The assumption that predictive maintenance is the only advanced, analytics-based use for Internet of Things (IoT) data in the maintenance world has created a great deal of misconception and loss of value. While predictive maintenance can generate substantial savings in the right circumstances, in too many cases such savings are offset by the cost of unavoidable false positives.
Luckily, while predictive maintenance is probably the best-known approach, there are other powerful ways to enhance a business’s maintenance-service organization and create value from analytics-based technologies. The two most valuable of these, we find, are condition-based maintenance and advanced troubleshooting. A comprehensive look at all uses of IoT data and advanced analytics for maintenance purposes is therefore a crucial step in determining which technology—or combination of technologies—to employ.
Why companies have invested in data
Companies across the globe have made substantial investments in the IoT, advanced analytics (AA), and associated technologies. In 2019, our colleagues estimated that more than 25 percent of businesses were already using IoT to connect with their equipment, and the number of IoT-connected devices was projected to increase to 43 billion by 2023. Working in partnership, IoT sensors gather data such as equipment temperature, vibration, flow, and pressure, and AA analyzes this data to help to determine the equipment’s condition.
Leading executives have used these technologies to transform the way their companies service equipment. And one of the most popular approaches for doing so in recent years has been the use of predictive-maintenance systems, which determine the causes of a machine’s previous breakdowns and use sensor data to intervene before another breakdown occurs, minimizing downtime.
What could be more attractive than better uptime, greater predictability, and, presumably, lower service costs?
Would you like to learn more about our Operations Practice?
When predictive maintenance falls short
After spending heavily to establish predictive-maintenance programs, however, some businesses have struggled to recoup their investments. The technologies that promised to alert them to a pending breakdown are effective, yet predictive maintenance can go beyond what’s required, causing companies to shut down equipment earlier or more often than necessary.
Although predictive maintenance is often held up as a prime example of the value that IoT and advanced analytics can generate, in fact, any predictions in the real world are imperfect. Our research shows that some organizations, even with highly qualified AA teams, are unlikely to realize the desired impact. The AA algorithm employed may fail to predict a breakdown, giving a false negative, and in other cases can predict an event that would not have happened, giving a false positive. Although much effort is often put into minimizing false negatives, it is often the false positives that make predictive maintenance less viable.
Make no mistake, predictive maintenance can be very valuable. In situations with very high cost or safety issues associated with a breakdown, such as the midair failure of a jet turbine, operators need the closest estimate possible of when a breakdown might occur. In addition, in cases in which failures are highly predictable and well-understood—and the chance of a false positive is therefore minimal or very low-cost—predictive maintenance is well worth the expense.
Unfortunately, quite a few business situations fail to meet these parameters. Many events are difficult to predict, and the costs associated with taking preventive action are substantial; in contrast, the savings from predicting these events a few days in advance tend to be modest. And any equipment shutdown is a hassle—whether the machine simply breaks down on its own or is stopped during a deliberate, preventive shutdown.
One company, for example, produced a piece of equipment that required servicing an average of four times a year, costing around $1,500 in parts and labor for each occurrence. With a lot of effort, the company succeeded in using predictive maintenance to predict about one-quarter of its breakdowns with 85 percent accuracy, saving more than 10 percent on each predicted breakdown by avoiding overtime and rush shipments of parts. Given that software and user-caused issues are notoriously hard to predict (and the breakdown of electronics is essentially random), these results represent a major improvement.
Over its entire installed base, the company’s management then estimated that savings would reach more than one million dollars a year. Unfortunately, the algorithm used had a false-positive rate of about 10 percent—excellent by most standards, but in practice resulting in 1,000 extra cases per year, which wiped out the overall savings. In addition, the benefit to customers from having scheduled their downtime was minimal: although the service could now be planned a few days in advance, the number of events increased by 10 percent, resulting in greater overall shutdown time.
This type of situation is unfortunately all too common.
Alternative maintenance options
Two other uses of IoT and related technologies in the maintenance world can provide good results with less complex and costly interventions, depending on the situation. These options are condition-based maintenance (CBM) and advanced troubleshooting (ATS). While they rely on the same technologies as predictive maintenance to retrieve and analyze data, they use the data in different way (Exhibit 1).
Condition-based maintenance
In situations in which predictive maintenance is not mandated by the risk involved or the ease of predictability, but a business still wants keep a close watch on its equipment, CBM can provide useful insight. CBM uses IoT and AA to monitor the ongoing condition of the asset and determine its maintenance needs, just as predictive maintenance does. However, CBM typically monitors key performance indicators (KPIs) to identify pending issues, which companies can check through noninvasive measurements, visual equipment inspections, reviews of performance data, or scheduled tests, as well as through IoT and historical data. The KPIs are gathered at certain intervals, or continuously—as is done when a machine has internal sensors. CBM can be applied to both mission-critical and non-mission-critical assets.
Like predictive maintenance, CBM also operates on the principle that maintenance should only be performed when certain indicators show signs of decreasing performance or an upcoming critical failure. Compared to traditional preventive maintenance, however, which calls for equipment to be shut down at regular intervals to replace parts no matter their condition, CBM only requires equipment to be shut down for maintenance on an as-needed basis, increasing the time between maintenance repairs.
One large technology manufacturer introduced a CBM framework that pulled data from several different sources, including IoT devices and a historical service database. The framework allowed the company to identify the appropriate steps to resolve any customer-service request—reducing its labor, downtime, parts, and other related costs by 30 percent.
Advanced troubleshooting
In situations in which a piece of equipment has broken down or seems to have an issue, ATS uses machine data and data about previous failures to identify the root cause of the issue, enabling a faster resolution and improved uptime.
Even with the best assets, equipment failure is bound to occur at some point. When it does, engineers without the benefit of IoT and related technologies traditionally troubleshoot by using issue trees, heading out to the site, and trying several solutions until they achieve success. This approach can result in multiple trips, many labor hours, and unnecessary parts usage, as the engineers try one solution after another—not to mention frustration for both the engineers and the customers who are plagued with higher downtime.
In contrast, ATS uses IoT and related technologies to allow engineers to identify the root cause of an issue remotely, thereby reducing the number of field visits, labor time, and related costs.
One large medical-device manufacturer was suffering from the high costs of unnecessary parts usage, higher-than-standard truck rolls, and long labor hours. In response, it developed an ATS solution that uses historical failure data along with sensor, log, and customer reports to resolve equipment issues. The solution generated an 18 to 25 percent reduction in maintenance costs and a significantly improved customer experience through reduced downtime, as measured against historical data. The solution also led to a standardized resolution process that starts with remote troubleshooting.
For tickets that cannot be resolved remotely, the manufacturer now schedules field-engineer visits that usually can resolve complex problems in a single appointment. As part of the scheduling process, the engineers get all necessary information on the equipment they are servicing, including historical issues and probable root causes, along with standard operating procedures, equipment guides, and specialized support from other experts. Simultaneously, the system provides the engineer with details of any planned preventive maintenance and other work on the equipment, allowing the engineer to complete that work as well and prevent unnecessary truck rolls (Exhibit 2).
Improved troubleshooting can also improve remote resolution, as at an IT service provider that was using its service-desk operators to provide technical solutions to enterprise clients over the phone. Although operators filled out call-description logs on the diagnosis and resolution steps taken to resolve customers’ issues, the process wasn’t standardized, leading to high variability in average handling time (AHT). Introducing an ATS framework allowed agents to follow a standard resolution process, which reduced AHT for those issues by 40 percent.
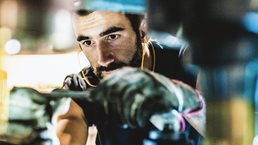
A smarter way to digitize maintenance and reliability
Lessons of simplicity
By itself, deploying the most advanced predictive-maintenance model isn’t likely to deliver the full potential of the IoT and AA for every business. Instead, executives can start by weighing their organization’s unique situation, after which they can choose the most appropriate solutions and make changes to people, processes, and technologies to implement them. While each organization will face distinct challenges, the following steps can help any program improve services.
- Prioritize ATS and CBM. Focusing on ATS or CBM can help a business increase its impact over that of traditional preventive maintenance, while laying the foundation for an analytics-driven maintenance-service strategy. By prioritizing one or both of these approaches, a maintenance program can start to show results at an early stage and become self-funding for more complex models that use data from all IoT sources.
- Combine domain expertise with model intervention. Only by using a combined team of data scientists and service experts can a business develop a true understanding of possible service interventions—and a model that’s effective in generating real value. Data scientists may recommend a given analytics model, but only service experts will be able validate the recommendation and the feasibility of the potential interventions.
- Instill a data-friendly mind-set. Our research shows that organizational mind-sets are the most important factor in successful analytics-enabled transformations. Convincing the service organization’s leadership about these approaches—especially by helping them understand how a change in their roles can potentially make their jobs more rewarding—is therefore essential. If there is no analytics-adoption mind-set and leaders are unable to communicate the benefits to everyone involved, the organization is much more likely to reject the model recommendation as a threat, rather than adopt it as a source of opportunity.
- Employ a data-capture strategy. Analytics models need data, and a strategy for master-data management and governance will be critical for success. Executives therefore need to ensure that data from all sources is being captured, whether through sensors or through logbooks.
The use of advanced analytics is a critical tool for service organizations and cannot be approached successfully as a one-off effort. Instead, it’s best for service organizations to treat AA implementation as an ongoing transformation, one that requires starting small, with less complex models, and includes changed mind-sets—from top leadership to middle managers to field agents.