
3. Advise on the hierarchy of company values.
AI development always involves trade-offs. For instance, when it comes to model development, there is often a perceived trade-off between the accuracy of an algorithm and the transparency of its decision making, or how easily predictions can be explained to stakeholders. Too great a focus on accuracy can lead to the creation of “black box” algorithms in which no one can say for certain why an AI system made the recommendation it did. Likewise, the more data that models can analyze, the more accurate the predictions, but also, often, the greater the privacy concerns.
What should a model-development team do in these instances if, for example, a company’s values state both to strive to build the best products and to always ensure customer satisfaction? A leader’s business judgment is necessary to help teams make the best decisions possible as they navigate trade-offs.
Leaders should also emphasize their organization’s diversity values and make sure they’re translating into diverse analytics teams. Diverse people bring a variety of experience, which gives rise to not only the innovative approaches needed to solve tough problems but also those required to prevent bias. For example, an all-male team building a resume-scanning model might generate a hypothesis that continuous employment is an indicator of good job performance, overlooking the need to modify the hypothesis to address how maternity leave can affect career history. Gender diversity, however, isn’t enough. Leaders should also stress other types of diversity, such as different ages, ethnicities, disciplines, and backgrounds to ensure teams represent a broad range of experiences and perspectives.
Beyond values: Five areas demanding leadership from the top
While ensuring that company values can be more easily applied to AI-development decisions is a foundational step in responsibly building AI, it’s not enough. There are too many instances in which well-intentioned and talented data-science teams have accidently waded into murky waters and their organizations were dragged into a riptide of negative press. Advances in AI techniques and the expanding use of AI only complicate matters by continually shifting the line that data scientists must walk. As a result, CEOs need to dig deeper, challenging analytics teams to evaluate their actions in the blistering heat of public opinion in five key areas.
1. Appropriate data acquisition
Data serve as the fuel for AI. In general, the more data used to train systems, the more accurate and insightful the predictions. However, pressure on analytics teams to innovate can lead to the use of third-party data or the repurposing of existing customer data in ways that, while not yet covered by regulations, are considered inappropriate by consumers. For example, a healthcare provider might buy data about its patients—such as what restaurants they frequent or how much TV they watch—from data brokers to help doctors better assess each patient’s health risk. While the health system believes acquisition and use of this data are in the best interest of its patients (after all, office visits are short, and this knowledge can help guide its physicians as to a patient’s greatest risks), many patients might perceive this as an invasion of privacy and worry that the data might paint an incomplete picture of their lives and lead to unnecessary or inaccurate medical recommendations.
As a result, leaders must be vigilant in asking data-science teams where they acquire data from and how the data will be used, and challenge them to consider how customers and society might react to their approach. For example, a financial institution that wanted to provide additional assistance to financially vulnerable customers developed capabilities to identify digital behaviors that indicated likely mental health issues. However, the organization chose not to include this dimension in the final AI system, because of potential customer reaction to such classification—despite the best of intentions.
2. Data-set suitability
Ensuring data sets accurately reflect all of the populations being analyzed is a rightfully hot topic, given that underrepresentation of groups can lead to different impacts for different cohorts. For example, a facial-recognition system trained on a data set that included far more images of white males can fail to identify women and people of color as a result. While racial, gender, and other human biases top the list, leaders should also consider the impact of more mundane data biases, such as time-selection bias—where, for example, data sets used to train a predictive-maintenance algorithm miss a failure because they draw on only nine months, rather than several years, of data.
As history has shown, hard-working data scientists in the thick of deadlines and drowning in data can think they have covered all bases, when in fact they have not. For instance, we have seen many analytics teams exclude protected variables from a model input without checking whether there is conflation with other input variables, such as zip codes and income data.
As a result, leaders must ask data-science teams fairly granular questions to understand how they sampled the data to train their models. Do data sets reflect real-world populations? Have they included data that are relevant to minority groups? Will performance tests during model development and use uncover issues with the data set? What could we be missing?
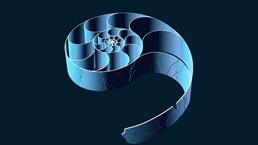
Confronting the risks of artificial intelligence
3. Fairness of AI outputs
Even when data sets do reflect real-world populations, the AI outputs may still be unfair due to historical biases. Machine learning algorithms, which have driven the most recent advances in AI, detect patterns and make predictions and recommendations from data and experiences. They have no awareness of the context in which their decisions will be applied or the implications of these decisions. As a result, it is easy for historic human biases and judgment to cloud predictions—anything from which prisoners should get paroled to which customers should get loans or special offers to which job applicants should get interviews. Even sophisticated organizations can “sleepwalk” into industrializing and perpetuating historical bias, as a leading tech company found when it discovered that its resume-screening algorithm was discriminating in favor of male candidates (because, historically, men predominantly held the roles for the position to be filled).
Leaders therefore need to frame and ensure adoption of a thoughtful process around “fairness by design”—first by establishing definitions and metrics for assessing fairness, as described earlier, and then continually challenging data-science teams to consider fairness during the full range of their work when doing the following:
- Choosing data. Maximizing fairness is not as clear-cut as removing a protected attribute or artificially accounting for historical bias. For instance, excluding gender in the resume-screening application might lead to a false sense of fairness, as the aforementioned technology company found out. Likewise, inflating the proportion of female applications included in the data sets may level the playing field for women but lead to unfair outcomes for other categories of applicants. It’s important for leaders to discuss with their teams what historical human biases might affect their AI systems and how the company can address them. In this example, to ensure stronger representation of women applicants, a company might need to collect gender data to measure the impact of gender inclusion but not use that data in training the AI model. While collecting protected or sensitive attributes can be essential to demonstrate that an AI system is acting fairly, strong data governance is required to ensure that the data are not then used for any other purpose.
- Choosing “features” from the raw data. In building algorithms, data scientists must choose what elements (known as features) from the raw data an algorithm should consider. For the resume-screening system, these features might include the length of time applicants have been at their previous job, what level of education they have achieved, or which computer languages they are proficient in. Selecting these features is an iterative process and somewhat of an art. Data scientists typically work with experts from the business to generate hypotheses about what features to consider, identify the necessary data, test, and repeat. The challenge here is that if they measure model performance against a single metric (such as the accuracy of the prediction), they risk missing real-world realities that might compromise fairness. For example, a hypothesis to consider length of time in prior roles might not account for frequent job changes military spouses often make due to relocations. Leaders who encourage their teams to use a wide range of metrics to evaluate model performance and to curate features under the auspices of the larger business goals can help safeguard for fairness.
- Developing, testing, and monitoring models. It’s easy to assume that if teams choose the right data and the right features, the resulting algorithm will deliver a fair outcome. However, there are at least a dozen commonly used modeling techniques, and different approaches (or combinations of them) can yield different results from the same data sets and features. During development, teams typically test model performance (for example, is the model performing as expected?) and are increasingly bringing in specially trained internal teams or external service providers to conduct further tests. The same rigor should be applied to testing models against the organization’s definition and metrics for fairness. And, just as model performance should be monitored throughout the life of an AI system, model fairness must also be monitored by risk teams to ensure that biases don’t emerge over time as the systems integrate new data into their decision making. For instance, a leading pharmaceutical company used machine learning models to identify clinical-trial sites that were most at risk of having patient-safety or other compliance issues—the early versions of the models were repeatedly tested against on-the-ground reality, and the hundreds of users of the model outputs were trained to flag any anomalies.
4. Regulatory compliance and engagement
In the past, organizations outside of regulated industries such as banking and healthcare often had a lower bar when it came to data-privacy protections. With existing and emerging regulations, such as the General Data Protection Regulation (GDPR) or the California Consumer Privacy Act (CCPA), all leaders have had to reexamine how their organizations use customer data and interpret new regulatory issues such as the right to be evaluated by a human, the right to be forgotten, and automatic profiling. To that end, in the United States, telecommunication operators, for example, have pledged to stop selling data to location-aggregation services amid reports that private user data had been used without prior consent. It is incumbent on leaders not only to ask their teams what regulations might be applicable in their work and how they ensure compliance but also to make sure that their data-science, regulatory, and legal teams collaborate to define clear compliance metrics for AI initiatives.
Additionally, leaders must encourage their organization to move from a compliance mind-set to a co-creation mind-set in which they share their company’s market and technical acumen in the development of new regulations. Recent work in the United Kingdom between the Financial Conduct Authority (FCA), the country’s banking regulator, and the banking industry offers a model for this new partnership approach. The FCA and banking industry have teamed in creating a “regulatory sandbox” where banks can experiment with AI approaches that challenge or lie outside of current regulatory norms, such as using new data to improve fraud detection or better predict a customer’s propensity to purchase products.
5. Explainability
There is a temptation to believe that as long as a complex model performs as expected, the benefits the model delivers outweigh its lack of explainability. Indeed, in some applications, not understanding how an algorithm made its prediction might be acceptable. For example, if a healthcare application uses image classification to conveniently, consistently, and accurately predict which skin blemishes are at high risk for skin cancer, a patient is unlikely to worry about whether the model uses the shade of the blemish, its shape, its proximity to another freckle, or any of a million other features to drive its recommendation. Ultimately, the patient’s concern is whether the recommendation is correct or not—and, if the patient is at high risk, what he or she can do about this prognosis.
In other cases, however, having an opaque model may be unacceptable (for example, it is reasonable for job or loan applicants to want to understand why they were turned down) and even a hinderance in adoption and use (for example, a store manager likely wants to understand why the system is recommending a particular product mix for his or her store before acting on the advice). The ability to explain model outputs to stakeholders is a major lever in ensuring compliance with expanding regulatory and public expectations and in fostering trust to accelerate adoption. And it offers domain experts, frontline workers, and data scientists a common language through which to discuss model outputs, so they can root out potential biases well before models are thrust into the limelight.
The nascent but rapidly maturing field of “explainable AI” (sometimes referred to as XAI) is starting to offer tools—such as SHAP (SHapley Additive exPlanations), LIME (Local Interpretable Model-Agnostic Explanations), and activation atlases—that can remove the veil of mystery when it comes to AI predictions.
To ensure model outputs can easily be explained to stakeholders, leaders must probe their data-science teams on the types of models they use by, for example, challenging teams to show that they have chosen the simplest performant model (not the latest deep neural network) and demanding the use of explainability techniques for naturally opaque techniques (Exhibit 2). One analytics team at a media company routinely uses such explainable AI techniques for its marketing reports, so the executive team can understand not only which customers are most likely to churn within a given period but also why. XAI allows the use of more performant predictive models while enabling the marketing team to take data-driven preventive actions to reduce churn.
There are no easy answers here. But leaders who sharpen and unpack their corporate values, build teams with a diversity of perspectives, create a language and a set of reference points to guide AI, and frequently engage with and challenge AI-development teams position themselves to create and use AI responsibly.
Importantly, responsible AI builds trust with both employees and consumers. Employees will trust the insights AI delivers and be more willing to use them in their day-to-day work and help ideate new ways to use AI to create value. Consumer trust gives you the right to use consumer data appropriately, and it is these data that power and continually improve AI. Consumers will be willing to use your AI-infused products because of the trust they have in your organization, and happy to use them because they just keep getting better. It’s a virtuous cycle that drives brand reputation and an organization’s ability to innovate and compete and, most important, enables society to benefit from the power of AI rather than suffer from its unintended consequences. And if that’s not something to be proud of, what is?