Asset owners and industry professionals hold a tremendous amount of data on the condition, maintenance, and operation of infrastructure, and this data can be used to greatly improve their capital-planning decisions. Although advanced analytics is increasingly used by infrastructure players in both the private and public sectors, the industry still tends to lag behind others, such as retail, financial services, and automotive, in embracing advanced analytics comprehensively across the project life cycle. In particular, we see a significant opportunity to increase its use in capital-planning processes.
Stay current on your favorite topics
To the extent managers are applying data-driven methods in infrastructure, it is predominantly in the project-delivery phase rather than through integration into capital planning. As a result, many asset owners experience weaker capital productivity than what we see in other sectors, as they are making big infrastructure decisions based primarily on qualitative, rather than quantitative, factors.
Through our work with infrastructure owners and operators, we have been applying advanced-analytics approaches to generate deeper insights and value on infrastructure life cycles, maintenance versus replacement decisions, and asset-longevity trends. Taking advantage of data-driven methods when making decisions in these areas could help asset owners free up capital—we’ve seen 5 to 15 percent portfolio savings—and permit them to reallocate money to more attractive projects. In this article, we outline how advanced analytics can benefit infrastructure owners when applied to capital planning and describe a methodology to pursue this rich opportunity. Those that seize the moment have a chance to become leaders in the field.
Taking advantage of the data-driven environment
Advanced analytics—the ability to generate valuable insights from large amounts of data—has emerged as a powerful tool to understand and learn from past performance as a guide to more accurately predict trends. Analytics creates value when big data and advanced algorithms are applied to business problems to yield a solution that is measurably better than before. By identifying, sizing, and prioritizing the biggest opportunities, businesses can create an analytics strategy that generates value (Exhibit 1).
When applied to infrastructure, acting on these predicted trends can radically improve the ability of owners to make capital-planning decisions based on the expected performance of their existing infrastructure—for example, by enabling much more refined asset life-cycle curves. It can assist them in aligning investments in design, construction, operations, and maintenance of infrastructure assets with expected needs.
Infrastructure owners can learn from technological advances in adjacent sectors, such as oil and gas or manufacturing, where organizations are using big data to spur increased performance and mitigate risk. Advanced analytics uses inputs from diverse data sets, from historical weather data to operations and maintenance records, combined with leading-edge processing systems and machine learning to develop predictive insights. For example, a common use is to forecast the probability of a given event, such as a maintenance need or shutdown.
One of the challenges facing owners of complex assets is to make use of the vast amount of data now being gathered by sensors and sources across their networks. Powerful analytics tools are readily available and can assist by creating a visualization platform and using machine learning to help spot patterns in the data. These tools are becoming commonplace in such diverse industries as healthcare and automotive manufacturing.
The impact can be profound. When an organization can predict the likelihood of a given event, that information can then inform its capital-portfolio-development process and help identify underlying life-cycle drivers, including which preventative mitigation measures it must invest in.
Another benefit of advanced analytics is the level of precision it enables in an owner’s decision making. Traditional industry serviceability benchmarks for longevity are typically time driven or event driven, or both, rather than condition driven. While traditional decisions may have focused on whether to maintain or replace an asset entirely, advanced analytics can help owners prioritize replacement or repair of specific components rather than a complete asset.
Would you like to learn more about our Capital Projects & Infrastructure Practice?
For example, the use of sensors can allow a department of transportation to identify bridges that require complete reconstruction and others that require only one or two new girders to replace those presenting structural issues. In one case, the South Carolina Department of Transportation has a Federal Highway Administration grant to evaluate the value of structural-health-monitoring technology to complement visual inspection and other information regarding specific bridges.1
Another emerging use is in the railroad industry, where sensors monitor track geometry, rail corrugation, and track-surface measurements, facilitating maintenance and long-term investment decisions. For example, one railway company analyzed its condition-monitoring data to develop a list of prioritized annual-maintenance tasks. Previously, this was done using industry-standard benchmarks that were tied to the broad asset category instead of the actual condition of the items. The use of existing-condition data as a predictor of required maintenance helped save more than 30,000 person-hours a year and allowed the company to redirect $20 million of annual engine-overhaul spending to capital-replacement investments.
How can advanced analytics help?
Asset owners, in both the public and private sectors, are continually faced with deciding whether to spend money to maintain an asset, replace it with a new and improved one, or buy a new asset that meets a fresh requirement or need.
Of course, regulatory, operational, and mission considerations factor into such capital-infrastructure decisions. For example, an asset may need to be replaced to meet new strategic requirements, such as berthing considerations at a port to accommodate larger vessels. Investment may also be necessary to meet new regulatory requirements, such as changes to airport runway-overrun-protection systems.
Nonetheless, we observe that many decisions center on the trade-off between continual investment in maintaining an asset compared with replacing it. Unfortunately, most managers are making those choices without access to the kind of analytics that could best inform their decisions. For example, assets are often replaced too early, based on an incomplete understanding of their expected life cycle and an overreliance on generally acceptable benchmark data instead of asset-specific data. Such premature replacement can deny an asset owner years of effective use and needlessly divert scarce capital funds. In addition, as discussed above, owners may assume that an asset needs to be replaced or reconstructed, when it only requires investment in certain pieces of equipment or structures. Conversely, we have also seen assets that become overmaintained and are effectively “budgetary sinkholes”; in other words, a replacement would have offered a better return on investment than continued spending on maintenance of the asset. These are significant capital-planning decisions that advanced analytics can inform with an accurate and data-based understanding of the current expected life cycle of the asset and its expected future maintenance. This not only generates significant cost savings for the owner but also allows the organization to make much more informed decisions about scheduling and prioritization of when repairs or replacements should take place.
Infrastructure owners generate vast amounts of data. But the data are often isolated, unassimilated, and underused. In some cases, the data are employed for narrow analyses and not aggregated to enable a broader understanding of how an asset is performing.
Three pillars support the integration of advanced analytics into the capital-planning process and development of useful insights for asset-portfolio owners:
- refining the current capital-planning process: integrating predictive insights and establishing structured, repeatable advanced-analytics processes using diverse data sets
- using and integrating leading-edge systems: identifying and aligning the data systems to inform the model and applying sophisticated advanced-analytics tools and systems
- committing to build capabilities: ensuring that the appropriate people and skill sets are in place to promote model development and use across the portfolio
Below, we explore how infrastructure-asset owners can move ahead in these three areas.
Incorporating analytics into the capital-allocation process
Capital-allocation processes vary among organizations. However, we believe a best-in-class approach uses evaluations of asset health in the development of the overall strategy. Exhibit 2 describes one process in which asset-health evaluation—informed by advanced analytics—is front and center in the capital-allocation process.
The decision to replace or refurbish an asset may be influenced by broad, historically established industry benchmarks that are often conservative, rightfully advocating early replacement to avoid failure. However, using advanced analytics allows managers to better understand “instantaneous asset health” by predicting expected asset performance using multiple indicators compared against a wide and deep data set. For example, one asset owner employed predictive models and ground-movement sensors to identify anomalies during tunneling under a city. The approach enabled the asset owner to install a more efficient sensor array and monitoring system that enhanced its ability to conduct predictive maintenance and thus reduce overall long-term capital investment.
Defining the advanced-analytics process
When companies embrace the use of advanced analytics in capital-investment decisions, the goal is to capture and use all applicable data sources, both internally and externally available, to develop a robust predictive model.
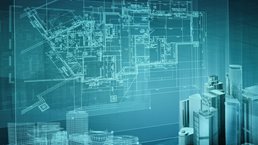
Navigating the digital future: The disruption of capital projects
Success employing these techniques is largely dependent on the ability of organizations to change the way they work and pilot innovation with an open mind. We have found that a three-phase approach is the most effective in developing the tailored predictive systems and integrating them into an owner’s ecosystem: design and data ingestion, proof of concept, and integrate and scale.
This approach is detailed in the following sections and outlined in Exhibit 3.
Design and data ingestion
During the initial phase of incorporating advanced analytics into the organization, a structured database of all likely internal and external data sources is developed. The early evaluation of the data set provides a first assessment of likely drivers affecting overall asset performance. Sources of data would include the following:
- design and construction records
- operational records
- maintenance and recurring capital-expenditure records
- inspection reports
- incident reports
- historical failure data
- expected remaining life
- seismic data
- historical weather data
- interviews
- previous criticality assessments
Owners need to understand that data are assets (in fact, tech companies typically build business models around data). As data ingestion becomes more sophisticated, owners should strive to collect macro and micro data about their projects throughout the life of the project-development and -execution cycle, as well as link it to external market trends.
Exhibit 4 illustrates the vast array of project-related data that owners can access and need to effectively “own.” These fall into three broad categories: project data (tied to the specific project), multiproject data (tied to a portfolio), and external data (tied to influences that will affect the project from the outside).
Proof of concept
The second phase is supported by the outputs from the initial model design and data-ingestion process. A range of tools can be used to model past events and identify a set of quantified performance drivers to predict future performance. Some of the analytical approaches include proportional-hazard modeling, incipient-failure detection, and probability-distribution functions.
The output from this phase enables a dynamic predictive model for critical assets to identify failure mode, performance drivers, and life-cycle timing.
Integrate and scale
In the final phase, the advanced-analytics model is constantly updated to provide real-time predictions of asset performance. Once the initial model is established, other predictive models can then be created to estimate the likely performance of other asset types and subsystems within the capital portfolio. Typically, the systems, processes, and capabilities developed up to this point are used to efficiently scale the use of advanced analytics across the remainder of the infrastructure portfolio.
Deploying and integrating leading analytics systems
Significant thought goes into which systems and tools to deploy when developing a predictive system. The systems and tools should be integrated with existing information-technology systems. System selection is underpinned by the required sophistication of analysis and the complexity of the data set.
Committing to build capabilities
The third pillar supporting the integration of advanced analytics into the capital-planning process is ensuring that the right people and skill sets are in place. Owners should invest in professionals who can develop enterprise-level analytics.
This will likely require investment in all aspects of talent development, including recruiting, hiring, and training. In addition, and perhaps most important, organizations will likely need to retool their systems, processes, and culture to reflect the value of advanced analytics. Leaders must make it clear that analytics is not a marginal capability; on the contrary, they must ensure that its practitioners have the authority and organizational reach necessary for impact.
Infrastructure-asset owners have been slow to introduce advanced analytics into their capital-planning processes. But they can take heart from the experiences of adjacent manufacturing industries, where the introduction of advanced analytics has led to improved returns on invested capital. Incorporating advanced analytics into the capital-portfolio-planning process could lead to more efficient deployment of capital in both the private and public sectors, enabling owners to fund additional projects across their networks.
Three pillars underpin the integration of advanced analytics into the capital-planning process: process, systems, and people. Each one must be addressed for a successful integration of advanced analytics into the organization. In summary, advanced analytics can assist infrastructure owners by predicting and identifying potential asset and component failures not captured under current processes; evaluating underlying asset life-cycle drivers and informing cost-mitigation and longevity-extending plans; and increasing analytical rigor in investment-opportunity identification.