Semiconductor R&D budgets are growing by about 6 percent annually, and the drivers behind the soaring costs are easy to pinpoint. On the technology side, Moore’s law is getting harder to maintain, while business models have increasingly shifted toward systems and solutions that require more complicated development processes. Organizationally, large internal software groups are now the norm as engineers grapple with increased complexity, especially in coding, testing, and verification. Given these developments, it’s no surprise that semiconductor companies rank above those in other S&P 500 industries in terms of R&D expenditures as a percentage of overall sales (Exhibit 1).
Semiconductor companies have already embarked on ambitious programs to decrease costs and boost productivity through advanced data analytics. But most of their efforts have focused on streamlining basic engineering tasks, such as chip design or failure analysis, rather than on improving management activities. Without automated tools to sort data, engineering managers have difficulty obtaining fresh insights and identifying patterns that might lead to better strategies. By default, they rely on the same management systems and performance metrics that they’ve used for years.
With costs continuing to rise, it’s time for semiconductor companies to reexamine how advanced data analytics could benefit engineering management. Although they could find some inspiration by looking at data-driven strategies in similar industries, we believe they also have much to learn from elite sports. The greatest lessons may come from baseball, where advanced analytics came into vogue after data scientists turned California’s struggling Oakland Athletics into a top competitor, in 2002. The now-ubiquitous use of data science to select baseball players and design teams also contributed to the Chicago Cubs’ historic World Series win in 2016.
Stay current on your favorite topics
How can semiconductor management learn from the advanced analytics used in baseball?
You don’t need to understand baseball to appreciate the Oakland Athletics’ transformation, which Michael Lewis described in his 2003 book, Moneyball: The Art of Winning an Unfair Game. The force behind the change was Billy Beane, the team’s manager, who had a much smaller recruitment budget than major-league powerhouses, such as the New York Yankees. Since Beane couldn’t afford to recruit stars with the best batting averages or other well-known performance measures, his statisticians investigated whether other metrics were equally or more effective in identifying talented players. One question was at the core of every analysis: What are the true drivers of winning teams?
Would you like to learn more about our Semiconductors Practice?
To handle the massive volumes of baseball statistics, the team’s analysts relied on tools that incorporated pattern recognition and machine learning. They could therefore sift through multidimensional data sets with greater precision and accuracy than a manual process would provide. Their research revealed that the teams whose players scored high on common metrics were not always the most successful. On the contrary, the statisticians discovered that certain overlooked metrics, such as on-base percentage combined with slugging percentage, had the highest correlation with a baseball club’s success.1
Armed with these facts, Beane recruited players who scored best on these offbeat metrics and could be acquired for reasonable salaries when he assembled the 2002 team. His strategy paid off that season: the Oakland Athletics achieved a club record 103 wins, on par with the Yankees but with a much smaller budget.
Although the Oakland Athletics stopped short of a championship that year, the analytics-led approach has taken Major League Baseball by storm. It paid off notably in 2004, when Theo Epstein, then general manager of the Boston Red Sox, used data analytics to help his team win its first World Series in 86 years. After Epstein moved to the Chicago Cubs, in 2011, he again made data analytics part of his core strategy. In 2016, after a five-year transformation, the Cubs won the World Series for the first time since 1908.
Similar strategies have been applied in sports as diverse as European football (analyses to predict the likelihood of injury) and basketball (finding the best pairs of players, not just the best players). It may seem like a leap, but semiconductor managers might also benefit from using advanced analytics to determine which metrics—including unlikely ones—are correlated with success in R&D for both individuals and teams.
How do advanced analytics work in semiconductor engineering?
Over the past two years, several organizations—including an electronics manufacturer; pharmaceutical, oil and gas, and high-tech companies; and an automotive OEM—have taken a cue from Billy Beane to improve management techniques in complex engineering environments. The “Moneyball for engineers” approach, which relies on pattern recognition and machine learning, has uncovered counterintuitive insights, typically delivering productivity gains of 20 percent or more for engineering groups. With some fine-tuning, it is equally effective in all industries, including semiconductors. For more information on the origin of this approach, see the sidebar, “Lessons from Formula One racing.”
You can’t use batting averages: Where do semiconductor managers start when applying advanced analytics to engineering?
In baseball, statisticians have been compiling detailed data for many decades, and the fact base is easily accessible for anyone who wants to conduct an analysis. But most companies can’t consult existing repositories that contain information on individual engineer performance, or even comprehensive data on project performance. Instead, they have bits and pieces of information scattered in siloed databases throughout the organization. For instance, separate databases usually track the following data:
- information on individual employees, such as current position, job grade or level, salary, prior employers, prior performance reviews, degrees, and patents
- project information, including team assignments, engineering time charges, milestones or stage gates, metrics on quality from design reviews, and the number of on-time tape outs and re-spins
- product information, such as customer support logs, sustaining engineering logs, bug-tracking logs, documentation, app-note authorship, and app-note downloads
- collaboration information, including communication patterns (such as the number of meetings in an employee’s online calendar and the frequency of emails to other group members); simulations; and analysis-system log-ins
- customer information, such as documentation, troubleshooting requests, and complaints
Companies following the Moneyball-for-engineers approach combine the information from these databases into a central repository, or data lake. The criterion for the inclusion of individual data sets should be their perceived relevance—specifically, whether a variable appears to influence engineering performance. Companies should also consider their confidence in the data (whether information is accurate and reliable), completeness (including whether long-term findings are available), and the level of detail. Within the data lake, they can link data sets by using tags, such as employee or project identification numbers (subject to the caveat below).
Evaluating individual performance: What’s the equivalent of slugging percentage in semiconductor engineering?
It’s easy to examine baseball statistics for on-base percentages or total bases and then identify the individual players who have scored highest on each. With engineers, however, individual performance may be more difficult to assess, since the metrics tend to be less straightforward. Another problem is that the typical engineering group is much larger than a baseball roster. All chief technology officers know who their five best people are but may be unfamiliar with the remaining staff, including most of their top 100 engineers.
Lacking information about individual capabilities, managers may have trouble identifying either problematic or exceptionally strong employees. They might therefore overlook opportunities to address performance issues and support professional development. Equally important, managers who do not have clear insights about their employees might create unbalanced teams with too many top or low performers.
Advanced data analytics can help identify talented people by uncovering patterns that may not be immediately obvious when companies look at individual performance. For instance, it might reveal which engineers consistently serve on projects that meet their release dates. One company that used analytics to assess individual performance segmented design engineers into one of three categories:
- top performers, who were two times more productive than the average engineer as measured by factors such as project costs and person-days
- project coordinators who tended to have significant communication issues (for instance, more than one standard deviation from the average email response time)
- the remaining staff
After this segmentation, the company adjusted the composition of its teams to improve their performance: for example, it ensured that top performers were allocated to separate teams so that more projects could benefit from their leadership and expertise. Dividing them among different teams also eliminated or reduced several problems, including potential clashes between experts about the best path forward. The company then discussed communication issues with project coordinators who appeared to be struggling and appointed additional staff to assist them or to serve as their backups, thus alleviating backlogs.
There’s one caveat to this approach: in some countries, an analysis that focuses on individual performance might violate privacy laws or create problems with unions or workers’ councils. In such cases, companies should use advanced data analytics only to identify and measure the drivers of team performance as described below, while keeping data about individuals anonymous.
Optimizing team performance: How do you assemble the best roster of semiconductor engineers?
We have conducted research to explore whether certain factors, such as geographic spread, influenced team performance by looking at data from past analyses. We found that shifting certain staffing parameters, such as team size, could significantly increase productivity. While managers have long known that such factors could affect team performance, our analysis found that some of them have a far greater impact on outcomes than expected. These five are particularly important:
- Team size. Companies typically achieved the best results when project teams had a maximum of six to eight engineers, since they often had difficulty coordinating larger groups.
- Team-member fragmentation. Conventional wisdom says that engineers should focus on one or two projects to maximize productivity. But our analysis showed that productivity increased when engineers worked on more projects. For example, mechanical engineers in one organization did best when they worked on three projects simultaneously, and their productivity didn’t drop until they were assigned to a fourth or fifth. By contrast, firmware engineers benefited from much higher levels of fragmentation, with teams seeing productivity gains when their members were spread across seven or more projects.
- Collaboration history. Strong group dynamics among team members who have worked well together in the past can raise productivity by 7 to 10 percent. There are some limits to this finding, however. In closed networks, where individuals tend to work with the same people on project after project, some teams may see performance decline, which can hurt overall quality, cost, and timelines. Companies can combat these trends by shaking up the membership of such teams.
- Individual experience. We analyzed how various personal attributes affected team performance in workplaces requiring high skills. Experience was the strongest performance driver, surpassing education level and other factors. Companies should therefore strive to have some experienced members on every team—and should also make greater efforts to retain them.
- Geographic footprint. Managers have long known that a diverse geographic footprint—team members based in multiple locations—can make teams less productive. Many, however, might not be aware of the extent of the problem. In our analysis, we found that adding an additional site to a team’s footprint can decrease productivity by as much as 10 percent (Exhibit 2).
How do you track performance data for engineering productivity?
Typically, semiconductor companies track the performance of teams simply by looking at end results—metrics such as overall costs and person-days, as well as whether timelines were met. That’s a bit like looking at a baseball team’s wins for a season but not determining what contributed to them.
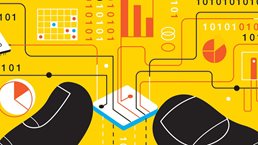
Improving the semiconductor industry through advanced analytics
Our approach can help companies track performance at a more detailed level. For instance, companies can use advanced data analytics to determine how they performed on individual tasks that contribute to product quality, rather than just looking at the overall quality rate. The performance-analysis process is also automated—a big improvement from past practices, which required managers to comb through data manually, enter relevant results in spreadsheets, and create charts for various metrics. All performance information is displayed on dashboards, in real time, using simple visuals. The information is also retained after each project, giving current and future teams an easily accessible record of effective strategies and past mistakes.
With multiple challenges ahead, semiconductor companies are looking for new ways to decrease costs and increase productivity. If they apply advanced analytics to management, as they have to many basic engineering tasks, they can upgrade individual performance and create high-functioning teams. In addition to providing a competitive edge, these changes will help their employees gain greater satisfaction and more enjoyment from their jobs. It’s a win for all.