The pharmaceutical industry continues to pursue the discovery of medicines for cancer patients with unprecedented rigor. Over the past several years, three major trends have reflected this pursuit. They include the growth of the industry pipeline of cancer-drug candidates, the evolution of the clinical-trial landscape, and the explosion of available clinical and real-world data.
Stay current on your favorite topics
The cancer-drug pipeline and associated investment has been growing substantially. We estimate that currently more than $50 billion is invested in oncology R&D annually. With this investment, the number of active compounds in oncology R&D has quadrupled since 1996 and nearly doubled since 2008 alone. Today, oncology makes up nearly 40 percent of the global clinical pipeline (Exhibit 1).
Not surprisingly, immuno-oncology (IO) has been a main driver of the growth of the industry pipeline. According to our own research, more than 40 percent of the annual R&D investment in oncology is directed to the exploration of immune checkpoint inhibitors both in monotherapy and combination programs. Between 2015 and 2016 alone, we witnessed growth of 70 percent in the number of unique hypotheses or experiments tested in combination with PD-(L)1 or CTLA-4 across 85 mechanisms of actions, further underscoring the dynamic nature of this field (Exhibit 2). At the moment, there are over 1,500 IO clinical trials in the industry pipeline in monotherapy and combination testing across 183 unique therapeutic mechanisms.
The clinical-trial landscape has also evolved significantly. While the number of trials is three- to fivefold higher than the number of active compounds, the rate of increase in the number of trials relative to the increase in number of compounds has decreased from 5:1 to 3:1 in recent years. While there are many factors playing into this trend, a noticeable shift toward accelerated approvals and breakthrough designations, as well as changes in the clinical-development paradigm toward adaptive trial designs and combined phases, has played a role. This is highlighted by the fact that more than 40 percent of US Food and Drug Administration (FDA) pivotal trials for novel anticancer agents between 2013 and 2015 were Phase I or II (Exhibit 3).
These statistics highlight the evolution of regulatory data packages, with increased scrutiny of postmarketing data-generation commitments. Importantly, breakthrough-designated trials have also recruited one and one-half to two times faster, further adding to the speed with which many novel cancer therapeutics are being developed today. Pioneering examples of these innovative development paradigms include, but are not limited to, pembrolizumab (KEYNOTE-001 supported the initial registration in both NSCLC and melanoma as a Phase I trial), osimertinib (followed the accelerated-approval path as a breakthrough therapy with a single-arm design), and LOXO-101 (announced plans to submit data from the ongoing NAVIGATE Phase II trial for accelerated approval, enrolling patients with NTRK-fusion proteins across 14 metastatic solid tumors). While there are successes and failures, the pursuit of approaches independent of tumor site is becoming more prominent. Examples include denosumab approval across primary tumors based on bone metastases selection in 2010, the first FDA tissue/site-agnostic approval for pembrolizumab in MSI-H or dMMR cancers in 2017, and the NCI-MATCH study (a 30-arm trial of treating cancers according to molecular abnormality) (Exhibit 4).
The increase in clinical activity is interesting in the broader context of the explosion of data generation. Nearly 90 percent of the data ever created originated in the past two years. In healthcare alone, data is rapidly proliferating from different sources—from patients (for example, vital signs, behavioral data, patient-reported outcomes), providers (for example, electronic medical records, clinical notes, medical imaging), pharmaceutical companies (for example, drug discovery, clinical trials, genomics), or payors (for example, health claims, billing, population health data).This presents significant challenges in how to structure, integrate, and interpret this data for relevant insights to inform care decisions as well as R&D and to complement or even replace wet-laboratory innovation in the long term. Within the context of rapidly increasing healthcare data generation, oncology is once more at the forefront. More than 13 million electronic medical records exist for cancer patients in the United States alone. The next-generation sequencing market is projected to grow at an annual rate of 20 percent or more from 2017 to 2022. Additionally, payor spend on data and analytics capabilities is growing at a 20 percent clip year on year (Exhibit 5).
To maximize the opportunity from these rapid changes and thus bring the right therapies to the right patients faster, the whole cancer community must embrace five major imperatives:
- Improve patient access and recruitment to clinical research.
- Ensure real-world impact of new medicines and faster approvals.
- Optimize data capture, standards, and integration.
- Further push the clinical-development model.
- Evolve the pharmaceutical clinical-development operating model.
Improve patient access to clinical research
The first step to more-comprehensive clinical-data generation and acceleration of clinical research must address today’s recruitment challenge. For example, in the United States, only about 3 to 5 percent of patients participate in clinical trials. The resulting poor accrual is the underlying cause of 33 percent of the terminations observed in oncology clinical trials, the largest segment by far. In breast cancer, for example, 80 percent of the incident patients would have been required to complete recruitment for open clinical studies in 2016, not considering any exclusion or inclusion criteria.
The reasons for this accrual challenge are manifold. Physicians, especially in the community setting, are not always aware of open trials and often do not have adequate incentives to refer patients to clinical studies. Patients often misperceive the nature of clinical studies in cancer. For example, they often do not want to join a clinical study for fear of being randomized to the control arm and expecting to receive placebo. In addition, approximately 80 percent of cancer patients are diagnosed in the community setting and require logistical support to reach the nearest trial centers. And this is all while clinical trials are becoming more complex, with the number of total procedures having doubled since 2000. Increasingly demanding inclusion and exclusion criteria have led to an uptick in screen-failure rates, and after such an experience, patients often do not embark on participating in clinical studies again.
Would you like to learn more about our Life Sciences Practice?
Finding a solution to these issues is by no means trivial. The search would need to start with increased physician awareness, alignment of incentives, and patient education. In addition, a system by which trials are conducted in a more decentralized fashion in the community while not losing quality would ideally be established. This system would include leveraging community nurses for select procedures and digital approaches for remote consent and other steps in the trial. Finally, advanced-analytics approaches combining multiple data sources would help improve patient recruitment through more-effective identification of patients, especially those with rare tumor types.
Ensure real-world impact of new medicines
With the advent of targeted therapies in the late 1990s and early 2000s as well as the recent wave of IO-based therapies, we have seen a dramatic improvement in clinical outcome as measured through progression-free survival (PFS), overall survival (OS), or surrogate endpoints. Some solid tumor examples include prostate, breast, and melanoma. Additionally, in select hematologic indications, therapies like imatinib and ibrutinib have turned treatment of cancer almost into treatment of a chronic disease.
On the other hand, over the past two years, two studies were published that have questioned the long-term impact of modern medicines in cancer on real-world survival and quality-of-life outcome. These studies, which examined drugs approved by the FDA and European Medicines Agency (EMA), found that there was limited follow-up after approval with a subset of agents and that other agents did not show survival or quality-of-life improvement in the real-world setting. The authors highlight that there are clear limitations to these studies, including data comparability, differences in trial design and comparator, and selection of studies for analytical interpretation. However, they do illustrate the complexity in comparing data from randomized controlled clinical trials and real-world settings and underline the need for continued monitoring of approved medicines.
To address this issue, the industry is continuing to invest in different avenues of data generation after therapy approval. Given the increase in accelerated approvals over the past several years and the differences in PFS and OS observed in multiple studies with PD-(L)1 checkpoint inhibitors, it will be critical to continue to monitor closely not only the correlation between surrogate and survival endpoints in controlled settings but also data generated in the clinic and the real-world setting.
Optimize data capture, standards, and integration
A cancer patient generates over 100 million data points daily. Numerous efforts, both in the private and public setting, are attempting to integrate disparate data sets, including electronic medical records, pathology and genomic data, and clinical-trial data to generate insights that could lead to better clinical care and more-effective R&D.
Two key areas that need to be addressed are standardization and sharing of data. Multiple data standards, including HL7, ICD-10, and others, have been developed over the years. However, each standard has faced its unique challenges to broad adoption, including lack of specificity in some instances (for example, ICD-10), limited standardization within the specific envelope itself (for example, HL-7), and focus on specific subsets of data (for example, TransCelerate for clinical data). The Oncology Research Information Exchange Network (ORIEN) and Project GENIE (Genomics Evidence Neoplasia Information Exchange) of the American Association for Cancer Research (AACR) are starting to combine and standardize across larger sets of data, including cancer genomic and clinical outcome data as well as electronic health records, pathology, and clinical data, respectively. However, these data sets more closely represent data from academic medical centers, and more health systems need to join.
Data sharing is hampered by a multitude of factors, including adequate financial and nonfinancial incentives, the type of data being shared (limited sharing of negative data from nonclinical research), governance of sharing and patient control (opt-in, directive, or other measures), metrics for sharing (data points posted versus reused), a sufficient infrastructure to create insights, and many more.
Solving the data issue will require technical as well as governance aspects. Learning health systems, systems in which health information generated from patients within that system is continuously analyzed to improve knowledge that will be transferred to patient care, could be one potential solution to address data standardization without creating yet another standard. Project TRANSFoRm is such an example and has shown proof of concept in a set of three use cases showing that, using the same platform, linked cross-country clinical and genomic data could be used across several countries, diagnostic decision support can be established, and trials can be designed prospectively across international sites with different data systems. Data sharing, in addition to requiring an adequate infrastructure and financial incentives to capture and share data, will rely even more on policy and governance solutions. These solutions need to provide scientists with incentive to capture and share data during (not just after) nonclinical research, establish adequate metrics to ensure high quality of shared data (for example, measured by reuse), and develop approaches for patients to opt into or direct data sharing to different degrees, to name a few goals.
Further push the clinical-development model
Over the past several years, the clinical-development paradigm in oncology has evolved significantly, in part due to alternative regulatory pathways offered by the FDA and EMA. The community has moved away from the classic three-phased design to approvals based on data found as early as in Phase Ib open-label studies as well as basket trials (for example, pembrolizumab MSI-high). Multiple other mechanistic studies are ongoing both in the private sector (for example, the LOXO-101 NAVIGATE program) and the public sector (for example, NCI-MATCH).
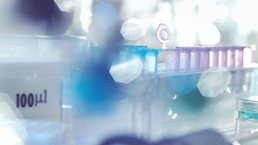
The next wave of innovation in oncology
While this trend lends significant additional agility to the clinical-development paradigm, select questions remain. Survival times as well as therapeutic choices after clinical trials are increasing. This will make it increasingly difficult to improve OS (versus PFS) in select indications or even to conduct clinical studies due to time and cost considerations. While more diverse surrogate endpoints would address this problem in part, their correlation with OS remains a point of debate. Furthermore, and especially evident in the recent wave of PD-(L)1 clinical programs, there is a certain level of redundancy with control arms for studies of similar drugs in the same tumor type and line.
While it is essential to retain the scientific rigor behind clinical research, potential considerations to address these topics could include increased use of adaptive designs and master protocols as well as a broader assessment of additional surrogate endpoints for earlier-stage disease and indications with longer survival times. Additional clinical testing to validate these endpoints or additional control arms more reflective of a real-world-like setting have been debated. However, safety and ethical aspects must be considered first and foremost. Last, synthetic control arms might offer potential for more-efficient clinical programs but will require significant further exploration before having impact.
Evolve the pharmaceutical clinical-development operating model
For a long time, drug development required a somewhat stable operating model for manufacturers. The broader move toward translational medicine started to change this need, especially in oncology, given the low predictability of preclinical and even early-stage clinical data for later-stage significance in efficacy and safety. The change in the development paradigm, increased exploration of combination therapies, and the power of advanced analytics in drug development is taking this change to another S-curve.
Clinical-trial design must take into account potential opportunities for accelerated approval paths, trading additional post-approval commitments and, potentially, smaller patient populations with faster market entry. A deep understanding and translation of the disease biology is necessary for these considerations, as illustrated in the AURA3 program for osimertinib.
Clinical-operations teams must become more agile, changing compounds more frequently with faster development paths. In addition, they will increasingly need to work across company boundaries in the pursuit of combination therapies and development partnerships. And last, they must harness the power of advanced-analytics approaches to drive effective and efficient clinical development through patient finding, improved site selection, in-trial monitoring, and other approaches. In our experience, these approaches can liberate up to 15 to 20 percent clinical capacity that can be redirected to additional development programs to develop more medicines for cancer patients globally.
Embracing these five imperatives and addressing the disruption and opportunities they bring to drug development will permit the community to develop more medicines faster and in a more directed fashion to improve the lives of cancer patients around the world.