As we progress into the digital era, semiconductor manufacturing competition is intensifying, with industry players looking to make productivity improvements while undertaking a record level of M&A activity. Front-end fabs and back-end manufacturers have typically focused transformational improvement efforts on direct and indirect labor-cost reduction, overall equipment effectiveness and throughput increases, material consumption and cost reductions, and global-procurement and spending adjustments. Although lean techniques have been the standard method of achieving productivity gains, many companies—particularly back-end manufacturers—have difficulty sustaining lasting impact. Our experience working in Asia shows that a differentiating factor to effectively manage increasing cost pressures and sustain higher profitability is improving end-to-end yield—encompassing both line yield (wafers that are not scrapped) and die yield (dice that pass wafer probe testing).
Stay current on your favorite topics
Yield optimization has long been regarded as one of the most critical, yet difficult to attain goals—thus a competitive advantage in semiconductor operations. According to the Integrated Circuit Engineering Corporation, yield is “the single most important factor in overall wafer processing costs,” as incremental increases in yield significantly reduce manufacturing costs.1 In this regard, yield can be viewed as being closely tied to equipment performance (process capability), operator capability, and technological design and complexity. Over the years, advances in fab technology such as more efficient air-circulation systems and better operator capabilities, as well as efforts to lessen direct human contact with the production process through the use of automation, have led to a decline in particulate problems.2 And yet many semiconductor players struggle to implement sustainable yield improvements due to ingrained mind-sets, an insufficient view of data, and isolated efforts as well as a lack of advanced-analytics capabilities.
As devices continue to get smaller and more sophisticated, the effects of Moore’s law—that is, the estimation that the number of transistors in a given chip doubles every two years—will continue unabated. Thus in the semiconductor industry, the risks to yield due to process variability and contaminations are ever increasing, as is the importance of continuously improving design and machine capabilities. In this paper, we describe a new approach to changing mind-sets, gathering the right data to inform improvement initiatives, and achieving sustainable yield increases through systemic improvements. We also offer an overview of the impact that advanced analytics can have on semiconductor yield and highlight seven capabilities that semiconductor players can pursue to inform their efforts.
Current perspectives on improving yield
Much has been discussed around the advent of Industry 4.0 tools to improve yield across front-end and back-end manufacturers. Yet without even entering that stage of technological maturity, most semiconductor players still seek to understand yield data by focusing on excursions, percentage, or product—or a combination of the three.
A percentage focus involves a bottom-up approach toward viewing yield percentages, either as an integrated view or by specific process areas. This information is typically highly dependent upon the accuracy of the data captured by operators and made readily available for engineers through manufacturing execution systems.
Some manufacturers focus on a specific set of products or product families, either by highest volumes or lowest yield performances. Resources are then assigned to solve for the root causes of specific product problems, as a means of prioritizing the company’s efforts. This approach requires engineering resources from cross-functional teams, such as equipment, process, product, quality, testing, and, of course, yield.
Excursion—that is, when a process or piece of equipment moves out of preset specifications—can be a significant contributor to yield loss, particularly if it goes undiscovered until after fabrication. An excursion focus can thus be defined as tackling the highest and most obvious sources of yield loss or excursion cases identified from past historical occurrences either in the plant or from customer incidents. The key focus is to ensure the root causes of those yield losses and their potential failure modes are addressed to avoid a repeat occurrence.
These approaches can enable manufacturers to capture, monitor, and control various forms of yield losses—but they may leave other opportunities on the table. To target the highest impact on profitability, semiconductor companies must first translate yield loss into actual monetary value (rather than simply volumes or percentages), enabling them to more effectively direct resources toward solutions across all products and processes. This approach goes beyond a yield-loss focus on specific products or excursion cases to encompass a more end-to-end view. As a result, semiconductor companies can more effectively implement systemic process changes and, particularly given the different cost structures for each product, result in significant and as yet unrealized cost savings.
A new approach to semiconductor yield improvements
To translate yield loss into actual monetary value, a semiconductor company must begin by aligning the language and data used by engineering and finance to gain a better understanding of end-to-end yield. Next, it can use a loss matrix to develop a holistic view of the company’s greatest sources of loss; then it can use that data to design more targeted initiatives that will have the biggest impact on increasing yield—and thus on improving the company’s bottom line.
Align the language and data of engineering and finance
In our experience with semiconductor manufacturers, there is a consistent disconnect between the engineering and finance functions. Engineers focus on and celebrate gains in percentage yield, but they often overlook the connection between yield and cost. Indeed, the celebrated percentage increases may or may not lead to any significant impact on the bottom line. Furthermore, many engineering and finance functions use different systems to track yield, which can result in constant disagreements or misalignment between the functions, rendering data less usable by the lack of agreement about which to use as the source of truth.
Would you like to learn more about our Semiconductors Practice?
The first step in ensuring that all functions are aligned in a yield transformation effort is to speak a common language—the cost of poor quality. Using this understanding as a means of alignment immediately proves fruitful for all involved. Not only can engineers and finance personnel understand each other but the ease of translation and communication also extends vertically through the organizational ladder, allowing both ground-level engineers and top-level management to agree on justifications for pursuing initiatives and on progress achieved for successful improvement activities.
To overcome divergent sources of truth, semiconductor companies can construct a cost-of-nonquality (CONQ) baseline that uses cost data from finance as well as engineering (Exhibit 1). For example, finance provides data on standard costs, standard yields, and yearly volumes per product, while engineering provides detailed breakdowns on the nature (reject category) and source (process) of the defects by product. Merging these two views provides a full and readily approachable view of the cost of yield losses.
Develop a holistic, data-driven view of what needs to improve and where
Typically, engineers are dedicated to discrete processes, enabling them to develop deep expertise in a given area and more effectively serve on the line. However, when embarking on a yield transformation, a semiconductor company must develop a holistic view of the manufacturing process. Therefore engineering must take a step back to see exactly what parts of the process, and specifically what reject categories, lead to the greatest amount of loss. While some companies already undertake a product focus to yield losses, an overarching view of the entire manufacturing line is usually not top of mind. Thus, instead of a singular transformation, what usually happens is a lot of the efforts are siloed into individual processes, products, and even pieces of equipment.
A loss matrix enables engineering to map process areas (in a heat map) and reject categories against yield performance of the manufacturing line from start to finish. One manufacturer found that across the eight major steps of its semiconductor production process, the company was losing almost $68 million due to yield losses overall, including almost $19 million during electrical testing alone (Exhibit 2). Engineers can use their technical knowledge of what happens in particular processes to determine why certain reject codes are high within those processes. By also calculating the addressable amount of loss, this heat map view enables the organization to prioritize its focus and allocate resources to the process areas most likely to improve profitability.
In our experience, having this view handy is extremely useful not only to ensure that everyone has a view of what must be addressed and where but also to keep track of what areas have been covered—and which ones are still unexplored. The heat map also enables engineers to take a top-management approach toward the line as a whole, instead of focusing only on their particular process, and reinforces the view that all engineers are responsible for managing quality and yield.
Implement systemic improvements to identify yield loss
Once the biggest loss areas are identified using the loss matrix, it is important to ensure the resulting improvement activities are sustainable; this starts by isolating the products that are the biggest contributors to scrap (Exhibit 3). This per-product analysis ensures that action is taken only on items that have the biggest impact on yield.
As a result, engineers have the detailed insight they need to solve for key themes that drive the particular losses identified by the loss matrix. They can also use a product Pareto analysis to identify the use cases where addressing an issue will solve the most significant, far-reaching problems.
Key improvement themes are generally structured using the traditional “5 Ms” of lean manufacturing—machine, man, material, measurement, and method. While organizing loss categories along these lines, semiconductor companies should also analyze which rejects are true and which are false, as well as discuss what potential cross-functional collaborations may help solve the issue. One manufacturer completed an analysis on four of the Ms (measurement was not applicable in that case) and sorted out true from false rejects while also developing a sound foundation for improvement initiatives (Exhibit 4).
One finding from the yield loss analysis showed that the manufacturer was experiencing contamination and wrinkle issues at a particular process point. The ensuing problem-solving session identified underlying, systemic issues in the manufacturing process, resulting in four improvement initiatives relating to both true and false rejects (Exhibit 5).
Given their cross-functional nature, the machine variability initiatives entailed both internal effort and external involvement. Internally, product, process, and test engineers, quality engineering, and R&D worked together to run the necessary tests and qualifications to ensure the activity had no negative impact on semiconductor quality. Armed with their analysis, engineers could have more meaningful discussions with external vendors about legacy patches to existing equipment and ideas to improve machine performance.
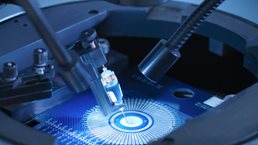
How the semiconductor industry is taking charge of its transformation
The implementation of these four initiatives reduced contamination rejects for identified products by 90 percent, and wrinkle rejects by 40 percent, and in the long term gave valuable insight to engineers in both collaborating with third parties as well as ingraining an ownership mind-set.
Impact to a yield engineer’s typical day, with the holistic view of yield improvements
Yield engineering resources are typically spent supporting or leading improvement activities across both product and process engineering. At one manufacturer, yield engineers’ daily activities ranged across three main areas—root-cause problem solving of excursions and other critical identified yield losses, cross-functional yield improvement activities and collaborations with other teams, and operational tracking and reporting of yield performances across the fab. By applying a holistic approach toward yield improvements based on the steps described above, a typical day in the life of a yield engineer improved in all three realms.
Root-cause problem solving. The majority of yield engineering resources used to be spent on yield loss analyses and low-yield threshold troubleshooting, for both mature products and new product releases, from product development including buy-off approvals. Due to the yield loss analysis, the manufacturer’s yield engineers could shift from a reactive “firefighting” stance on tackling ad hoc requests or manufacturing execution system triggers to solving for root causes of major excursions or other weekly yield losses on the line. Engineers can now identify key losses as per the loss matrix that are unaddressed and start with the one that will have the biggest forecasted impact to the bottom line. Internal problem solving is further strengthened with the help of big data analytics solutions that proactively highlight commonalities or pattern recognition—for example, a particular tool, process group, or even upstream product or process that contributes significantly to yield losses (see sidebar, “The role of advanced analytics in semiconductor yield improvement: Converting data into actions”). Yield solutions can help push efficiency improvements to the team by providing proactive, low-yield threshold warnings and reporting while also improving turnaround time for lot releases.
Cross-functional yield improvements. Previously, resources were spread across multiple projects or initiatives with other engineering teams, with the main task of using analytics to identify the impact of recommended improvements. Armed with end-to-end traceability of yield losses from front end to back end, yield teams benefit from a more granular view of bottom-line impact, reducing the analytical resources needed and allowing for more insights to be shared with the cross-functional team, including R&D, business-unit sales and marketing teams, and front- and back-end managers. Teams can effectively link decisions from customer requirements (either by R&D or business units), down to bottom-line impact on front-end and back-end expected yield losses, to identify systemic root causes cutting across processes, reject categories, or products. This capability helps yield engineers be more precise in identifying which teams (product or process engineers) are needed and to prioritize which initiatives they ought to invest most of their time. From an efficiency improvement and workload-reduction perspective, teams can better rationalize meeting participation.
Yield engineers are further empowered with data to highlight potential opportunities to implement more yield gains by aligning or relaxing internal specifications, without affecting customer demand or satisfaction. Transparency enables teams across the value chain to collaborate around more data and push initiatives to be more fact based and prioritize resources to maximize profitability.
Yield performance tracking and reporting. For both mature and new unreleased products, yield engineers have shifted from daily or weekly yield percentage monitoring to more continuous monitoring thanks to the capabilities of the loss matrix. Performance baselines and improvements can be tracked and reported either in the form of the loss matrix, or with the help of analytical yield solutions. Teams can now visualize the distribution of key forecasted shifts in yield losses as measured by monetary impact, which helps prioritize the next wave of improvement initiatives. Reporting is more mutually exclusive and collectively exhaustive than previously limited reporting by process and integral yield percentages.
For semiconductor companies, the successes of effective yield improvement lead not only to increased profitability but also to better organizational health as a whole. Our experience points to three central key pillars that make yield transformations successful:
Aligning the language and data of engineering and finance. Looking at yield percentages only provides one view of the situation; engineering and finance alike must align on using the cost of poor quality as the method for understanding and guiding the direction of the company’s yield improvement efforts. Collaboration on the creation of a CONQ calculation can ensure that improvement initiatives are based on a viable foundation of data and collaboration.
Develop a holistic, data-driven view of what needs to improve and where. Work on yield can often be siloed due to how manufacturing organizations are structured. Using the loss matrix and analytical solutions—where costs can be easily viewed by processes, reject codes, or products—allows engineers and managers to gain a better view of the health of the entire manufacturing process, from R&D through wafer fabrication and die packaging, to push improvement efforts to the right areas. This view also gives engineers and managers a chance to track what areas they are already tackling, as well as what areas have yet to be explored.
Implement systemic improvements. Yield improvements should address excursion cases—but more important, they should also tackle the baseline yield. By setting up discussions where engineers can explore historic causes of yield loss, new levers can be discovered that will increase overall yield performance for a certain product or process. There can also be situations where certain losses are tolerated simply because they have historically been seen as acceptable. Focusing on standout issues of yield loss, as well as working to continuously improve the baseline yield percentage as a whole, leads to more sustainable yield improvement.