Generative AI’s (gen AI) transformative potential promises to revolutionize business and propel up to $4.4 trillion in economic impact annually. Unprecedented adoption rates from companies across industries and sectors, as well as significant private investment, underscore this potential.
Capturing the value from technology, however, is never just about the tech alone. Companies looking to move beyond running gen AI experiments will need to rewire how they work to achieve the full value of their efforts. One key component of this rewiring is developing strategic alliances1 with gen AI providers. The unique challenges of working with gen AI—from the lack of experience that many have in using the technology to gen AI’s instability and risk to the technology’s rapid pace of change—have made collaborations increasingly vital.
While many companies are already working to some degree with gen AI providers, outdated notions of what to look for in a provider and how strategic alliances should function are putting these efforts at risk. To make the most of strategic alliances, companies should focus on three areas:
- Go deeper on collaboration. Working with providers on gen AI programs requires a greater degree of trust and collaboration than has been necessary with traditional vendors, with thoughtful transparency, frequent communications, and explicit alignment across planning, development, and ongoing management.
- Zero in on providers who provide scalability, interoperability, and reusability. No single provider can offer companies everything they need. Achieving scale with a range of providers means understanding not only how well providers can scale but also how well their solutions will work with other components in a company’s gen AI ecosystem.
- Stay in control of your destiny. It is important to strike a balance between building on a provider’s capabilities and becoming overly dependent on them. That means investing in a flexible infrastructure, monitoring provider performance continually, and tying compensation to outcomes while being clear about intellectual property (IP) boundaries.
Go deeper on collaboration
As many companies are learning, the “build versus buy” approach to creating gen AI capabilities falls short when it comes to harnessing gen AI’s full potential. Building solutions entirely in-house can be time consuming and resource intensive, especially given the lack of gen AI talent at most companies.2 And while buying existing gen AI products or services can provide quick access to proven solutions, these solutions often require experienced gen AI workers to customize them to what the business really needs.
Collaborating with providers, on the other hand, can offer significant benefits in terms of access to the latest capabilities and expertise, development speed, and tailored solutions. But effective strategic gen AI alliances work differently from traditional vendor relationships. The technology is still rapidly maturing, implementation is complex, and stability issues bedevil solutions, requiring closer collaboration and higher degrees of trust. Sharing data to fine-tune models, for example, can happen only if client companies trust strategic allies to protect it effectively. Similarly, the complexity of addressing root cause issues in AI models, many of which are not yet fully stable, necessitates both clear lines of communication and alignment on resolution protocols. This level of trust and collaboration should be established on three essential building blocks:
-
Cocreation of solutions. For most companies, the greatest value from gen AI will come from adopting established capabilities, which providers offer, and tailoring these capabilities to companies’ unique data. This requires a highly iterative and collaborative process where the company and provider work closely together to source and prepare the right data, engineer relevant prompts, fine-tune models based on specific use case needs, and test and iterate on the models in the field. To manage the range of providers a company might need to work with, it will be important to institute frequent touchpoints to share updates, discuss challenges, and align on priorities across providers. Dedicating time for in-person workshops and co-innovation sessions, celebrating milestones, and sharing learnings openly during this process is also critical for building trust.
When a luxury retail company, for instance, partnered with a gen AI provider to create a personalized product recommendation system, the company shared its vast catalog of product information, including detailed specifications, features, and customer reviews. The company also provided valuable insights into the nuances of customer preferences and behaviors, such as how they pose queries. This helped the provider engineer relevant prompts, fine-tune the gen AI models to understand and interpret this domain-specific data, and comprehend the specific language, terminology, and attributes used to describe luxury products.
This collaboration was instrumental in allowing the resulting model to surface the most appropriate products, even for complex or ambiguous queries, with over 99 percent accuracy. Early results indicate the solution could yield a 10 to 20 percent improvement in the conversion rate from product discovery to purchase, a significant leap for the luxury industry.
-
Joint planning. It is essential for the gen AI provider to offer visibility into its product road map, including upcoming features and capabilities, and possibly grant access to alpha or beta releases. This allows the client company both to anticipate how the provider’s offerings might evolve to meet the company’s own future needs and to possibly influence the direction of the road map. The road map can also be aligned when the company shares its strategic goals and relevant customer insights to help the provider better understand the company’s needs.
This level of communication and coordination is particularly important given that companies will likely be working with different gen AI models and applications—often developed by different providers—that need to be closely integrated for a solution to work well. Potential benefits of providing clarity and transparency include helping various vendors align their road maps and identify dependencies (for example, between multiple models that need to work together to deliver a specific gen AI solution). One area where we’ve seen big dividends is investing significant time together up front—often meeting every couple of days for two to four weeks—to work out a mutual road map and system dependencies. The output from this effort should include a primary project plan that captures milestones and dependencies between providers so the client company can better manage and coordinate all parties.
One leading technology company adopted this approach. It engaged in joint planning sessions with its gen AI providers to identify high-impact use cases to strengthen its core product. The company shared valuable customer analytics insights and outlined its long-term vision for AI-driven innovation, which helped the gen AI providers develop a more aligned road map. For their part, the gen AI providers offered early access to new gen AI features and models, allowing the company to test and provide feedback before general release. Through these collaborative-planning efforts and continual communication about road map needs and adjustments, the company was able to reduce time to market for deploying at-scale solutions. For instance, it launched a personalized marketing campaign powered by gen AI within six months of starting the strategic alliance, resulting in a significant boost in sales conversions.
-
Risk and investment sharing. Gen AI programs often require sizable investments in specialized hardware, large-scale data acquisition and tagging, and extensive computational resources for model training. In addition, the risks associated with adopting gen AI capabilities—from hallucinating models to privacy issues—require close attention. For these reasons, companies should consider how best to distribute among providers financial, technological, and operational resources and risks associated with gen AI development. Companies and providers, for example, should be explicit in defining specific risks associated with the gen AI project, such as data privacy breaches, model biases, or IP infringement. These agreements will ideally outline each party’s responsibilities for mitigating and managing these risks, as well as any financial or legal liabilities.
The same technology company mentioned earlier shared risks with their gen AI providers by structuring contracts around outcomes instead of token usage. This approach allowed the company to manage uncertainties and costs while aligning incentives and fostering a shared commitment to success.
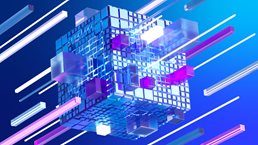
Learn more about McKinsey’s strategic alliances capability
Zero in on providers that offer scalability, interoperability, and reusability
A single provider that can provide all the best components for an effective gen AI solution doesn’t exist, at least not yet. The variety of components and models needed to work together across the tech stack means that companies will need to team up with a curated network of specialized tech providers.
In developing an ecosystem of providers that can scale, the component parts working together are more important than the parts individually. Companies need to weigh those criteria that enable the overall gen AI system to work most effectively. Selecting the right providers has become particularly challenging given the growth in the provider landscape. In fact, since the launch of ChatGPT in November 2022, the number of open-source large language models (LLMs) and commercial LLMs has quadrupled.3 Furthermore, there are currently more than 1,000 AI vendors, with more than 600 new products introduced over the previous year, mostly spurred by gen AI.4 To that end, companies should zero in on three key criteria when selecting providers:
- Scalability. Given the mind-boggling scale of gen AI—from the amount of data needed to train and fine-tune models to the number of queries models respond to—providers should have a proven track record of handling increased volumes of complex traffic and user queries without compromising performance. Companies should pressure test pilot programs, which often do not replicate live conditions and are typically not a good barometer for scaling readiness. When evaluating scalability, it is important to look for providers that can commit to specific milestones, such as handling a 50 percent increase in user queries over six months without degradation. Providers should also be willing to regularly review and adjust milestones and contracts to ensure alignment with evolving goals.
- Reusability. Reusing code can accelerate the development of gen AI use cases by 30 to 50 percent, so it’s critical that providers offer solutions that can be easily repurposed across multiple projects.5 Companies should therefore look for providers that offer flexible, modular components and pretrained models (such as customizable natural-language-processing modules or configurable data pipelines) that can be fine-tuned and adapted to various contexts. They should also seek providers that offer tools and frameworks (for example, intuitive APIs and software development kits for integrating and extending gen AI components or drag-and-drop interfaces for model fine-tuning) that can enable the easy customization and extension of solutions.
- Interoperability. Interoperability between models and components is crucial for creating a cohesive, efficient, and scalable gen AI ecosystem. When evaluating model or solution interoperability, companies should look for providers that adhere to industry standards and best practices for data exchange, API design, and software development (for example, standard data formats such as Apache Avro and JavaScript Object Notation, established machine learning frameworks like PyTorch, or data governance standards). Providers should use widely adopted programming languages, offer well-documented and easy-to-use APIs, and support smooth integration with the company’s data sources, applications, and platforms.
These three criteria are crucial for selecting gen AI providers that can scale, but companies should also ensure that all providers meet a high bar on other criteria, such as ethical guidelines and adherence to local privacy and tech sovereignty regulations. Establishing and aligning around clear data governance and security protocols can go a long way toward building trust with providers.
Stay in control of your destiny
Finding the sweet spot between forging close strategic alliances and maintaining agency over the broader direction and vision of these collaborations presents a critical challenge for organizations. Companies looking to maintain independence and control of their destiny would do well to consider the following guidelines:
- Establish a flexible infrastructure. A flexible, scalable gen AI infrastructure can serve as a foundation for quickly integrating different providers. This “chassis” could be a centralized platform or a set of well-defined APIs, integration protocols, and data formats that enable different gen AI components to work together seamlessly. To ensure maximum flexibility, companies can adopt MLOps (machine learning operations) best practices, such as containerization, automated testing, and continuous-integration and continuous-delivery (CI/CD) pipelines. These practices help ensure the reliability and performance of the gen AI stack and allow for rapid rollback of changes if issues arise.
- Continually monitor model performance. Companies need to maintain a clear understanding of what providers are building to avoid receiving a “black box” solution. They should ensure that providers include proper documentation and sufficient transparency during development. Robust monitoring and testing capabilities are needed to track provider performance and identify issues early (for example, automated reporting capabilities to collect and aggregate relevant metrics, including model inputs and outputs, latency and throughput statistics, and user feedback). It’s important to regularly conduct end-to-end tests of the gen AI solution—from data ingestion to model outputs—so as to track performance and identify the source of a problem across the provider ecosystem. Experience has shown that involving all providers in establishing a comprehensive testing strategy (joint integration testing and scenario testing, for instance) helps to set clear expectations and responsibilities.
- Establish clear IP boundaries. Difficult questions about IP are still being worked through with respect to gen AI, so it is important to establish clear boundaries up front. Companies should specify, for example, the existing IP that each party brings to the collaboration, such as proprietary data sets, algorithms, or models. They should define how IP that is created during the collaboration (for example, any patents, copyrights, or trade secrets) will be owned and managed, including predefined terms for licensing, commercialization, and revenue sharing. And they should outline a process for tracking and attributing individual contributions to the codeveloped IP, which can help prevent disputes and ensure proper recognition of each party’s contributions. Being transparent and assuring alignment during this process can also help build trust.
- Tie compensation to outcomes. While following best practices on contract structure (for instance, including clear KPIs, service level agreements, and licensing arrangements) and specifying risk-sharing provisions, it is critical to tie a provider’s compensation to measurable outcomes such as model accuracy, uptime, and user satisfaction. Companies should avoid minimum spend requirements and include clear exit clauses and data portability requirements to avoid limiting flexibility.
Getting started
As the transformative potential of gen AI continues to unfold, companies should act decisively to position themselves for success in this new era. To get started, executives can consider the following actions:
- Establish a steering committee made up of key stakeholders from business, IT, legal, and procurement to oversee the gen-AI-alliance strategy. The committee should be tasked with defining strategic-alliance criteria, setting performance metrics, and establishing governance guidelines. To do so, the team needs sufficient autonomy to make decisions within strategic guidelines.
- Develop a strategic gen-AI-alliance playbook that includes a standardized framework for evaluating, onboarding, and managing new gen AI providers. This framework should include guidelines for assessing scalability, reusability, and interoperability, as well as templates for contracts, service-level agreements, and performance dashboards.
- Conduct a strategic gen-AI-alliance audit to assess current strategic alliances and identify gaps, redundancies, or misalignments with the gen AI strategy. Determine which strategic alliances to maintain, expand, or phase out based on their potential to spur business value.
- Assign dedicated relationship managers to the gen AI alliance. The managers should have a solid understanding of gen AI technologies, architectures, and best practices so they can effectively communicate with providers, assess their capabilities, and ensure alignment with the company’s technical requirements. They also oversee the entire gen AI ecosystem and act as the “central authority” to help coordinate activities among providers, monitor progress, and resolve issues. In many cases, it will be useful to have a solution architect on board, as well as to regularly meet with providers to understand exactly what they are doing and how they are progressing.
Building trust and fostering collaboration are just as important as choosing the right technology. Companies should start small, learn fast, and iterate often to ensure that they are well on their way to unlocking the full potential of gen AI.