When it comes to dynamic pricing, Amazon is still the retailer to beat. Other retailers continue to marvel at—and attempt to emulate—the e-commerce giant’s ability to rapidly and frequently change prices on millions of items. Amazon continually burnishes its low-price reputation by undercutting competitors on top-selling, high-visibility products, while protecting margins by charging more for less price-sensitive items. Indeed, the success of Amazon and a handful of other leading online players has made clear that dynamic pricing is a critical capability for competing in e-commerce, omnichannel, and even brick-and-mortar retail to drive revenue and margin growth.
Stay current on your favorite topics
But as retailers have begun to invest in dynamic-pricing solutions—whether off-the-shelf or custom-built by third-party providers—they’ve often run into the “black box” problem: none of the end users actually understand the math or logic behind the algorithms. The tools somehow crunch data and spit out pricing recommendations, which are sometimes much higher or lower than current retail prices. The pricing staff thus ends up rejecting them entirely because they don’t trust the recommendations.
Overcoming that trust barrier requires customizing every part of the solution, including the implementation. In our experience, a dynamic-pricing solution should be optimized for use by category managers and pricing managers. These end users should be involved in developing, refining, and rolling out the tool and be able to override the pricing recommendations. Only when this happens can businesses expect to capture significant and sustained impact—typically, sales growth of 2 to 5 percent and increases of 5 to 10 percent in margins, along with higher levels of customer satisfaction through improved price perception on the most competitive items.
Five modules of dynamic pricing
Dynamic pricing plays a crucial role in boosting both consumer price perception and retailer profitability. Many retailers sell about one-fifth of their assortment at very low prices to shape their price image and remain competitive. These key value items (KVIs) are usually top sellers, traffic generators, or highly-searched SKUs whose prices consumers tend to remember. Key-value categories can account for up to 80 percent of an average retailer’s revenue but only half of its profit. The retailer therefore needs to make up margins in the rest of the assortment—the “long tail” items. However, identifying KVIs isn’t as easy as it sounds, and setting and validating prices for long-tail items is difficult precisely because of the sparse historical data on such items.
Dynamic-pricing solutions help retailers overcome both of these challenges. Generally speaking, a robust dynamic-pricing solution should consist of five modules, all working in parallel to generate price recommendations for every SKU in the assortment (Exhibit 1).
- The long-tail module helps a retailer set the introductory price for new or long-tail items through intelligent product matching—that is, the module determines which data-rich products are comparable to new items (which have no history) or long-tail items (which, as mentioned, have limited historical data).
- The elasticity module uses time-series methods and big data analytics to calculate how a product’s price affects demand, accounting for a wide variety of factors including seasonality, cannibalization, and competitive moves.
- The KVI module estimates how much each product affects consumer price perception, using actual market data rather than consumer surveys. This enables the module to automatically detect changes as to which items consumers perceive as KVIs.
- The competitive-response module recommends price adjustments based on competitor prices updated in real time.
- The omnichannel module coordinates prices among the retailer’s offline and online channels.
While a best-in-class solution includes all five modules, retailers can often begin with only the KVI and competitive-response modules. These help retailers nimbly respond to competitive moves on key items. Retailers can then add the rest of the modules over time.
Developing a world-class dynamic-pricing solution starts with a thorough understanding of the retailer’s business context and objectives, and then translating those into mathematical “recipes” that can be executed repeatedly. Careful selection of the inputs, as well as the sophistication of the underlying analytics, will largely determine the accuracy of each module’s calculations. The tool needs to be flexible and adaptable enough for businesses to customize the inputs and features based on their particular objectives and existing capabilities, which greatly increases confidence in the outputs. And of course, whether category managers and pricing managers will ultimately use the solution in their daily work depends partly on how intuitive the interface is and how easily it integrates into the retailer’s existing systems and work flows.
To build a case for dynamic pricing, a retailer could first quantify the potential of introducing dynamic pricing into the organization—for instance, by systematically comparing the retailer’s price levels to those of its chief competitors, assessing how frequently competitors change their prices, and studying how competitors react to the retailer’s own price changes. The findings from such an exercise will almost certainly spur the retailer to take action on dynamic pricing.
The next logical step would be to conduct a pilot in a handful of categories for concept design and testing. Done right, the pilot—and the subsequent rollout of dynamic pricing across all product categories—will yield meaningful improvements in revenue, profit, and customer price perception.
Would you like to learn more about our Marketing & Sales Practice?
Customizing the modules: Three case examples
The following examples illustrate how retailers can tailor dynamic-pricing modules to their particular business needs and objectives. In each case, the retailer collected massive amounts of granular data, used advanced analytics, and made sure that category managers and pricing managers participated in developing and testing the solution.
A US retailer’s long-tail module
A US-based general retailer with more than two million SKUs in its assortment had two high-priority business objectives that required frequent trade-offs: to maximize absolute revenues and to increase productivity. The objective functions of the algorithms in each of its modules therefore had to be adjusted accordingly—a level of customization that wouldn’t have been feasible with an off-the-shelf solution.
To build its long-tail module, the retailer assembled a rich set of data, including daily sales data for its 100,000 top-selling SKUs, competitor prices (gathered via web scraping) for those SKUs, data on customer browsing and purchasing behavior, product attributes and descriptions, and online metrics such as impressions and search rankings. With algorithm-design experts and analysts working alongside category managers, the team codified a set of product-association rules specific to the retailer, using factor analysis to assign “attribute similarity scores” that indicated which products its customers find comparable. The retailer set simple ground rules for its product groupings—for example, a grouping should have minimum sales of 20 units a week, or all products in a grouping should be in the same life-cycle stage. The algorithms also helped the retailer understand which of its product prices should move in concert so as to avoid cannibalization effects.
In just eight weeks, the team built working prototypes of both the long-tail module and a competitive-response module. Both were designed and tested with pricing managers to integrate simply into the retailer’s regular pricing processes and cadence. The impact: up to 3 percent increases in both revenue and margins in the pilot categories.
An Asian online retailer’s elasticity module
A leading Asian e-commerce player aspired to develop an item-level pricing strategy that could optimize for both profit and gross merchandise value (GMV). To that end, the company knew it needed to be able not only to change prices frequently, but also to take many more factors into consideration when setting or changing prices.
As part of a broader dynamic-pricing effort, the company built an elasticity module. At its core was a multifactor algorithm that drew on data from approximately ten terabytes of the retailer’s transaction records. Data for each product included the price of the product, the price of a viable substitute product, promotions, inventory levels, seasonality, and estimates of competitors’ sales volumes—creating a custom module unique to the retailer’s available data and pricing strategy. The module then generated pricing recommendations, taking into account both of the retailer’s business objectives.
Recommendations were displayed on an easy-to-read dashboard that category managers helped design and test. Category managers, who on any given day would be weighing other important trade-offs with regard to, say, a product’s growth potential or expected additional inventory, could then accept or reject the pricing recommendations. The retailer felt strongly that category managers should have the final word on pricing decisions.
After only a few months of using the module, the company saw a 10 percent rise in gross margin and a 3 percent improvement in GMV in the pilot categories.
A European nonfood retailer’s KVI module
Looking to stand out from competitors, a leading European nonfood retailer sought to identify and prioritize the KVIs in its assortment. It built a tailored KVI module that could statistically score each item’s importance to consumer price perception on a scale of 0 to 100. The module generated this “KVI index” by analyzing granular internal and external data, including shipping costs, return rates, search volume, number of competitors carrying the product, and competitor pricing. It also identified which other retailers were the true competitors for that specific item. Importantly, the module was flexible enough that category managers could adjust the weighting of each parameter.
The module defined the price range, or the upper and lower price bounds, for each item (Exhibit 2). Each product’s exact price position within the range would then be based on its score in the KVI index.
But a KVI index to help set the base price was only the first step. Via the competitor-matching module, the retailer also programmed into its dynamic-pricing solution a set of business rules that would trigger pricing changes. For instance, if inventory levels for a certain SKU were high or if a competitor reduced the price of that SKU, the solution might recommend a price drop for the SKU. These rules would all feed into the solution’s recommended price, which the category manager could either accept or reject based on additional indicators and considerations.
At the end of a three-month pilot, the retailer saw a 4.7 percent improvement in earnings before income and taxes in the pilot categories and identified a 3 percent improvement potential in overall return on sales. And it had a trusted solution that category managers could incorporate into their work flows.
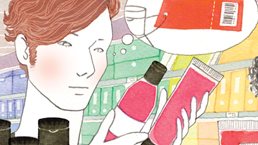
How retailers can improve price perception—profitably
Building dynamic-pricing capabilities
In each of these examples, the retailer custom-built the algorithms and invested time and effort to ensure that the tool was adopted by end users. A test-and-learn approach, beginning with a pilot in a few categories, will help produce a solution that builds trust and yields market-proven, statistically sound results. Just as important, the testing process can pinpoint how best to embed the solution into end users’ existing work flows.
Each of the three retailers invested in detailed documentation and thorough training to strengthen the organization’s skill base and capabilities in dynamic pricing. One of the retailers even established a certification program for dynamic pricing, creating a pipeline of employees who would be qualified to manage and continually improve the pricing process.
In light of the explosive growth of e-commerce, dynamic pricing is fast becoming a must-have capability to drive growth while sustaining margins. By understanding how to move quickly and customize solutions, retailers can build this capability into a significant competitive advantage.