Doctors, healthcare providers, and caregivers are all too familiar with the problem: once patients are diagnosed and put on a treatment regimen, 50 to 60 percent are likely to skip medications, follow-up appointments, and other treatment protocols. The personal and economic costs of inadequate patient adherence are enormous. An estimated 125,000 unnecessary deaths occur each year in the United States because patients don’t follow their regimens,1 and the problem adds an estimated $290 billion to US health expenditures annually.2
Improving on traditional approaches to adherence
Given limited alternatives, the healthcare system has traditionally used a narrow range of interventions to encourage medication adherence. Generally this means warning patients of the consequences of deviating from treatment and scheduling a standard set of follow-up consultations across the entire patient population. Such an approach, which addresses all patients equally, may be costing billions of dollars in the United States alone in potentially ineffective interventions.
Stay current on your favorite topics
To have a significant impact on patient adherence, pharmaceutical companies can mirror efforts from the retail, banking, and technology industries, among others, and create a 360-degree perspective of patients’ lives, looking not only at whether they adhere to treatment regimens but also at contributing factors such as behavioral patterns, demographics, and lifestyle choices. Such rich analysis can help uncover opportunities to reshape behavior and craft specific interventions tailored to individual profiles.
One example comes from a leading airline, which used big data analytics and personalized marketing to improve satisfaction, increase purchases from members of its loyalty program, and raise enrollment in the program. By merging data from its internal customer-relationship-management program with that acquired from a consumer-profiling company, the airline created a full picture of loyalty-program members and prospective members, covering aspects such as buying patterns, social and economic characteristics, preferred media channels, and interests.
With this information, the airline developed algorithms to predict each customer’s propensity to purchase tickets and to customize offerings accordingly. For example, members might receive timely, individualized email offers for free bonus miles when staying in a partner hotel during an upcoming trip, in-flight menus targeted to their tastes, or unexpected seat upgrades if the algorithm predicted their loyalty was weakening. After only a year, the airline converted 4 percent more customers to its loyalty program, representing tens of millions of dollars in additional revenue. Over the past few years, many consumer-focused businesses have launched similar personalized-marketing initiatives.
The healthcare industry is primed to follow this example. Insurers, providers, pharmaceutical companies, and data aggregators have access to large volumes of data that can help predict behavior and enable tailored interventions if assembled and analyzed properly. For example, analyzing claims and consumption data can highlight a patient segment that tends to skip dosages during the first month of treatment and abandon therapy completely within six months. New direct-to-patient channels, such as patient hubs and social media, can be used to reach such patients directly with planned interventions that could improve adherence.
Would you like to learn more about our Life Sciences Practice?
Three crucial steps
Taking lessons from other industries, the healthcare sector could use a three-step approach to increase patient adherence: predicting which patients are most likely to lapse on their treatments, tailoring interventions for patients who need the most support, and building agile teams to deliver personalized interventions to individual patients when needed (Exhibit 1). Some companies have increased patient-adherence levels for chronic conditions by 5 to 10 percent using this type of tailored-intervention approach.3
Predicting patient behavior
Adherence patterns and individual motivations vary widely, even among patients undergoing the same treatment. One patient, for example, may stop taking medication because of its side effects, the inconvenience of returning to the doctor, and a fear that the doctor may chastise the patient for failing to follow the regimen. In contrast, another patient with the same diagnosis may routinely skip dosages because of a hectic lifestyle, frequent work travel, and misunderstanding the seriousness of the condition.
Advanced analytics can help uncover such traits for patient segments not adhering to treatment programs, construct patient profiles based on these patterns, and build predictive models to identify who is most at risk of nonadherence. In many cases, this may require the use of a third-party organization to ensure full compliance with the requirements of the US Health Insurance Portability and Accountability Act (HIPAA).
Generally, four critical dimensions of a patient’s life—social or economic aspects, the condition itself, the health system, and the medications—can drive nonadherence (Exhibit 2).
Data analysis across each of these dimensions is needed to fully understand how they affect a patient’s likelihood of following a treatment regimen.
To analyze the variables across these dimensions that have the biggest impact on adherence, anonymous data must be combined at the patient level, adhering to requirements of HIPAA or similar regulations in other geographies. Once the data is assembled, predictive models can be employed. Data can be gathered from a range of sources, including medical claims, prescription data, and consumer demographics and profiles—such as income, interests, purchase patterns, and media preferences (Exhibit 3).
Such models produce anonymous profiles of patient segments, in particular identifying drivers of adherence, and these segments should be prioritized based on estimated size and risk. The analysis defines patient characteristics for each segment and can earmark patients for targeted interventions. In some cases, variables are specific to a healthcare system or patient population, but in others they can be unique to individual conditions and regimens and require individualized analysis.
One pharmaceutical company identified three significant segments among patients suffering from a rare disease. Patients in one segment were strongly influenced by how effective their physician was at encouraging adherence among all patients. When patients in this segment were seen by a physician with low average adherence, they were five times more likely to drop off therapy within the first year. A second segment was more career minded, with busy work schedules. These patients were also far more interested in convenience and were more likely to switch to an alternative medication with less frequent dosages, even if they lost copay incentives. The final segment was characterized by a lack of family support and community involvement, such as support groups and volunteering. These patients were about a third as likely to remain adherent as those who had such a support system.
Designing tailored interventions
Once factors that can help predict nonadherence are identified, healthcare companies can develop interventions that target specific drivers at the optimal time. To allocate resources most effectively, these interventions will often focus on predictive factors that encompass the largest segments or those most at risk of lapsing.
To design tailored interventions, healthcare companies should begin by developing a full understanding of the drivers behind patient behavior. One approach is qualitative research focused on target segments and moments of opportunity when interventions could be most effective. Ethnographic interviews with target patients and their physicians, as well as surveys, can help provide deeper insight into these drivers.
Next, the design must address a patient’s, or physician’s, specific need at a particular time and provide a streamlined experience. Attempts to increase adherence often fail because they add to a patient’s burden—for instance, by requiring additional information to be entered into an app—rather than streamlining and improving the experience.
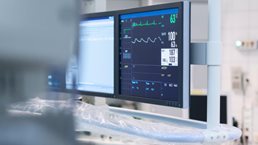
Using data and technology to improve healthcare ecosystems
For a skin-condition drug, analysis revealed that patients who frequently purchased towels were more adherent. Qualitative research with patients who agreed to be interviewed helped uncover the underlying causes—these patients were using the drug in conjunction with wet-wrap therapy, a procedure in which the patient applies a topical medication and wraps it in wet towels to seal it in. The pharmaceutical company then created an intervention plan in which its sales force encouraged physicians to discuss wet-wrap therapy with patients, which increased adherence to the drug.
In another case, a pharmaceutical company found that patients of smaller practices were more likely to lapse in administering a specific self-injected medication than those visiting larger clinics. Physician interviews showed that smaller clinics had less nursing support and were struggling to train patients properly on how to administer the drug. To solve the problem, the company started a nurse hub to support patients visiting smaller clinics. Adherence levels quickly matched those seen at larger clinics.
Effective intervention design requires identifying drivers that can predict nonadherence for each patient segment; prioritizing efforts based on the size of the segment, the risk, and the expected effectiveness of the intervention; understanding the variables fully; and then formulating an intervention that improves and streamlines patient experience.
Building agile capabilities
Interventions alone are not sufficient. Pharmaceutical companies should also follow other industries in building more flexible organizations—for example, by creating agile teams that can quickly assess the success of these interventions, identify any lingering patient challenges, and fine-tune or redesign interventions as needed to improve success rates. The effectiveness of interventions can be tracked at the patient or segment levels, depending on how the program is set up.
Agile operating models are critical to ensure that ideas are turned into interventions quickly and that feedback loops for testing effectiveness and informing revisions are maintained. These models help create greater collaboration across functions such as marketing, medical, and patient services; provide dedicated resources with appropriate skills in IT, analytics, legal, compliance, privacy, and other vital areas; and ease coordinated execution across all channels.
Programs to improve patient adherence will require new skills, especially centered on big data analytics. Data from multiple sources will have to be integrated and analyzed, while algorithms and predictive models must be developed. In addition, many companies will also have to build strong customer-centric organizations, developing a services mentality that understands and responds to the needs of patients.
To improve patient adherence, healthcare companies can draw valuable lessons from other industries on how to understand patients better based on their individual needs, preferences, and lifestyle choices. By adopting a 360-degree approach to understanding patient behavior, companies can raise adherence levels, reduce waste in healthcare systems, and contribute to better patient outcomes and lives.