Executives have seen that the move from running artificial intelligence (AI) experiments and proofs of concept to capturing lasting value at scale requires an investment in strong foundations. These include aligning AI with core areas of the business; embracing important cultural and organizational shifts; and investing in new kinds of technology, training, and processes for building AI.
More and more organizations are adopting these basic practices, and those that do tend to report the highest bottom-line impact from AI. But successful organizations don’t just behave differently; our experience in thousands of client engagements around analytics and AI over the past five years shows that they also think differently about AI. At these companies, AI is etched in the collective mindset (“We are AI enabled”), rather than simply applied opportunistically (“Here’s a use case where AI can add value”).
Having this mindset means deeply internalizing the long-term competitive benefits of augmenting human decision making, processing data from many sources at a massive scale and enormous speed, and continuously adapting business models and operational strategies based on signals from the data.
It means valuing collaboration and continuous learning over individual knowledge and experience, with employees seeking out new data, skills, workflows, and technologies for driving ongoing performance improvements. Individuals and the collective crave not just to know more than they did, say, last year, but to know more in the future.
Finally, this mindset embraces end-to-end thinking and consistent architectural principles over siloed solutions when combining new technologies and tools with existing infrastructure.
This shift is not easy. Leaders must reorient their own thinking and then move every mindset in the organization. We find that the leaders who succeed in this effort do so in a few ways: they reorient the company’s focus toward its multiple instead of its earnings, and they actively emphasize and encourage global learning loops and technological adaptability throughout the organization. Although this mindset shift doesn’t supplant an investment in strong foundations for AI, organizations that embrace an AI-enabled mindset are better at meeting the most formidable technical and cultural challenges and making organizational changes so they can reap the full value that the data and technology offer.
We’ll explore the levers companies can use to make the shift and, since mindsets can be tricky to measure, offer ways to gauge if the shift is working. To show the utility of these ideas, we’ll describe experiences of two industry giants whose leaders are betting their futures on winning with an AI-enabled mindset. One of them is a global pharmaceutical company whose multiyear AI transformation achieved a 10–15 percent reduction in patient-enrollment times for clinical studies and a 10 percent gain in productivity across its initiatives, allowing it to redirect hundreds of millions of dollars toward other pressing needs. It is now on course to increase the rate at which drugs can safely be brought to market and replicate this ever-growing cycle of improvement in other divisions, such as manufacturing. The other company, a leading bank, is targeting a 50 percent reduction in time to value for new use cases across the organization as it makes the mindset shift, enabling it to rapidly deploy many hundreds of AI models that drive continuous learning and increase its annual revenue run rate with its first AI-driven learning system.
Would you like to learn more about McKinsey Analytics?
Setting sights on the multiple instead of earnings
When it comes to AI, some CEOs tend to focus on how it will drive profitability in the next earnings cycle, leading them to pursue often-unrelated use cases that offer quick and measurable financial benefit. However, in our experience, such short-term thinking typically results in incremental changes at best—for example, a one-time reduction in customer churn or a single boost in efficiency for a given operational process.
By contrast, leaders at AI-enabled companies take a more systematic view, focusing on their company’s multiple, an indicator of long-term ability to add value to the organization. This requires company leaders to agree that the purpose of AI is to fundamentally transform the way the business conducts its day-to-day operations. In practice, that means using AI in the end-to-end process of capturing every event or data point from customers, processes, or machines (a click, transaction, milestone, indicator, or sensor) to ensure that consequent actions, decisions, and interactions are more focused and effective. This sets the stage for a continuous loop of learning and improved performance, which we detail in the next section.
Take, for instance, a global pharmaceutical company that shifted from being a traditional drugmaker to operating as an AI-enabled company. To align all leaders and smooth the transformation, the CEO gathered nearly 150 direct reports from ten regions for a three-day leadership workshop. They discussed industry changes and relevant trends in innovation likely to occur over the next five to ten years, and they brainstormed how they could disrupt their own organization to improve performance dramatically. This workshop was the first activity in a wider capability-building effort being carried out through a formal analytics academy.
The CEO appointed new data and analytics leaders and committed to several additional senior hires, including a lead translator with strong knowledge of AI, the business, and change management to drive the AI program.
The chief executive also restructured the organization to flatten hierarchies, so frontline teams own the responsibility to act on the new AI insights. This independence is foundational; it gives employees the encouragement and confidence necessary to widen their aperture from identifying, for example, which customers are churning (which drives a one-time performance improvement) to taking actions that bring the company closer to its customers, which opens a new wave of potential. AI training for employees further bolstered their confidence and aptitude to apply the technology.
Finally, the CEO tasked the company’s clinical-trials function (a high-value area that was knee-deep in data and experiencing significant variations in quality, efficiency, and speed across clinical trials) with bringing people together from across the trial life cycle to rethink the process from the ground up with AI.
How to get started
Getting all leaders to support this shift is imperative. The most successful companies we see have a CEO who lays the groundwork for support up front. Such leaders take time to explore and share examples of AI-enabled companies inside and outside their industry and hire AI-experienced senior talent to fill the leadership positions required to help drive the change, if the talent doesn’t already exist in the organization. They also reduce hierarchy, make AI education a priority, and consistently communicate at every level the strategic nature of these changes. (For more on how to enact these changes, see “What it really takes to scale artificial intelligence.”)
Forging global learning loops with and for AI
For employees to keep raising the performance bar as required to maintain growth, there must be a mechanism for capturing the experiences, experiments, and learning occurring across the organization. At many businesses, learning typically gets stuck in the mind of one individual, team, business unit, or silo, rather than contributing to the organization as a whole. In contrast, AI-enabled companies develop the skills, processes, and technical systems to build global learning loops that turn individual knowledge and local insights into an ever-increasing flow of collective wisdom that everyone in the organization shares and contributes to. These learning systems codify valuable knowledge gained from the frontline business systems (operated with insights derived from AI at scale and speed) and the AI teams’ approaches to processing data and developing AI models for solving business problems.
One of the best ways to create this global learning loop on the business side is through the development of an AI-driven nerve center for managing operations. The global pharmaceutical company, for instance, developed what it calls its “clinical control tower” that continually updates and shares findings derived from the diverse data gathered from hundreds of clinical trials across thousands of sites around the world. This system enables decision makers to understand in detail what drives variations among clinical trials (in speed, quality, and cost) and delivers predictions that enable interventions to reallocate resources and avoid delays and waste.
This is not a one-off change where employees leverage a prediction and then return to business as usual. With every trial they run, clinical-trial managers and operators learn more about the outcomes of various decisions from across all trials, such as when audit visits are scheduled in a different way or different components of the supply chain are used for distributing drugs to trial sites. Managers can adjust their strategies based on these insights. They can also run different trial scenarios to test ideas, generating more data and revealing new patterns. All of this is automatically incorporated into the learning system, so everyone can build upon it and feed the continuous learning loop.
This loop is being reinforced and replicated both within and well beyond clinical operations. Thousands of employees today use this system in their daily work. With word spreading, the company is now adding more data and investing in additional AI tools for employees in other divisions.
On the technical side, AI-enabled organizations standardize processes in order to scale AI more swiftly, successfully, and safely. They design capabilities for scale from the start and create integrated, comprehensive protocols to build and deliver AI tools that institutionalize learning loops and avoid the increased technical debt and complexity caused by incremental, uncoordinated technology choices (Exhibit 1).
One leading bank achieved this standardization by investing in a “protocol infrastructure” that provides AI teams with templates, guides, best practices, and checklists. The infrastructure spans more than a dozen topics, covering the most frequent activities at each stage of project delivery, and is integrated within a workflow and knowledge-management application. This enables the bank to ensure that everyone speaks the same analytical language when developing AI solutions, that all AI teams use current best practices, and that there’s a documented path to improve practices systematically over time.
In constructing this protocol, the company’s AI center of excellence gathered and codified best practices and advice from team benchmarks, surveys with AI experts throughout the company, and interviews with business leaders. Because they made the protocol an organization-wide effort, rather than a top-down edict, AI teams don’t view it as “overhead” but as an asset representing the shared wisdom of their colleagues. As a result, every lesson learned translates into benefits and time savings for users and the organization, and teams contribute to as well as draw on the advice captured within the protocol. For example, codified best practices outlining how to get models ready for deployment enable teams to start building production-ready AI tools from the first line of code, rather than after they have completed a pilot. This reduces the amount of rework needed, improves consistency and collaboration, and frees up time for more value-added work. Similarly, the protocol ensures that teams bake risk management into AI development, replacing ad hoc efforts or point-in-time assessments, which sometimes miss critical risks that AI can pose by introducing bias or compromising fairness, explainability, or information security.
These protocols are not at all static. Through regular and formal retrospectives following each AI deployment, teams continually add new knowledge to them, creating learning loops that repeatedly increase the rate at which every AI team can deliver new insights to the business.
As these learning loops converge and ripple across the organization, employees will naturally seek out more data from data owners. This in turn will increase their mutual understanding and pursuit of new opportunities with data and AI that enable greater speed and accuracy. It also will increase their knowledge about the elements of data gathering, cleaning, linking, and modeling—knowledge that more and more types of workers will need for success in an increasingly digital world.
How to get started
The way to get started with learning loops depends on the type you’re creating initially—an AI-driven learning system to improve operations or a protocol system to improve AI development. For the former, it’s best to begin in one high-value area of the company, using design principles to understand how employees make decisions, what decision support tools they currently use, and what assumptions they make based on their experiences. You build out from there, keeping the ultimate users involved in the process.
For a protocol system, it’s hard to codify practices on theory alone, so it’s best to build your protocol simultaneously as teams deliver AI use cases. Start by diagnosing your current state of AI use cases and understanding how AI practices vary across your organization. Then design the end state you’re after by coaching teams, developing early protocols, and testing them on live projects as you develop the governance needed to sustain adoption. Once a protocol is finalized, expand access and ownership to all subsequent AI teams and projects.
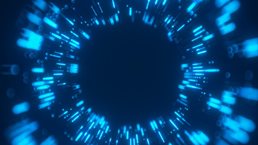
The state of AI in 2020
Advancing technological adaptability
For continuous learning loops to occur, AI-enabled organizations recognize that they need to support them with the right architectural and end-to-end technology choices. As many incumbent organizations have learned at their cost, legacy technologies are rarely fit for purpose in an AI-driven world, and adapting them is expensive and time-consuming. At the same time, as AI technologies rapidly advance, there is an obvious risk of increasing technical debt and complexity, so companies should think beyond what is fit for their purpose today. Instead, they should create an infrastructure where technologies can be easily integrated inside end-to-end processes to turn data into actionable insights and predictions—and easily swapped out for newer ones without breaking the entire system.
More flexible infrastructure, appropriate data-management capabilities, reusable modular components, and scalable tooling for collaboration are just some of the requirements for becoming AI enabled. Of course, associated workflows and roles often must be aligned with these technologies to capture benefits of greater agility and speed. The move from an old monolithic architecture to a more modern data architecture won’t happen overnight, but an important start is to reframe all technology investments as components contributing to global learning loops.
AI-enabled organizations also recognize that continuous performance improvement at the model level requires adaptability in the process of AI model design. To achieve this, their protocols emphasize opportunities to refresh and improve models as well as the often-neglected monitoring of their performance after deployment. They also stress the development of reusable tools and components such as model and feature libraries and AI workbenches, which not only accelerate the development process by enabling teams to build on previous experience and learning but also build in compliance by design and explainability.
Greater explainability of models is more important to cultivating an AI mindset than it might appear to be at first glance, because explainability essentially keeps learning loops intact. On the development side, explainability enables AI teams to understand the choices and assumptions of the initial model authors and to adapt models more quickly to market changes. As many leaders saw firsthand in the early days of the COVID-19 pandemic, rapidly changing customer behavior patterns and market indicators can break some models, and fixing them is difficult without visibility into how they were built. On the business side, the better employees and users understand how a model works, the more likely they are to trust and use it. Equally important, this understanding equips them to know when to override a recommendation because the model may be operating on assumptions that are no longer valid.
At the previously mentioned bank, best practices for explainability are outlined in its protocols and included in a model-development and -assessment questionnaire. This ensures that AI teams anticipate the need for end users to understand the inner workings from the start. It also signals the model-development team to involve users in the development process, which gives users the confidence to apply model recommendations and participate in improving how the model performs over time.
How to get started
Context is everything, so there is no silver bullet or one-size-fits-all solution for achieving technological adaptability. There are, however, road-tested blueprints and practices that leading organizations use to break through the data-architecture gridlock and accelerate modernization. (For a comprehensive discussion of these practices and how to get started, see “Breaking through data-architecture gridlock to scale AI.”) Additionally, a technology incubator, such as an innovation lab, can provide a space to test-drive and rapidly iterate on new AI technologies, techniques, and tools. Once matured, these can be scaled throughout the organization.
How to know it’s working
Once you’ve pivoted the focus to your growth multiple, established your learning loops, and started modernizing your infrastructure, you might see some early improvements. But it’s harder to see whether mindsets are shifting.
Many performance metrics, when regularly and collectively tracked, can help leaders (and all employees) understand their progress toward becoming an AI-enabled organization (Exhibit 2).
The global pharmaceutical company we have discussed tracks nearly two dozen key performance indicators that display each quarter’s clinical-trial performance at program, country, and site levels.
These metrics include clinical-trial cost (for instance, the percentage of countries achieving less than 50 percent of patient targets), quality (such as the number of quality issues per trial), and revenue (including the net present value of additional months at peak sales for new medicines).
Leaders should also watch for how employees embrace learning itself and the speed of the learning loops. To do so, they could measure employee contributions to the knowledge ecosystem, using metrics such as the number of contributors per month and frequency of new entries and articles. Companies also may look at how often employees use learning systems, as indicated by measures such as the number of knowledge-platform users, number of monthly active users, and total time spent on the platform.
Businesses will continually obtain new types and growing amounts of data to process, and new tools and techniques will become available to turn data into actionable insights and predictions. Meanwhile, the competitive operating environment is constantly in flux, in ways both predictable and, as we’ve seen during the COVID-19 pandemic, completely unexpected. Organizations with an AI-enabled mindset don’t just recognize this but embrace it, building a culture of curiosity and commitment to continuous learning and improvement. This gives them an edge in both AI and their business, today and for many tomorrows.