Clinical development and the randomized clinical trial (RCT) remain largely unaffected by the unprecedented wave of innovation in pharmaceutical R&D fueled by developments in artificial intelligence (AI), including generative AI (gen AI) and foundational models. However, they are under pressure from the rise of precision medicine and a more competitive development landscape. So far, the adoption of AI for clinical development has emphasized operational excellence and acceleration, but advances in scientific AI have made this the time to leverage modern analytical tools and novel sources of data to design more precise, efficient trials with greater success rates.
The introduction of RCTs in the mid-20th century ushered in the modern era of evidence-based drug development. Their rigidity and simplicity were welcome defenses against an overreliance on anecdotes and case studies and have unarguably served patients well, supporting the introduction of countless safe, effective therapies. Yet RCTs are starting to be seen as bottlenecks that lengthen the time for therapies to gain approval and can increase costs.1 Meanwhile, as gen AI breaks milestone after milestone, patients eagerly await the translation of such unprecedented technological progress into pharmaceutical R&D that could deliver faster access to better treatments.
In addition to rising expectations about timelines, clinical development is also facing increasing demands to generate targeted and meaningful data. As precision medicine becomes mainstream, RCTs often must prove not only the general efficacy of a treatment but also whether it will benefit a specific segment of the patient population. The smaller that segment, the harder it can be to enroll enough patients in trials. In addition, the bar for what constitutes a clinically meaningful treatment effect is creeping higher to meet standards set by regulators and payers as well as competitive pressure. In most therapeutic areas today, there are, on average, 40 percent more assets per indication than in 2006,2 raising the need for greater differentiation. And clinicians and patients alike need more evidence to make decisions about the best available treatment at a given time.
All this comes as researchers accelerate drug discovery by making greater use of AI and gen AI, such as DeepMind’s AI-enabled platform AlphaFold, which can predict the 3-D structure of molecules3 leading to a pipeline of more and better-designed preclinical assets with a better target validation. Companies are also striving to improve their operational processes with a view to speeding up the time it takes to apply for a first-in-human study. But if clinical development fails to keep pace, the benefits to patients of faster drug discovery will inevitably be delayed, and delivering on the promise of AI-enabled acceleration may flounder.
While the RCT will remain a central pillar of clinical development, the tide is changing. Regulators have recently issued guidance on appropriate use of real-world data (RWD).4 The healthcare data ecosystem is booming, leveraging privacy-respecting technology to make patient-level data safely available for research. And evidence generation from multiple data sources is now possible using causal machine learning, which aims to distinguish correlation from causation via a combination of biostatistics with machine learning (ML) and, increasingly, gen AI and foundational models.
Despite this level of promise, only a handful of established companies are deploying AI and data-driven approaches systematically in their clinical development. The focus so far has been on improving operational excellence and increasing acceleration rather than helping to inform trial design strategy. The remainder of this white paper dives deeper into the context, gives tangible example use cases and associated impact, and identifies challenges companies face when adopting AI for clinical development. Rather than staying at a high level, it aims to bring the topic to life through relevant details and case examples.
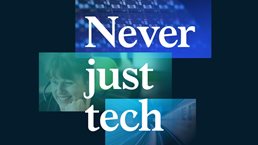
Creating value beyond the hype
Expanding adoption of AI and RWD for clinical development
Today AI can draw upon growing volumes of RWD. Electronic health records and claims data from certain geographies are widely available, and novel data sources—including biobanks, data omics panels, population-wide genomic studies, patient registries, and imaging and digital pathology—are increasing in number and diversity. All these sources reflect a notable change in patients’ ability to share their data for the purpose of advancing research and treatments in privacy-respecting ways, including technology that enables the training of ML models in a manner that respects patients’ privacy.
In addition, new tools can systematically capture knowledge from unstructured data. Large language models (for example, BioGPT) are able to convert unstructured physician notes into high-quality structured data. Similarly, these models can search the vast corpus of published literature and identify connections between biological entities on a large scale, generating high-quality input for knowledge graphs that better represent the totality of available evidence on a given indication across domains, including genes, targets, proteins, pathways, and phenotypes.
Most pharma companies using AI and RWD in clinical development tend to do so only in isolated use cases, and they rarely deploy both in combination. For example, they might use machine learning to select trial sites or to predict patient enrollment rates. They might use RWD to measure disease prevalence—the size of an eligible population—to understand the natural course of a disease among untreated patients, or to construct an external control arm for regulatory purposes. While these use cases deliver value, a great deal more is now possible. Similarly, while the level of funding and investment going into AI-enabled drug discovery companies has tripled in the last five years, that same trend is not true for equivalent companies in the clinical development space.
Four use cases that demonstrate the potential of AI
Companies that are deploying AI combined with RWD are seeing impactful results. While RWD can be valuable supporting evidence in health authority submission packages, the most successful companies focus their use of AI and RWD to make better and more informed decisions to support the success of clinical development programs in every step, from asset and portfolio strategy to protocol and trial design:
- At the stage of defining an asset strategy, AI and RWD can reveal which indications are most promising indications to pursue for novel assets. Several leading biopharma companies identified multiple new indications for existing assets in this way, and an early-stage biotech used AI and RWD to assess whether to shift its indication selection strategy regarding a novel asset.
- AI and RWD can support decisions about the target patient population of a clinical trial, with subgroup discovery, refine trial eligibility criteria and help remove patients that are highly unlikely to benefit from the treatment, and shorten the length of trials. One early-stage biotech could better characterize “super-progressors” (that is, patients whose disease was likely to progress faster within the time frame of a clinical trial) and design a trial with similar expected benefits in a faster time frame.
- For decisions about portfolio strategy, AI and RWD can help companies identify the right combination of drugs for an indication or for the right patients. One biopharma company leveraged a prospective observational data set to generate evidence supportive of earlier positioning of its third-line treatment. Another was able to identify “super-responders” for several drugs in its portfolio—insights that helped the company position its assets optimally in a crowded indication.
- At the step of selecting and optimizing the end points of a clinical trial, AI and RWD can help a company identify patient attributes that closely track the primary end point over time. One biopharma company replaced a rare disease’s existing end point, which was an infrequent event, with end points that occurred with greater frequency or could be measured with blood tests. This cut the length of trials by 15 to 30 percent.
The following discussion looks in greater depth at four detailed use cases, illustrating the wealth of information that AI can unlock.
Indication selection for asset strategy
Selecting which indications to target with a specific molecule is one of the most important decisions a biopharma company makes. This decision is often informed by a combination of input from key opinion leaders, literature reviews, omics analysis (for example, genome-wide association studies), RCT data, and competitor decisions. Such decisions rarely are fully data driven, typically prove hard to integrate, and cover only part of the available evidence base, resulting in a subjective and suboptimal synthesis. In contrast, strategies informed by RWD and AI are objective and comprehensive in their use of multiple data sets and foundational models built on clinical data continually redefining the art of the possible.
For already-approved therapies, companies can use RWD to understand the therapies’ likely efficacy on alternative indications. One approach is examining the outcomes of patients who were prescribed the drug on a spontaneous basis by their physician; another is determining a drug’s average effect on patients exposed to the treatment because of incidental comorbidities. AI techniques can then extrapolate any findings to a set of patients whose characteristics may be distributed differently, by leveraging observed correlations between patient characteristics and outcomes.
Where companies are looking to expand the indication of an approved asset or identify for a novel asset, RWD and AI can estimate the biological proximity of one indication to another from a patient and clinical perspective—that is, whether the patient experiences similar symptoms, comorbidities, lab characteristics, and treatment journeys. Foundational models that treat medical events as words and patient medical histories as documents can uncover the semantic similarity of different events, including diagnoses. Each indication, from what is likely to be a sizable list of those with biological proximity, is scored according to its similarity to one or more anchor references—perhaps the indication for which the asset was originally approved or, in the case of a novel asset, one for which there is strong preclinical evidence of efficacy of the asset’s mechanism of action (MoA).
The scoring can also incorporate information from molecular knowledge graphs that show new connections—for example, between entities such as proteins or human biological pathways that have already been identified in literature or public data. Exhibit 1 shows a potential outcome of this approach: it can reveal the indications most strongly connected to each of the reference indications, serving as an anchor into the existing evidence base. The indications with the most connections to these references are further prioritized by unmet medical need, strategic fit, and technical feasibility, resulting in an evidence-based indication selection.
These analytical approaches can identify novel indications that can be rapidly validated via in vitro or animal models, can increase the confidence in selecting indications with a high probability of success, and can derisk resource allocation accordingly. The analysis provides a clearer and more holistic evidence base for investors, shareholders, and R&D leaders, and it can reduce the opportunity cost of blind alleys by helping new treatments reach patients faster.
Subgroup discovery for trial design
Once an asset has been matched with an indication, pharmaceutical companies pursue an all-comer clinical development and trial design strategy—that is, one that includes all patients except those deemed high-risk based on input from key opinion leaders and conventional wisdom.5 As a result, patients unlikely to respond to a treatment may be included in the trial. A notable exception to this method is in precision oncology, where researchers use specific biomarkers discovered preclinically (such as genetic mutations) to stratify patients according to their probability of progression or to predict a patient’s response to different treatments. This approach is revolutionizing oncology treatments, but even it can identify only a fraction of possible patient subpopulations.
In contrast, AI and omics-rich RWD can examine thousands of genetic and/or phenotypic attributes to pinpoint the combinations most likely to influence prognostic or predictive scores, explain response heterogeneity, and improve a trial’s technical probability of success. Even in situations where the patient cohorts are modestly sized, foundational models trained on broad RWD can be used as a starting point for more bespoke modeling at the indication level.
One large biopharmaceutical company used RWD to remove likely nonresponders from a trial and to reduce its expected duration by between 5 and 10 percent without compromising its probability of success, which would allow the treatment to reach patients faster. Another company leveraged hospital episode RWD to identify super-responders to its comparator arm for an asset in Phase I, ensuring the trial was designed in a manner that focused on patients without access to effective treatments.
AI for subgroup discovery can also directly support the strategic trade-off between the size of the eligible population and the level of the treatment effect in a smaller population, in the form of an efficient frontier (Exhibit 2). The different subpopulations that are marked as blue on the exhibit are those for which it is impossible to simultaneously increase their breadth without reducing the drug’s expected effect. This approach widens the overlap between patients with access to this new treatment and patients who are most likely to benefit significantly from it.
Subgroup discovery and comparative efficacy for portfolio optimization
Not only can AI be used to discover subgroups to inform the design of a trial, it also can contribute to the wider portfolio strategy. The product pipelines and portfolios of many biopharmaceutical companies contain multiple assets aimed to treat a similar set of patients, often because companies lack sufficient evidence to inform the portfolio strategy differently, especially for new MoAs. However, the systematic analysis of all available data sources can predict the response of different patient subgroups (or disease endotypes), which could help companies hone their portfolio strategy—in some cases, resulting in a double-digit percentage improvement in net present value. This strategic stance can support targeted trials that produce the type of precision evidence that clinicians need to make the best possible treatment decisions for their patients amid a proliferation of novel assets.
In addition, several analytical approaches and data sources can be combined to determine the efficacy of novel MoAs in the portfolio relative to each other or to approved treatments of different patient subgroups. Enriching RWD with data from molecular knowledge graphs can enable foundational-model-powered representations of treatments that represent their associated biological pathways. From this, the company can estimate the efficacy of a novel treatment by observing the outcomes of approved treatments that share similar biological action mechanisms with the novel treatment. This exercise can be repeated across multiple disease endotypes or patient subgroups, identified through a combination of patient-level attributes (Exhibit 3).
End point optimization in clinical development
In many trials, there is no single, established end point. In others, it can be hard to know which of several would best measure the intended action of the drug. Some end points may take a long time to manifest, leading to extended development timelines. Others may detect progression that is more reliable for some types of patients than for others or that may be particularly invasive, increasing the trial’s burden on patients.
AI used on rich, patient-level longitudinal data sets can be deployed to identify patient attributes that closely track the primary end point over time. Such an application can control multiple confounding factors to ensure the association persists in future trials where the patient population may differ, thereby establishing potential novel end points.
Here, X-rays, CT and MRI scans, and other imaging techniques are often valuable sources of data for monitoring biomarkers in a noninvasive manner (as is the case with using MRIs to help detect Alzheimer’s disease6). Foundational models using medical imaging are increasingly able to extract imaging biomarkers curated by human expertise from raw images at scale. They can even discover altogether novel, deeply hidden visual signatures of disease activity and severity with better predictive characteristics.
In all cases, the association between novel end points and desired patient outcomes such as survival or quality of life must persist even under treatment. The clinical data and the information present in RWD must be combined with a biological understanding of the mechanisms affected by the considered treatment.
Tackling the organizational challenges
Inevitably, supplementing a century-long, RCT-dependent approach to clinical development with a new, analytics-driven one using novel data poses organizational challenges. Here are some ways to tackle them.
Embed AI into clinical development
The governance processes and incentive structures in place in most biopharmaceutical companies encourage default solutions in clinical development at the asset team level and beyond. One consequence is the prevalence of all-comer trials, which target the broadest possible population without leveraging evidence about nonresponders and super-responders—a method that can increase trials’ duration and decrease their probability of success, resulting in longer waits for patients who would benefit from the treatment.
R&D leaders can help change that culture by encouraging the use of analytical methods to integrate all sources of available evidence when making key decisions. Individual incentives can help. A stronger step is to strengthen the development governance model to require that all key decisions be supported by AI.
Develop internal capabilities and talent
External providers can supply off-the-shelf AI solutions to support some use cases. But with so many new solutions emerging, no provider can yet support them all. Even if they could, companies would be unwise to become dependent upon them for critical strategic decisions. Providers with such rich intellectual property might eventually become competitors.
Biopharmaceutical companies could therefore consider building their own AI capabilities and products. However, significant investment is needed to acquire the relevant data sets; build up the necessary data science, data engineering, and business translator expertise; and establish agile product development processes that ensure use cases deliver business value in a timely and cost-efficient manner.
Companies will also have to work hard to attract and retain top data scientists and data engineers, as the biopharmaceutical industry is not typically their first choice. Measures that can help include offering competitive remuneration packages, building an innovative employer brand, and locating in key talent hubs. So, too, can a focus on the industry’s value proposition—offering employees the chance to help people lead longer and healthier lives.
Develop a targeted data strategy
With so much data becoming available, companies should consider formulating a detailed and iterative data strategy for each disease area. The purchase of certain commercial data sets, such as electronic health records, claims, and sometimes omics, can be relatively straightforward, even in therapeutic areas such as oncology, where biomarker-rich data are required. But other types of data sets—registries, biobanks, and clinical trials from other pharma companies—can involve complex negotiations and contracts, and companies will have to partner with a range of different data providers.
In addition, each data set is likely to be structured differently, with varying levels of quality and completeness. This means companies will likely need data curation and analytical capabilities for tasks such as combining different data sets to plug gaps in evidence and meeting regulatory expectations for the use of real-world data.
Build scalable products
AI’s power lies in its application across the clinical development portfolio, not restricted to isolated use cases. Achieving this kind of scale can be hard, due to limitations of the code base, data platforms, the company’s data engineering capabilities, and the analytical stack needed to collect, combine, and analyze data. As a result, pilots often remain just that—never reused or deployed against other assets in development.
To counter this, analytical use cases should be built as products, meaning solutions built in a manner that makes them easy to reuse and scale for different use cases, even at the proof-of-concept phase. The code base should be well annotated, for example, and deploy common, reusable analytical components that are either developed internally, open-source, or bought off the shelf. Shared ML development standards and coding frameworks can help by speeding up development work across different use cases because data scientists and engineers are familiar with them. Companies also need a mature and flexible ML operations stack—that is, the operational components needed to streamline the process of taking ML models to production and maintaining and monitoring them.
By harnessing novel data and the power of AI, biopharmaceutical companies can move beyond RCTs to vastly improve clinical development. The benefits accrue at each stage of the development process, from formulating asset strategy to designing the protocol and trial planning. For patients, the results can speed up access to new treatments, thanks to an accelerated development timeline, and make treatments more likely to elicit the strongest response.
High-impact solutions are already being implemented, and more will follow. Companies can benefit from beginning to build the capabilities they need and scaling them across the portfolio and their assets’ life cycles. The clinical development and trial design system is ripe for change, and the value to patients will be vast.