Achieving the level of customization, personalization, and operational efficiency necessary to compete in an increasingly fast-paced, digital environment is top of mind for nearly every business executive in any industry.
By now, many recognize artificial intelligence (AI) as a critical enabler in these pursuits. They’ve begun to envision a bold AI-powered future where AI helps, for example, retailers deliver individualized products before customers even request them or manufacturers customize products on demand and deliver them the same day via small, local factories that reside in every city.
However, many executives at incumbent organizations have yet to recognize what it takes to get there. While most acknowledge the need to rethink business models for digitization efforts, we don’t see a similar understanding around the use of AI. It’s a major impediment to getting real value from a technology that’s already providing significant returns for some companies.
Based on our experience working with hundreds of clients, we find that organizations are most successful at implementing AI when they start by reimagining a core process, journey, or function (what we call a domain) end to end with the technology. In so doing, they can take full advantage of AI while reducing development time and costs and ignite an organic cycle of change throughout their organization for achieving their bold visions.
In our recent article, “Getting AI to scale,” published in Harvard Business Review, we discuss in depth how leaders can apply this approach, and provide illustrative real-world examples. Here, we summarize five steps leaders can take to get it right.
Would you like to learn more about McKinsey Analytics?
Rightsize the challenge
When it comes to applying AI, organizations often consider one of two paths.
Some look at applying AI to solve discrete problems, plugging it into existing processes to automate them or add insights. Others consider undertaking a complete makeover of their business, trying to overhaul the whole organization with AI all at once.
Neither of these routes, however, can deliver the level or speed of change companies require to grow and thrive in the digital age. To be sure, discrete use cases can provide incremental efficiencies. But they’re too constrained to drive a material change in how companies operate or in their bottom line, and they’re difficult to scale. Conversely, while all-encompassing efforts promise bold returns, they typically have too many moving parts, stakeholders, and AI initiatives to achieve meaningful impact quickly, if at all.
So, what is the right approach?
We advise leaders to identify and prioritize up front those business domains that are broad enough where new ways of working enabled by AI can significantly improve financial performance or customer or employee experiences but that are confined enough to deliver results in under 18 months. These areas should have several characteristics that can enable an effective transformation with AI:
- A set of interrelated business activities. Look for areas that, when addressed together, will solve systemic business problems, such as chronic process inefficiencies, rapidly fluctuating customer demand, or difficulties getting products to customers.
- A business sponsor and team. Domains ripe for reinvention with AI have a supportive senior business leader (or two); business staff that can fill roles such as product owner, translator, and change lead; and a group of frontline “users.” AI practitioners can either exist in the business area or be pulled in from a center of excellence.
- Reusable data and technology assets. It’s important to select domains where the data and technology components necessary to run the AI models overlap, so each new AI project within the domain can build off past work, rather than start from scratch every time.
Most leaders can likely identify around eight to ten domains where AI can transform their business. However, we find that companies are most successful when they focus on one or two priority domains to start—based on value, feasibility, and leadership support—so they build up their capabilities and skills before expanding further.
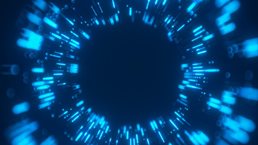
The state of AI in 2020
Assemble and empower teams
AI innovation doesn’t happen in a vacuum or solely among a group of data scientists. It sprouts from a team of people from business, digital, analytics, and IT functions.
In some cases, we find that most of the people necessary for reconceiving a given domain will already report into that business area. In such cases, leaders need only to shift individuals to new “execution” teams.
In other cases, however, leaders will need to reassign people from different parts of the organization into a single area. Setting up this team for success requires enabling it to be fully resourced and self-sufficient. Leaving team members in separate silos of the business creates delays and disconnects as requests for input and approvals move from one department to another. Empowering the team to design, build, and support new ways of working also facilitates excitement and ownership among the team.
Reimagine business as usual
Typically, we find that companies are best served by using first principles or design-thinking techniques to work backward from a critical goal or challenge. They might, for example, want to create a five-star customer experience and so would begin by sketching out what that might look like and then move to detailing how they could achieve it.
Execution teams should be prepared to spend time with end users early on to understand existing ways of working along with any inefficiencies and disconnects in the process, as well as to map out what the ideal process or journey might look like. Use of agile sprints can enable teams to rapidly develop prototypes of new capabilities in a few weeks and then test and refine the systems with users before widespread deployment.
Invest in organizational and technological change
On the organizational side, leaders will need to prepare, motivate, and equip their workforce for working in new ways. This includes a shift to interdisciplinary collaboration, data-driven decision making, and agile mindsets. In fact, companies reporting the highest returns on AI are more likely to enact effective change-management practices, with leaders modeling these behaviors.
On the technology side, organizations should focus first on those technologies that can accelerate AI development, such as cloud-based data platforms, application programming interfaces (APIs), microservices, and modern DevOps practices. We find that deployment of these technologies is achievable in just a few months and can enable companies to develop new business capabilities two to three times faster.
Beyond this, teams should triage additional investments as required to deliver new AI capabilities—for instance, adding new data sources as they’re needed rather than building out a 360-degree view of customers from the start. They should also assess how the solution will integrate with and impact upstream and downstream processes, and implement measures to address it, such as aligning employee incentives with the new process and developing reporting tools to provide transparency across the company to the new insights.
Expand your efforts
Once AI development matures within the first few domains, organizations will have a repeatable playbook of methodologies and protocols and a mindset for reusability that enables them to accelerate AI innovation and pursue multiple domains in parallel. It’s best to sequence domains to continue to build off past work. This might lead companies to prioritize domains with similar data and skills (such as supply chain and logistics), or it might lead them to pursue the same domain (such as campaign management) in other business units.
Ultimately, as companies move from one domain to another, their pace will quicken, their AI capabilities will rapidly compound, and they will find that the future they imagined is actually closer than it once appeared.
For a deeper look at how leaders can more successfully deploy AI, read “Getting AI to scale,” on hbr.org.