Maintenance has always been a conundrum for asset-intensive industries: while high uptime is critical to ensure return on assets, these industries often involve difficult and unpredictable circumstances. Mining equipment needs to work in challenging environments, for example. Power plants face tough operating regimes. The refining and chemicals sectors must process demanding materials. These conditions put machines under stress, and the high preventive and reactive maintenance expenditures needed to keep them healthy can be a drain on profitability.
For more than two decades, companies have viewed predictive maintenance (PdM) as a panacea, seduced by the idea that they can predict failures long before they occur. This, they hope, will enable them to better plan or even avoid downtime, increasing uptime while reducing unnecessary preventative and corrective maintenance costs. While many companies have launched isolated pilots, however, few have been able to deploy PdM at scale across their operations.
Several things can stand in the way of a successful large-scale PdM program, and most companies face issues in one or more of the following broad categories:
- Data is insufficient, inaccessible, or of low quality
- Technology is inadequate, with too few sensors or poor IT infrastructure
- Prioritization is difficult, as companies lack a clear view of which assets to include in their PdM programs
- Capabilities are missing, especially the skilled data engineers and data scientists required to build advanced analytical models
- Change management is weak, often because of user-unfriendly design
- Economic return is low, due the high cost of developing models to cover diverse assets and numerous potential failure modes
Predicting success
Overcoming these challenges requires a systematic and holistic approach to the design, development, and implementation of PdM. That approach begins with a clear understanding of the organization’s asset base and its reliability goals. Companies also need to recognize that PdM encompasses a wide range of analytical and technological approaches, with differing levels of complexity, costs and benefits (Exhibit 1).
The highest-maturity, asset-wide PdM 4.0 systems are still rare today. They require substantial investment in R&D, along with deep industry knowledge, access to relevant data, and practical operational experience. While bringing in a partner with a proven track record substantially reduces the cost of deployment and adoption, the players that stand to benefit most from such a sophisticated approach tend to share several characteristics:
- Multiple assets or plants sharing a degree of similarity, which enables scale advantages such as replication of models and sharing of data and best practices
- Asset-constrained growth, with no commercial limitation to selling more product—so that additional production converts into additional sales
- A large and diverse range of downtime root causes that need to be addressed to achieve sizeable impact
- High-value failure modes (such as in critical equipment) that occur at low frequency each year, making them harder for traditional methods or typical AI approaches to predict accurately
We believe the time has come for more organizations to move toward higher levels of PdM maturity. Although barriers to success remain, several recent developments have reduced their extent and improved the business case for the approach. Those developments include cheaper and more readily available sensors, higher data availability, increased processing power, a gradually increasing pool of advanced-analytics talent, and a stronger ecosystem of technical partners that have invested in the necessary IP to further industrialize the predictive-maintenance-model development process.
From our experience working with industrial companies across sectors, we have identified five golden rules for the successful implementation of predictive maintenance at scale.
- Be judicious about which assets to include
- Consider the right partners
- Provide sufficient time to improve models
- Put people first
- Build predictive maintenance into the organization’s wider digital ecosystem
Would you like to learn more about our Operations Practice?
Golden rule 1: Choose assets carefully
Although Level 3.0 and 4.0 PdM implementations are now proven to work at scale, they require a certain level of Internet of Things (IoT) capability, a long data history, and downtime of sufficient value to provide an attractive return on investment. This is the case in situations such as upstream oil and gas facilities, large refineries, petrochemical plants, power-generation facilities, paper mills, and mining operations. Predictive maintenance may not be the most economic maintenance strategy at this moment for certain other industries and assets, however. Ultimately the decision on where to implement PdM requires an asset-by-asset validation of the potential benefits and data availability.
We believe companies can prioritize assets that fulfill the following three criteria. First, they are critical to operations, meaning failures can result in immediate loss of production. For instance, the breakdown of rotating equipment in oil refineries often causes the instantaneous shutdown of the unit or even the entire complex. Second, the assets have sufficient sensor coverage and data availability. For every asset under consideration for PdM, organizations should validate the number and types of sensors installed, the availability and retrievability of historical data, and the connectivity of online data, before deciding if the assets are suitable for model development. Third, they are assets that have demonstrated sufficient past failure or anomaly behaviors. To build machine-learning models, data scientists need to learn from historical behaviors. Assets with little failure history make it much harder to develop meaningful machine-learning models.
Successful companies across industries are prioritizing assets for PdM following these criteria. For instance, a renewable-power company prioritized the gear boxes of its wind turbines, an oil and gas company in Asia prioritized critical rotating equipment (such as the main air blower, compressors, gas turbines, and pumps), and several mining companies selected engines for the dump trucks and excavators as the critical equipment for their PdM implementations.
Golden Rule 2: Tech partners matter
The more sophisticated the approach for predictive maintenance, the more it requires a proven track record developed through heavy investments in knowledge, data, and development. PdM 4.0 is arguably more challenging to master than most digital and analytics use cases because of the complexity of initial modelling, model implementation, and ongoing model maintenance.
Beyond the technical implementation, the other half of the challenge is in the last mile: the change management involved in assuring that PdM tools are creating meaningful and tangible value is far from trivial. The right partner can help make PdM adoption seamless, for instance by providing training tailored to the personnel involved, while engaging them throughout the deployment to ensure buy-in, or by integrating PdM actions into existing workflow systems (Exhibit 2).
As the value of reliability for large industrials typically lies in a wide range of equipment and failure modes, a large and complex set of PdM models is required. It rarely makes sense to develop these models in-house, especially from scratch. Instead, there are partners that can provide substantial intellectual property and data, significantly reducing both the time to impact and the investment required. Some of these vendors have emerged from within asset-intensive industries themselves, with technology offerings and IP based on years of experience developing and deploying PdM in large-scale real-world applications.
Golden Rule 3: Allow time for continuous improvement
While building an initial set of predictive models for an organization’s assets is a significant investment, it is only the first step in an ongoing process of continual refinement and improvement. During this post-implementation phase, companies typically seek to improve three aspects of their PdM systems:
- Precision. This is the fraction of the alerts generated by the system that correspond to a real issue in the asset. In early iterations, PdM models will often generate a significant number of false alarms. Over time, this behavior can undermine operating teams’ confidence in the model, creating the risk that they start to ignore warnings generated by the system. To avoid this, the operating team needs to work with the PdM team as part of the deployment effort. Their consistent feedback will enable the modelers to improve precision and ensure that recommendations generated by the model are both accurate and actionable.
- Recall. This is the fraction of real-life issues with the asset that are predicted by the model. Recall is the metric that most directly indicates value capture, but there is usually a trade-off with precision. A model that generates numerous alarms may catch all the failures (high recall), but it is often incorrect and may not be trusted (low precision). With a multidisciplinary team and clear feedback from operations, models may be fine-tuned to achieve the best balance.
- Breadth. A predictive maintenance system is only valuable if it predicts the failure modes that cause value erosion. In large, industrial environments, this typically means a long tail of root causes across machines and processes, which slowly changes as plants get older and processes, machines, software, and people’s behavior all evolve. For a large-scale PdM system, many models are needed to capture substantial value in a plant (often exceeding triple digits). Given this, a continuous deployment of models addressing new root causes can be considered and pursued.
Golden Rule 4: Put people first
A successful predictive-maintenance implementation requires not only accurate model development, but also new processes and mind-sets to embed the changes. In fact, change management that puts the user at the center of the implementation is the single most critical success factor to ensure adoption at scale and achieve sustainable impact.
Five elements together form a change-management program that supports the introduction of PdM (Exhibit 3). The first is an end-to-end process redesign that aims to provide clarity on roles and responsibilities post implementation, based on the collective design input from cross-functional teams. The second is sustained capability building to equip the organization with the immediate technical skills required for the digital solution, and which also addresses the long-term requirement for an internal pool of talent for data engineering, data science, and design thinking.
Third, new KPIs and incentives are usually required, which look beyond technical issues (such as model accuracy) and focus instead on broader leading and lagging indicators, such as unplanned-downtime reduction, adherence to internal maintenance-process timelines, and the adoption rate of the solution. Some companies even use digitized gamification to incentivize user adoption and usage.
Fourth, top management also faces the task of transforming its collective mind-set to role model the new way of working and make their commitment to PdM visible to everyone. Finally, a carefully crafted change story, regular omnichannel communication and campaigns, and bite-size microlearning nuggets can promote engagement across all levels of the organization.
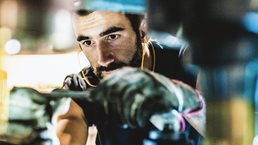
A smarter way to digitize maintenance and reliability
Golden rule 5: Build PdM into the digital ecosystem
Even the most sophisticated PdM system will create value only if it drives a response in the field: a set of actions that are clearly derived from the alarm the system generates. As a PdM system is scaled up, it becomes increasingly difficult to manage such actions consistently. That means creating tight links between PdM systems and other parts of the digital maintenance ecosystem (Exhibit 4). In particular, leading companies are integrating predictive maintenance technologies with new or existing digital work management (DWM) systems.
The links between PdM and DWM work in both directions. Predictive maintenance alarms should trigger work orders in the DWM system, for example, to ensure that action is taken to prevent failures. And the results of those actions should then be fed back to the team managing the PdM system, allowing them to continuously improve its performance. The combination of PdM and DWM allows the performance and impact of the system to be measured effectively, creating accountability and justifying investments.
Tight integration also helps companies improve the capabilities of their PdM systems and the effectiveness of their maintenance processes. Lessons learned from breakdowns or maintenance interventions can be stored in a knowledge base and shared among different teams, assets, and plants. The DWM system also aids the proactive planning of ongoing PdM developments, helping teams identify and prioritize opportunities to extend the assets and failure modes covered by the system. And planners can adapt and optimize their preventative maintenance strategies to take full advantage of their growing predictive capabilities.
While many companies have found it difficult to make predictive maintenance work at scale, the experience of the early adopters shows that success is possible. By judiciously prioritizing assets, establishing the right partnerships with vendors, investing sufficient time to improve models, putting people first, and integrating PdM into their wider digital maintenance ecosystems, best-in-class industrial players have shown the way, capturing value from both increased uptime and reduced maintenance costs.